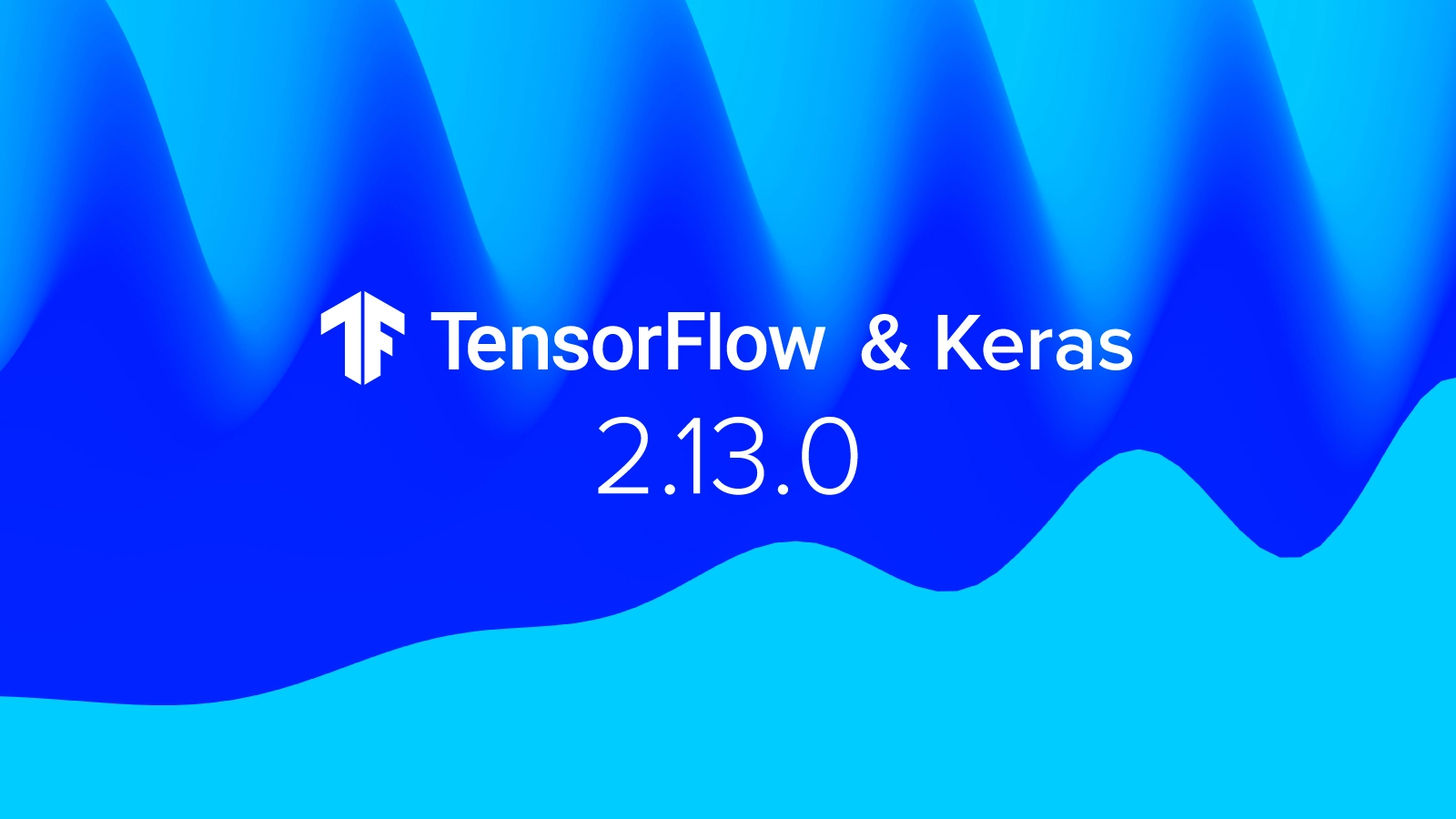
TensorFlow 2.13 and Keras 2.13 have been released! Highlights of this release include publishing Apple Silicon wheels, the new Keras V3 format being the default for .keras extension files, and many more!
