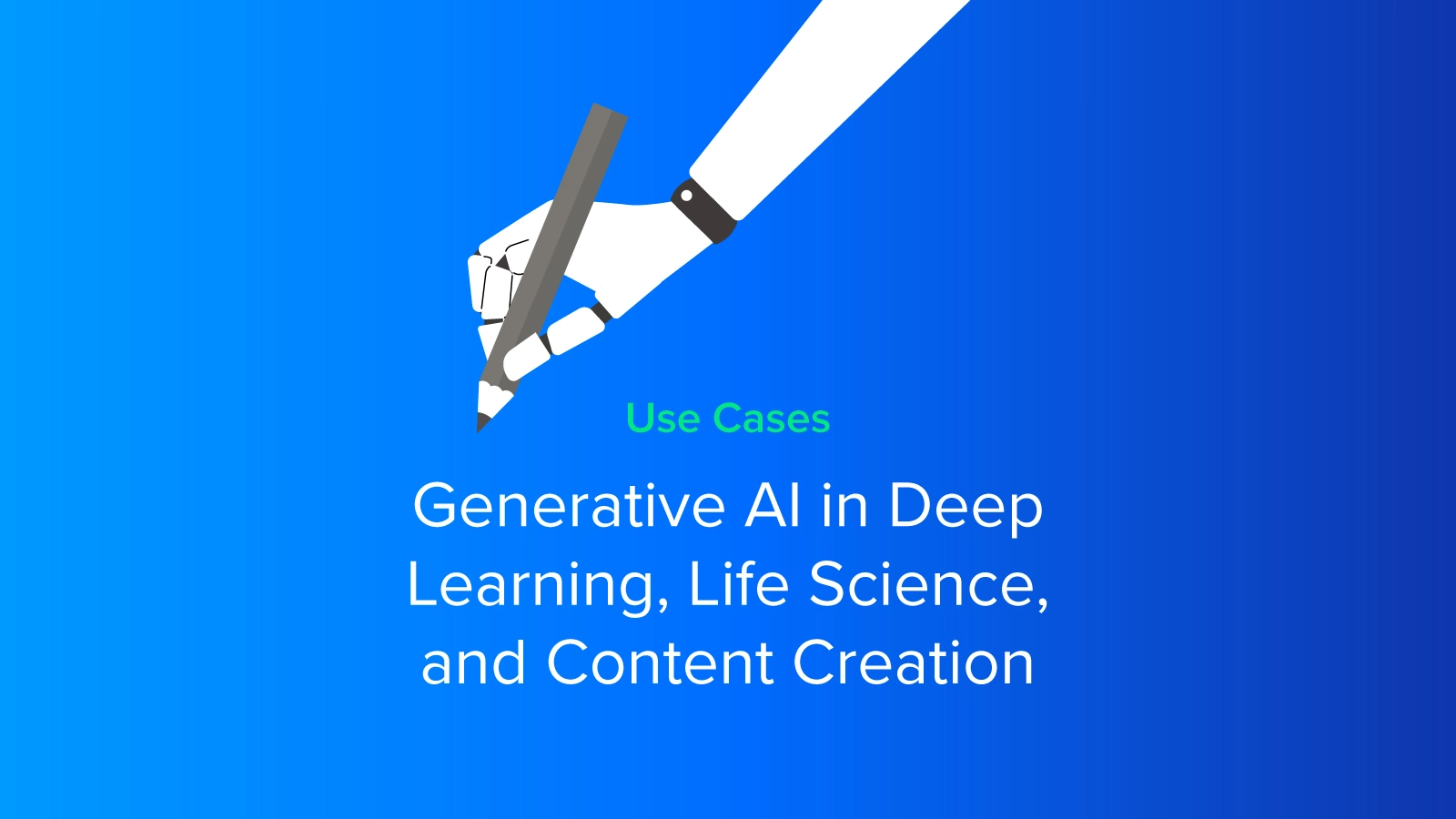
The Emergence of Generative AI
Advancements and innovation in the field of artificial intelligence has been highlighted by the incomprehensible performance of Generative AI, a revolutionary model capable of displaying impressive and unquantifiable creativity. Trained on billions upon billions of data points and parameters, the most prominent generative AI models that brought the field into the limelight is none other than OpenAI’s ChatGPT and DALLE-2.
DALLE-2 is a text to image generator that takes user input prompts and outputs 6 1024x1024 original images with convincing attention to detail. While the model was far from perfect, it displayed the never-before-seen comprehension from a computer, able to develop images detecting sentiment, art styles, and somewhat accurate subjects. Other text to image generative AI models emerged like Midjourney and Stable Diffusion, in which generated images where submitted and awarded in art competitions, displaying unnerving creativity, originality, and beauty. Below is the artwork by Jason Allen’s Midjourney-generated artwork titled “Théâtre D’opéra Spatial” that took first place at the Colorado State Fair for digital artwork, sparking backlash and discourse in the art community.
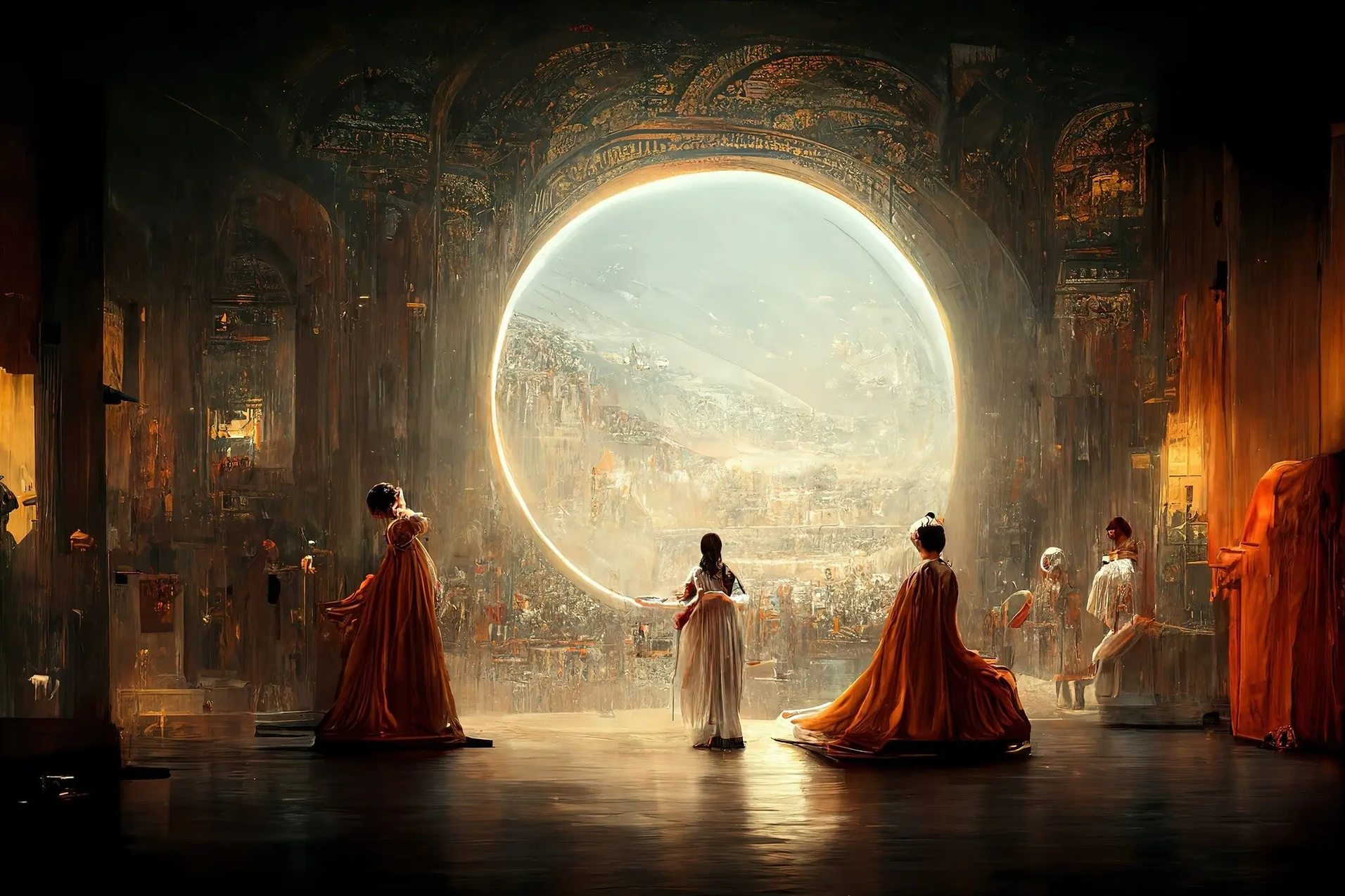
The most polarizing, well known, and widespread generative AI application was and is still ChatGPT, which holds the fastest growing application with over 100 million users in just 2 months of release. ChatGPT is powered by OpenAI’s GPT3 model, now updated to use GPT-3.5 (which includes better parameters and finetuning) and GPT-4 (which increased the training dataset, allows web search, and multi-modal input).