TensorFlow Core
TensorFlow 2.13 is the first version to provide Apple Silicon wheels, which means when you install TensorFlow on an Apple Silicon Mac, you will be able to use the latest version of TensorFlow. The nightly builds for Apple Silicon were released in March 2023 and this new support will enable more fine-grained testing.
tf.lite
The Python TensorFlow Lite Interpreter bindings now have an option to use experimental_disable_delegate_clustering flag to turn-off delegate clustering during delegate graph partitioning phase. You can set this flag in TensorFlow Lite interpreter Python API
interpreter = new Interpreter(file_of_a_tensorflowlite_model, experimental_preserve_all_tensors=False
The flag is set to False by default. This is an advanced feature in experimental that is designed for people who insert explicit control dependencies via with tf.control_dependencies() or need to change graph execution order.
Besides, there are several operator improvements in TensorFlow Lite in 2.13
- add operation now supports broadcasting up to 6 dimensions. This will remove explicit broadcast ops from many models. The new implementation is also much faster than the current one which calculates the entire index for both inputs the the input instead of only calculating the part that changes.
- Improve the coverage for quantization by enabling int16x8 ops for exp, mirror_pad, space_to_batch_nd, batch_to_space_nd
- Increase the coverage of integer data types
- enabled int16 for less, greater_than, equal, bitcast, bitwise_xor, right_shift, top_k, mul, and int16 indices for gather and gather_nd
- enabled int8 for floor_div and floor_mod, bitwise_xor, bitwise_xor
- enabled 32-bit int for bitcast, bitwise_xor, right_shift
tf.data
Improved usability and added functionality for tf.data APIs.
tf.data.Dataset.zip now supports Python-style zipping. Previously, users were required to provide an extra set of parentheses when zipping datasets as in Dataset.zip((a, b, c)). With this change, users can specify the datasets to be zipped simply as Dataset.zip(a, b, c) making it more intuitive.
Additionally, tf.data.Dataset.shuffle now supports full shuffling. To specify that, data should be fully shuffled; use dataset = dataset.shuffle(dataset.cardinality()). This will load the full dataset into memory so that it can be shuffled. Make sure to only use this with datasets of filenames or other small datasets.
Also added is a new tf.data.experimental.pad_to_cardinality transformation which pads a dataset with zero elements up to a specified cardinality. This is useful for avoiding partial batches while not dropping any data.
Example usage:
ds = tf.data.Dataset.from_tensor_slices({'a': [1, 2]})
ds = ds.apply(tf.data.experimental.pad_to_cardinality(3))
list(ds.as_numpy_iterator())
[{'a': 1, 'valid': True}, {'a': 2, 'valid': True}, {'a': 0, 'valid': False}]
This can be useful, e.g. during evaluation when partial batches are undesirable, but it is also important not to drop any data.
oneDNN BF16 Math Mode on CPU
oneDNN supports 2.12, is now the default for all files with the .keras extension.
You can start using it now by calling model.save(“your_model.keras”).
It provides richer Python-side model saving and reloading with numerous advantages:
- Faster & lightweight format:
- Human-readable: The new format is name-based, with a more detailed serialization format that makes debugging much easier. What you load is exactly what you saved, from Python’s perspective.
- Safer: Unlike SavedModel, there is no reliance on loading via bytecode or pickling – a big advancement for secure ML, as pickle files can be exploited to cause arbitrary code execution at loading time.
- More general: Support for non-numerical states, such as vocabularies and lookup tables, is included in the new format.
- Extensible: You can add support for saving and loading exotic state elements in custom layers using save_assets(), such as a FIFOQueue – or anything else you want. You have full control of disk I/O for custom assets.
The legacy formats (h5 and Keras SavedModel) will stay supported in perpetuity. However, we recommend that you consider adopting the new Keras v3 format for saving/reloading in Python runtimes and using model.export() for inference in all other runtimes (such as TF Serving).