Quick intro to ChatGPT and its backend GPT-X foundation; it is a generative AI large language model formatted as a chatbot that creates original and comprehensive text according to prompt. You can tell it to write a song, answer a question, summarize text, or even assist in code development. The tool is truly revolutionary with many companies utilizing it as an API for specialized use cases such as creating outlines, assisting with grammar, writing fully fledged blogs, and identifying code bugs and errors.
Generative AI models' creativity is a double-edged sword. Asking these models for highly creative outputs can cause hallucination a phenomenon where the output is erroneous disguised as true. This includes text-to-image AIs to fail at depicting hands and text, or AI LLMs stating false facts. Sometimes the AI models may even gaslight users that the generated output is accurate! There are serious efforts to attempt to mitigate the occurrence of hallucinations, but because of the complex inner workings of generative AI, this can possibly never be 100% solved.
How Generative AI Models are Developed?
At its core, generative AI involves the creation of models that can produce new, original content by learning patterns and structures from vast datasets. These models begin with a robust foundation in machine learning and a keen understanding of the underlying principles of neural networks.
It first starts with a very substantial dataset, essential for the neural network to comprehend intricacies and variations within a chosen domain whether text, images, or other modes. To train and run millions and billions of datapoints through the complex neural network requires ample computing, often servers configured with multiple GPUs are the building blocks for large scale computing infrastructures capable of training the most complex generative AI models. Exxact Corp. specializes in delivering high performance computing platforms to enterprise and researchers looking to train deep learning AI and generative AI models, ranging from a single server to multiple racks of servers.
The training process itself presents challenges. Training large-scale generative models is resource-intensive, requiring substantial time and computational power. Developers grapple with fine-tuning parameters and optimizing algorithms to prevent issues like overfitting or mode collapse. This intricate process demands expertise and patience in balancing hyperparameter tuning and model architecture refinement.
Generative AI in Deep Learning
To effectively train deep learning models and achieve accurate predictions, a substantial amount of data is essential. Data holds immense value, particularly in fields where highly specific real-world data is limited. Leveraging generative AI to generate synthetic data enhances the capacity to train a resilient model. Synthetic data can be useful for training AI models when data is nonexistent, private, niche, and or imbalanced.
- Cost Effectiveness: Collecting large amounts of data is both expensive and time consuming. If your business is not already capturing massive amounts of data, it can be difficult to acquire a substantial amount to train a convincing model. Synthetic data can used to generate large training datasets without manual labeling based on a subset of existing data.
- Private & Sensitive: Genuine data may contain sensitive information, like medical histories, making it challenging to share or use for training purposes. Generative AI models can analyze this data and produce statistically accurate synthetic data similar to real data but does not contain any sensitive information, to mitigate the risk of leaking private information and ensuring the security of sensitive data.
- Imbalanced and Biased Data: Synthetic data aids in identifying and correcting potential biases found in real-world data is. For example, if there's a bias in the data labeling most doctors as male where in your model can detract from its purpose, synthetic data can rectify this imbalance by generating more examples of underrepresented classes, preventing biases in your models that would’ve sent to wrong message unintentionally.
- Risky Data: In situations when data is rare and risky to replicate, such as in medical procedures, industrial operations, or autonomous driving simulations, generative AI can generate synthetic data replicating these scenarios without the need for actual data collection in potentially hazardous or complex settings.
Generative AI in Life Science and Healthcare
Researching and tackling the world's most complex issues heavily involves the exploration and examination of drugs and molecules. The life sciences sector stands to undergo a significant transformation through the utilization of generative AI, as it can lower expenses, enhance efficiency and precision, and aid in the creation of reports.
- Drug Discovery: Generative AI models can be train on domain knowledge using datasets containing molecules with distinct characteristics. Researchers can then instruct these models to generate novel molecules that satisfy specific criteria, serving as a valuable initial phase in drug development. This approach not only saves time but also mitigates the costs linked to identifying potential drug candidates. Models like AlphaFold do this already, developing 3D representations of models based on an amino acid sequence.
- Examining and Generating Text: Researchers harness the capabilities of Large Language Model (LLM) generative AI to streamline the summarization of extensive research papers. This method offers scientists a rapid snapshot of research methods and results, enabling them to grasp the essence of a paper swiftly. Additionally, these generative AIs prove instrumental in crafting scientific papers from the ground up. By guiding the AI to outline or produce concise summaries of discoveries, scientists can obtain preliminary drafts of their research reports, cutting down on human effort and accelerating the overall process.
- Patient Monitoring: Generative AI can be used to analyze patient data and create personalized treatment plans. It can also be used to predict patient outcomes and identify potential illnesses and those at risk of developing complications. Healthcare providers can develop interactive intelligent chatbots that can talk to patients about their symptoms via simple language. This can assist by offering treatment suggestions and reduce wait times on trivial calls or alert the institution when urgent action is needed.
Generative AI in Content Creation
The emergence of generative AI delivers a game changing tool for creative professionals. In the domain of content creation, it transforms the conventional approach to designing and animating virtual environments, characters, and objects. These innovations not only broaden creative possibilities but also enhance efficiency in a range of creative and technical workflows.
- Inspiration: Stable Diffusion, Midjourney, and DALLE-2, though not without imperfections, can serve as inspiration. Artists can employ text-to-image models as effective tools to overcome creators block, ensuring a continuous flow of ideas. Moreover, these models can be directed to emulate a particular art style, enabling the generation of images that closely align with the specified aesthetic.
- Assets Creation: Generative AI models revolutionize the industry but accelerating the process of creating models and assets. It can be trained to generate rough 3D models of objects, characters, and environments. It can also be used to quickly generate textures and materials that are automatically tiled which can save time and effort for artists and designers. By employing generative AI to spark creative ideas, design iterations can be significantly accelerated, valuable in fields like automotive and product design.
The Impact of Generative AI
The benefits stemming from training deep learning models to generate original content are significant. Generative AI empowers individuals and creative professionals by providing a means to bypass the challenge of starting from scratch, thereby boosting overall productivity. This capability to transform language or input into creative output redefines the possibilities achievable with artificial intelligence.
However, the implementation of these solutions is not a straightforward process and requires substantial computational resources. AI models like ChatGPT, for instance, rely on the support of thousands of servers to ensure their functionality is accessible to users seeking to leverage these tools. While these models are public, to train and deploy your own model requires on domain knowledge requires ample computing of your own.
Contact Us today if you’re interested in training your own model on domain knowledge for tailored outcomes, Exxact offers expertise in providing the appropriate workstations, servers, or clusters to meet your Deep Learning and AI requirements.