Full 2.13 Release Notes
TensorFlow
Breaking Changes
- The LMDB kernels have been changed to return an error. This is in preparation for completely removing them from TensorFlow. The LMDB dependency that these kernels are bringing to TensorFlow has been dropped, thus making the build slightly faster and more secure.
Major Features and Improvements
-
tf.lite
- Added 16-bit and 64-bit float type support for built-in op cast.
- The Python TF Lite Interpreter bindings now have an option experimental_disable_delegate_clustering to turn-off delegate clustering.
- Added int16x8 support for the built-in op exp
- Added int16x8 support for the built-in op mirror_pad
- Added int16x8 support for the built-in ops space_to_batch_nd and batch_to_space_nd
- Added 16-bit int type support for built-in op less, greater_than, equal
- Added 8-bit and 16-bit support for floor_div and floor_mod.
- Added 16-bit and 32-bit int support for the built-in op bitcast.
- Added 8-bit/16-bit/32-bit int/uint support for the built-in op bitwise_xor
- Added int16 indices support for built-in op gather and gather_nd.
- Added 8-bit/16-bit/32-bit int/uint support for the built-in op right_shift
- Added reference implementation for 16-bit int unquantized add.
- Added reference implementation for 16-bit int and 32-bit unsigned int unquantized mul.
- add_op supports broadcasting up to 6 dimensions.
- Added 16-bit support for top_k.
-
tf.function
- ConcreteFunction (tf.types.experimental.ConcreteFunction) as generated through get_concrete_function now performs holistic input validation similar to calling tf.function directly. This can cause breakages where existing calls pass Tensors with the wrong shape or omit certain non-Tensor arguments (including default values).
-
tf.nn
- tf.nn.embedding_lookup_sparse and tf.nn.safe_embedding_lookup_sparse now support ids and weights described by tf.RaggedTensors.
- Added a new boolean argument allow_fast_lookup to tf.nn.embedding_lookup_sparse and tf.nn.safe_embedding_lookup_sparse, which enables a simplified and typically faster lookup procedure.
-
tf.data
- tf.data.Dataset.zip now supports Python-style zipping, i.e. Dataset.zip(a, b, c).
- tf.data.Dataset.shuffle now supports tf.data.UNKNOWN_CARDINALITY When doing a "full shuffle" using dataset = dataset.shuffle(dataset.cardinality()). But remember, a "full shuffle" will load the full dataset into memory so that it can be shuffled, so make sure to only use this with small datasets or datasets of small objects (like filenames).
-
tf.math
- tf.nn.top_k now supports specifying the output index type via parameter index_type. Supported types are tf.int16, tf.int32 (default), and tf.int64.
-
tf.SavedModel
- Introduced class method tf.saved_model.experimental.Fingerprint.from_proto(proto), which can be used to construct a Fingerprint object directly from a protobuf.
- Introduced member method tf.saved_model.experimental.Fingerprint.singleprint(), which provides a convenient way to uniquely identify a SavedModel.
Bug Fixes and Other Changes
-
tf.Variable
- Changed resource variables to inherit from tf.compat.v2.Variable instead of tf.compat.v1.Variable. Some checks for isinstance(v, tf compat.v1.Variable) that previously returned True may now return False.
-
tf.distribute
- Opened an experimental API, tf.distribute.experimental.coordinator.get_current_worker_index, for retrieving the worker index from within a worker, when using parameter server training with a custom training loop.
-
tf.experimental.dtensor
- Deprecated dtensor.run_on in favor of dtensor.default_mesh to correctly indicate that the context does not override the mesh that the ops and functions will run on, it only sets a fallback default mesh.
- List of members of dtensor.Layout and dtensor.Mesh have slightly changed as part of efforts to consolidate the C++ and Python source code with pybind11. Most notably, dtensor.Layout.serialized_string is removed.
- Minor API changes to represent Single Device Layout for non-distributed Tensors inside DTensor functions. Runtime support will be added soon.
-
tf.experimental.ExtensionType
- tf.experimental.ExtensionType now supports Python tuple as the type annotation of its fields.
-
tf.nest
- Deprecated API tf.nest.is_sequence has now been deleted. Please use tf.nest.is_nested instead.
Keras
Keras is a framework built on top of the TensorFlow. See more details on the Keras website.
Breaking Changes
- Removed the Keras scikit-learn API wrappers (KerasClassifier and KerasRegressor), which had been deprecated in August 2021. We recommend using SciKeras instead.
- The default Keras model saving format is now the Keras v3 format: calling model.save("xyz.keras") will no longer create a H5 file, it will create a native Keras model file. This will only be breaking for you if you were manually inspecting or modifying H5 files saved by Keras under a .keras extension. If this breaks you, simply add save_format="h5" to your .save() call to revert back to the prior behavior.
- Added keras.utils.TimedThread utility to run a timed thread every x seconds. It can be used to run a threaded function alongside model training or any other snippet of code.
- In the keras PyPI package, accessible symbols are now restricted to symbols that are intended to be public. This may affect your code if you were using import keras and you used keras functions that were not public APIs, but were accessible in earlier versions with direct imports. In those cases, please use the following guideline:
- The API may be available in the public Keras API under a different name, so make sure to look for it on keras.io or TensorFlow docs and switch to the public version.
- It could also be a simple python or TF utility that you could easily copy over to your own codebase. In those case, just make it your own!
- If you believe it should definitely be a public Keras API, please open a feature request in keras GitHub repo.
- As a workaround, you could import the same private symbol keras keras.src, but keep in mind the src namespace is not stable and those APIs may change or be removed in the future.
Major Features and Improvements
- Added F-Score metrics tf.keras.metrics.FBetaScore, tf.keras.metrics.F1Score, and tf.keras.metrics.R2Score.
- Added activation function tf.keras.activations.mish.
- Added experimental keras.metrics.experimental.PyMetric API for metrics that run Python code on the host CPU (compiled outside of the TensorFlow graph). This can be used for integrating metrics from external Python libraries (like sklearn or pycocotools) into Keras as first-class Keras metrics.
- Added tf.keras.optimizers.Lion optimizer.
- Added tf.keras.layers.SpectralNormalization layer wrapper to perform spectral normalization on the weights of a target layer.
- The SidecarEvaluatorModelExport callback has been added to Keras as keras.callbacks.SidecarEvaluatorModelExport. This callback allows for exporting the model the best-scoring model as evaluated by a SidecarEvaluator evaluator. The evaluator regularly evaluates the model and exports it if the user-defined comparison function determines that it is an improvement.
- Added warmup capabilities to tf.keras.optimizers.schedules.CosineDecay learning rate scheduler. You can now specify an initial and target learning rate, and our scheduler will perform a linear interpolation between the two after which it will begin a decay phase.
- Added experimental support for an exactly-once visitation guarantee for evaluating Keras models trained with tf.distribute ParameterServerStrategy, via the exact_evaluation_shards argument in Model.fit and Model.evaluate.
- Added tf.keras.__internal__.KerasTensor,tf.keras.__internal__.SparseKerasTensor, and tf.keras.__internal__.RaggedKerasTensor classes. You can use these classes to do instance type checking and type annotations for layer/model inputs and outputs.
- All the tf.keras.dtensor.experimental.optimizers classes have been merged with tf.keras.optimizers. You can migrate your code to use tf.keras.optimizers directly. The API namespace for tf.keras.dtensor.experimental.optimizers will be removed in future releases.
- Added support for class_weight for 3+ dimensional targets (e.g. image segmentation masks) in Model.fit.
- Added a new loss, keras.losses.CategoricalFocalCrossentropy.
- Remove the tf.keras.dtensor.experimental.layout_map_scope(). You can user the tf.keras.dtensor.experimental.LayoutMap.scope() instead.
Security
- Fixes correct values rank in UpperBound and LowerBound CVE-2023-33976
TensorFlow and Keras 2.13 Release Notes are sourced from the TensorFlow Github and TensorFlow Blog. Visit TensorFlow for more information.
Have any questions?
Contact Exxact for more information on building out your computing infrastructure.
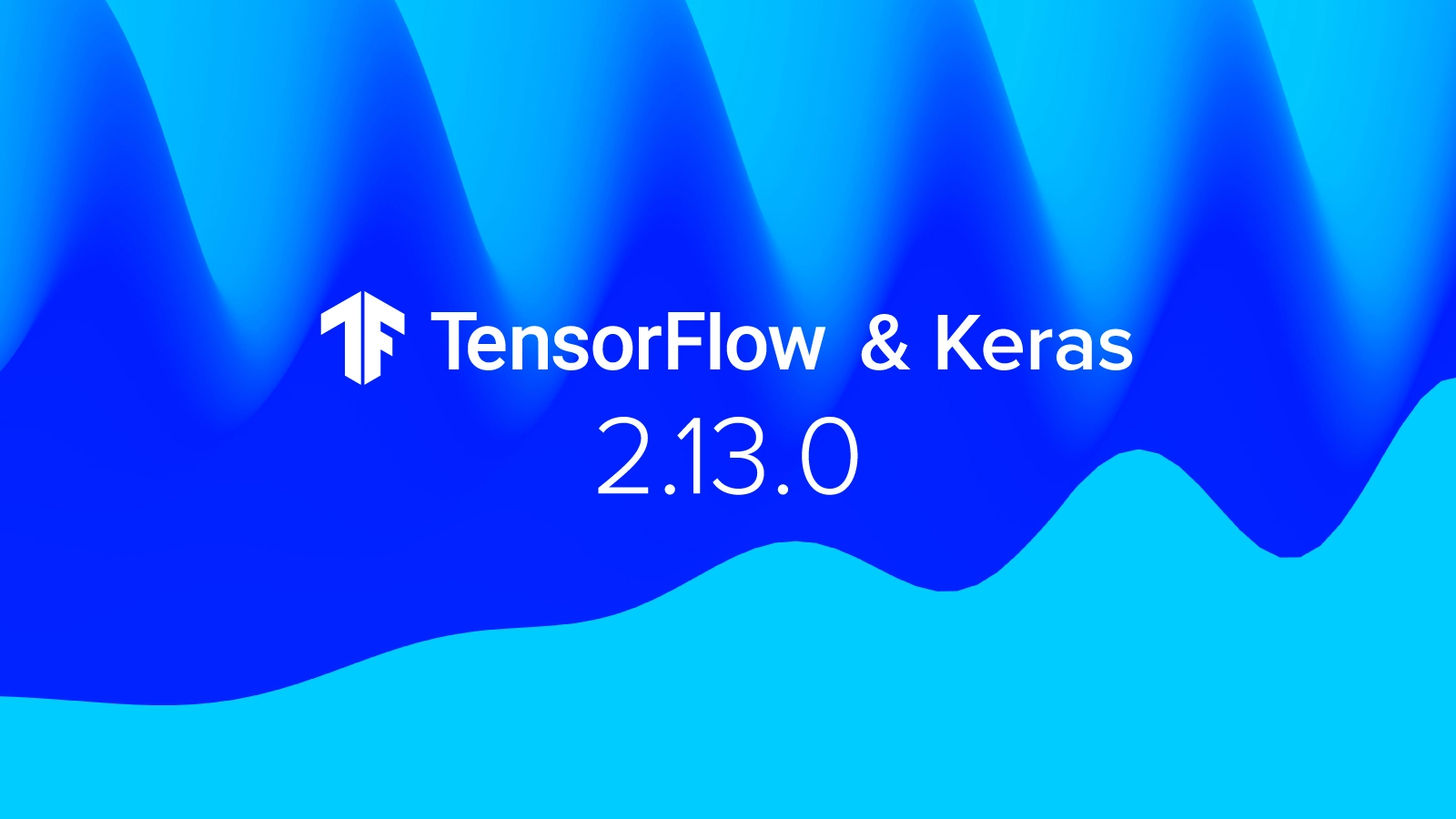
TensorFlow 2.13 and Keras 2.13 Release Notes
TensorFlow 2.13 and Keras 2.13 have been released! Highlights of this release include publishing Apple Silicon wheels, the new Keras V3 format being the default for .keras extension files, and many more!
TensorFlow Core
TensorFlow 2.13 is the first version to provide Apple Silicon wheels, which means when you install TensorFlow on an Apple Silicon Mac, you will be able to use the latest version of TensorFlow. The nightly builds for Apple Silicon were released in March 2023 and this new support will enable more fine-grained testing.
tf.lite
The Python TensorFlow Lite Interpreter bindings now have an option to use experimental_disable_delegate_clustering flag to turn-off delegate clustering during delegate graph partitioning phase. You can set this flag in TensorFlow Lite interpreter Python API
interpreter = new Interpreter(file_of_a_tensorflowlite_model, experimental_preserve_all_tensors=False
The flag is set to False by default. This is an advanced feature in experimental that is designed for people who insert explicit control dependencies via with tf.control_dependencies() or need to change graph execution order.
Besides, there are several operator improvements in TensorFlow Lite in 2.13
- add operation now supports broadcasting up to 6 dimensions. This will remove explicit broadcast ops from many models. The new implementation is also much faster than the current one which calculates the entire index for both inputs the the input instead of only calculating the part that changes.
- Improve the coverage for quantization by enabling int16x8 ops for exp, mirror_pad, space_to_batch_nd, batch_to_space_nd
- Increase the coverage of integer data types
- enabled int16 for less, greater_than, equal, bitcast, bitwise_xor, right_shift, top_k, mul, and int16 indices for gather and gather_nd
- enabled int8 for floor_div and floor_mod, bitwise_xor, bitwise_xor
- enabled 32-bit int for bitcast, bitwise_xor, right_shift
tf.data
Improved usability and added functionality for tf.data APIs.
tf.data.Dataset.zip now supports Python-style zipping. Previously, users were required to provide an extra set of parentheses when zipping datasets as in Dataset.zip((a, b, c)). With this change, users can specify the datasets to be zipped simply as Dataset.zip(a, b, c) making it more intuitive.
Additionally, tf.data.Dataset.shuffle now supports full shuffling. To specify that, data should be fully shuffled; use dataset = dataset.shuffle(dataset.cardinality()). This will load the full dataset into memory so that it can be shuffled. Make sure to only use this with datasets of filenames or other small datasets.
Also added is a new tf.data.experimental.pad_to_cardinality transformation which pads a dataset with zero elements up to a specified cardinality. This is useful for avoiding partial batches while not dropping any data.
Example usage:
ds = tf.data.Dataset.from_tensor_slices({'a': [1, 2]})
ds = ds.apply(tf.data.experimental.pad_to_cardinality(3))
list(ds.as_numpy_iterator())
[{'a': 1, 'valid': True}, {'a': 2, 'valid': True}, {'a': 0, 'valid': False}]
This can be useful, e.g. during evaluation when partial batches are undesirable, but it is also important not to drop any data.
oneDNN BF16 Math Mode on CPU
oneDNN supports 2.12, is now the default for all files with the .keras extension.
You can start using it now by calling model.save(“your_model.keras”).
It provides richer Python-side model saving and reloading with numerous advantages:
- Faster & lightweight format:
- Human-readable: The new format is name-based, with a more detailed serialization format that makes debugging much easier. What you load is exactly what you saved, from Python’s perspective.
- Safer: Unlike SavedModel, there is no reliance on loading via bytecode or pickling – a big advancement for secure ML, as pickle files can be exploited to cause arbitrary code execution at loading time.
- More general: Support for non-numerical states, such as vocabularies and lookup tables, is included in the new format.
- Extensible: You can add support for saving and loading exotic state elements in custom layers using save_assets(), such as a FIFOQueue – or anything else you want. You have full control of disk I/O for custom assets.
The legacy formats (h5 and Keras SavedModel) will stay supported in perpetuity. However, we recommend that you consider adopting the new Keras v3 format for saving/reloading in Python runtimes and using model.export() for inference in all other runtimes (such as TF Serving).
Full 2.13 Release Notes
TensorFlow
Breaking Changes
- The LMDB kernels have been changed to return an error. This is in preparation for completely removing them from TensorFlow. The LMDB dependency that these kernels are bringing to TensorFlow has been dropped, thus making the build slightly faster and more secure.
Major Features and Improvements
-
tf.lite
- Added 16-bit and 64-bit float type support for built-in op cast.
- The Python TF Lite Interpreter bindings now have an option experimental_disable_delegate_clustering to turn-off delegate clustering.
- Added int16x8 support for the built-in op exp
- Added int16x8 support for the built-in op mirror_pad
- Added int16x8 support for the built-in ops space_to_batch_nd and batch_to_space_nd
- Added 16-bit int type support for built-in op less, greater_than, equal
- Added 8-bit and 16-bit support for floor_div and floor_mod.
- Added 16-bit and 32-bit int support for the built-in op bitcast.
- Added 8-bit/16-bit/32-bit int/uint support for the built-in op bitwise_xor
- Added int16 indices support for built-in op gather and gather_nd.
- Added 8-bit/16-bit/32-bit int/uint support for the built-in op right_shift
- Added reference implementation for 16-bit int unquantized add.
- Added reference implementation for 16-bit int and 32-bit unsigned int unquantized mul.
- add_op supports broadcasting up to 6 dimensions.
- Added 16-bit support for top_k.
-
tf.function
- ConcreteFunction (tf.types.experimental.ConcreteFunction) as generated through get_concrete_function now performs holistic input validation similar to calling tf.function directly. This can cause breakages where existing calls pass Tensors with the wrong shape or omit certain non-Tensor arguments (including default values).
-
tf.nn
- tf.nn.embedding_lookup_sparse and tf.nn.safe_embedding_lookup_sparse now support ids and weights described by tf.RaggedTensors.
- Added a new boolean argument allow_fast_lookup to tf.nn.embedding_lookup_sparse and tf.nn.safe_embedding_lookup_sparse, which enables a simplified and typically faster lookup procedure.
-
tf.data
- tf.data.Dataset.zip now supports Python-style zipping, i.e. Dataset.zip(a, b, c).
- tf.data.Dataset.shuffle now supports tf.data.UNKNOWN_CARDINALITY When doing a "full shuffle" using dataset = dataset.shuffle(dataset.cardinality()). But remember, a "full shuffle" will load the full dataset into memory so that it can be shuffled, so make sure to only use this with small datasets or datasets of small objects (like filenames).
-
tf.math
- tf.nn.top_k now supports specifying the output index type via parameter index_type. Supported types are tf.int16, tf.int32 (default), and tf.int64.
-
tf.SavedModel
- Introduced class method tf.saved_model.experimental.Fingerprint.from_proto(proto), which can be used to construct a Fingerprint object directly from a protobuf.
- Introduced member method tf.saved_model.experimental.Fingerprint.singleprint(), which provides a convenient way to uniquely identify a SavedModel.
Bug Fixes and Other Changes
-
tf.Variable
- Changed resource variables to inherit from tf.compat.v2.Variable instead of tf.compat.v1.Variable. Some checks for isinstance(v, tf compat.v1.Variable) that previously returned True may now return False.
-
tf.distribute
- Opened an experimental API, tf.distribute.experimental.coordinator.get_current_worker_index, for retrieving the worker index from within a worker, when using parameter server training with a custom training loop.
-
tf.experimental.dtensor
- Deprecated dtensor.run_on in favor of dtensor.default_mesh to correctly indicate that the context does not override the mesh that the ops and functions will run on, it only sets a fallback default mesh.
- List of members of dtensor.Layout and dtensor.Mesh have slightly changed as part of efforts to consolidate the C++ and Python source code with pybind11. Most notably, dtensor.Layout.serialized_string is removed.
- Minor API changes to represent Single Device Layout for non-distributed Tensors inside DTensor functions. Runtime support will be added soon.
-
tf.experimental.ExtensionType
- tf.experimental.ExtensionType now supports Python tuple as the type annotation of its fields.
-
tf.nest
- Deprecated API tf.nest.is_sequence has now been deleted. Please use tf.nest.is_nested instead.
Keras
Keras is a framework built on top of the TensorFlow. See more details on the Keras website.
Breaking Changes
- Removed the Keras scikit-learn API wrappers (KerasClassifier and KerasRegressor), which had been deprecated in August 2021. We recommend using SciKeras instead.
- The default Keras model saving format is now the Keras v3 format: calling model.save("xyz.keras") will no longer create a H5 file, it will create a native Keras model file. This will only be breaking for you if you were manually inspecting or modifying H5 files saved by Keras under a .keras extension. If this breaks you, simply add save_format="h5" to your .save() call to revert back to the prior behavior.
- Added keras.utils.TimedThread utility to run a timed thread every x seconds. It can be used to run a threaded function alongside model training or any other snippet of code.
- In the keras PyPI package, accessible symbols are now restricted to symbols that are intended to be public. This may affect your code if you were using import keras and you used keras functions that were not public APIs, but were accessible in earlier versions with direct imports. In those cases, please use the following guideline:
- The API may be available in the public Keras API under a different name, so make sure to look for it on keras.io or TensorFlow docs and switch to the public version.
- It could also be a simple python or TF utility that you could easily copy over to your own codebase. In those case, just make it your own!
- If you believe it should definitely be a public Keras API, please open a feature request in keras GitHub repo.
- As a workaround, you could import the same private symbol keras keras.src, but keep in mind the src namespace is not stable and those APIs may change or be removed in the future.
Major Features and Improvements
- Added F-Score metrics tf.keras.metrics.FBetaScore, tf.keras.metrics.F1Score, and tf.keras.metrics.R2Score.
- Added activation function tf.keras.activations.mish.
- Added experimental keras.metrics.experimental.PyMetric API for metrics that run Python code on the host CPU (compiled outside of the TensorFlow graph). This can be used for integrating metrics from external Python libraries (like sklearn or pycocotools) into Keras as first-class Keras metrics.
- Added tf.keras.optimizers.Lion optimizer.
- Added tf.keras.layers.SpectralNormalization layer wrapper to perform spectral normalization on the weights of a target layer.
- The SidecarEvaluatorModelExport callback has been added to Keras as keras.callbacks.SidecarEvaluatorModelExport. This callback allows for exporting the model the best-scoring model as evaluated by a SidecarEvaluator evaluator. The evaluator regularly evaluates the model and exports it if the user-defined comparison function determines that it is an improvement.
- Added warmup capabilities to tf.keras.optimizers.schedules.CosineDecay learning rate scheduler. You can now specify an initial and target learning rate, and our scheduler will perform a linear interpolation between the two after which it will begin a decay phase.
- Added experimental support for an exactly-once visitation guarantee for evaluating Keras models trained with tf.distribute ParameterServerStrategy, via the exact_evaluation_shards argument in Model.fit and Model.evaluate.
- Added tf.keras.__internal__.KerasTensor,tf.keras.__internal__.SparseKerasTensor, and tf.keras.__internal__.RaggedKerasTensor classes. You can use these classes to do instance type checking and type annotations for layer/model inputs and outputs.
- All the tf.keras.dtensor.experimental.optimizers classes have been merged with tf.keras.optimizers. You can migrate your code to use tf.keras.optimizers directly. The API namespace for tf.keras.dtensor.experimental.optimizers will be removed in future releases.
- Added support for class_weight for 3+ dimensional targets (e.g. image segmentation masks) in Model.fit.
- Added a new loss, keras.losses.CategoricalFocalCrossentropy.
- Remove the tf.keras.dtensor.experimental.layout_map_scope(). You can user the tf.keras.dtensor.experimental.LayoutMap.scope() instead.
Security
- Fixes correct values rank in UpperBound and LowerBound CVE-2023-33976
TensorFlow and Keras 2.13 Release Notes are sourced from the TensorFlow Github and TensorFlow Blog. Visit TensorFlow for more information.
Have any questions?
Contact Exxact for more information on building out your computing infrastructure.