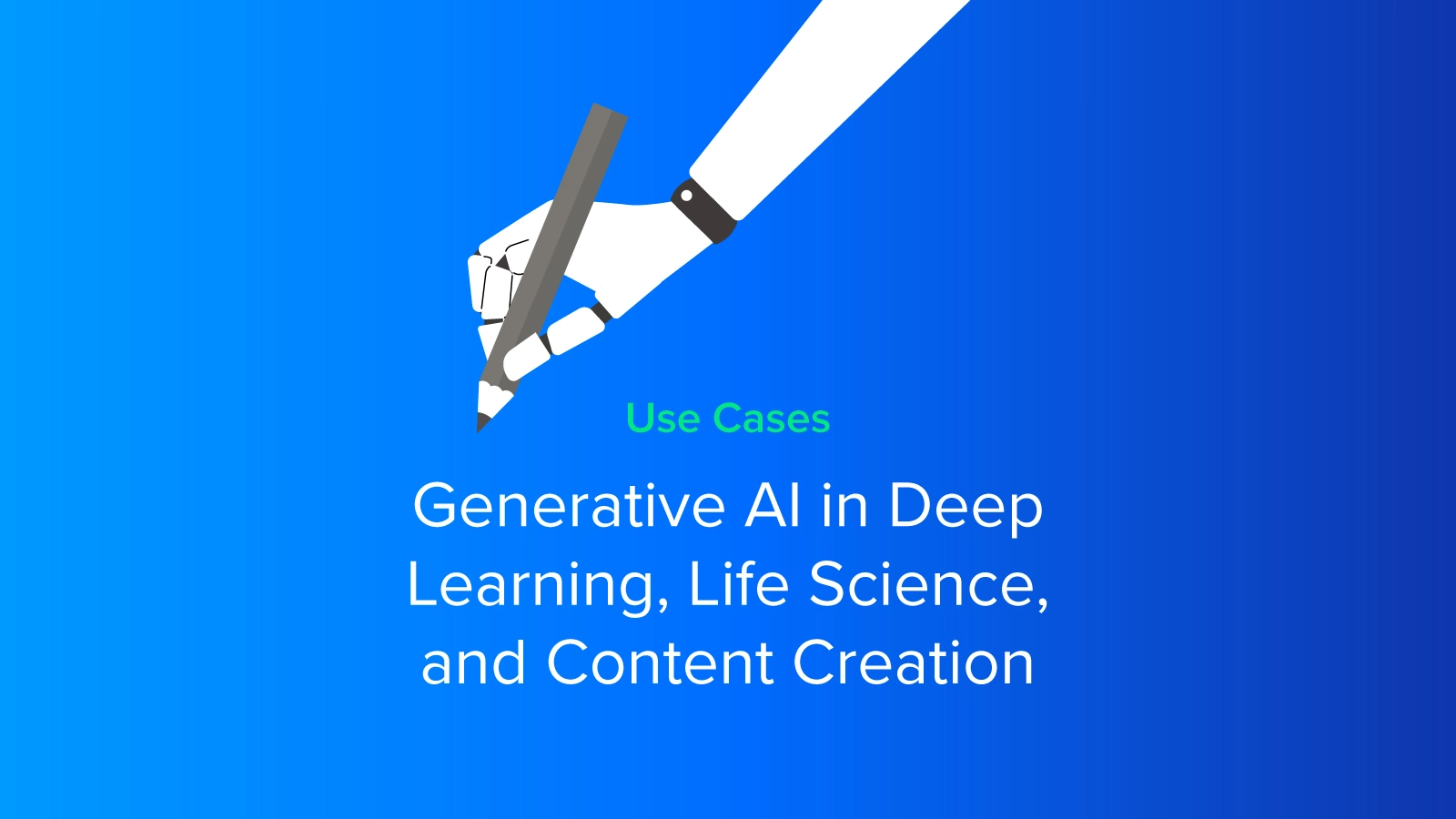
Generative AI Use Cases in Deep Learning, Life Science, and Content Creation
The Emergence of Generative AI
Advancements and innovation in the field of artificial intelligence has been highlighted by the incomprehensible performance of Generative AI, a revolutionary model capable of displaying impressive and unquantifiable creativity. Trained on billions upon billions of data points and parameters, the most prominent generative AI models that brought the field into the limelight is none other than OpenAI’s ChatGPT and DALLE-2.
DALLE-2 is a text to image generator that takes user input prompts and outputs 6 1024x1024 original images with convincing attention to detail. While the model was far from perfect, it displayed the never-before-seen comprehension from a computer, able to develop images detecting sentiment, art styles, and somewhat accurate subjects. Other text to image generative AI models emerged like Midjourney and Stable Diffusion, in which generated images where submitted and awarded in art competitions, displaying unnerving creativity, originality, and beauty. Below is the artwork by Jason Allen’s Midjourney-generated artwork titled “Théâtre D’opéra Spatial” that took first place at the Colorado State Fair for digital artwork, sparking backlash and discourse in the art community.
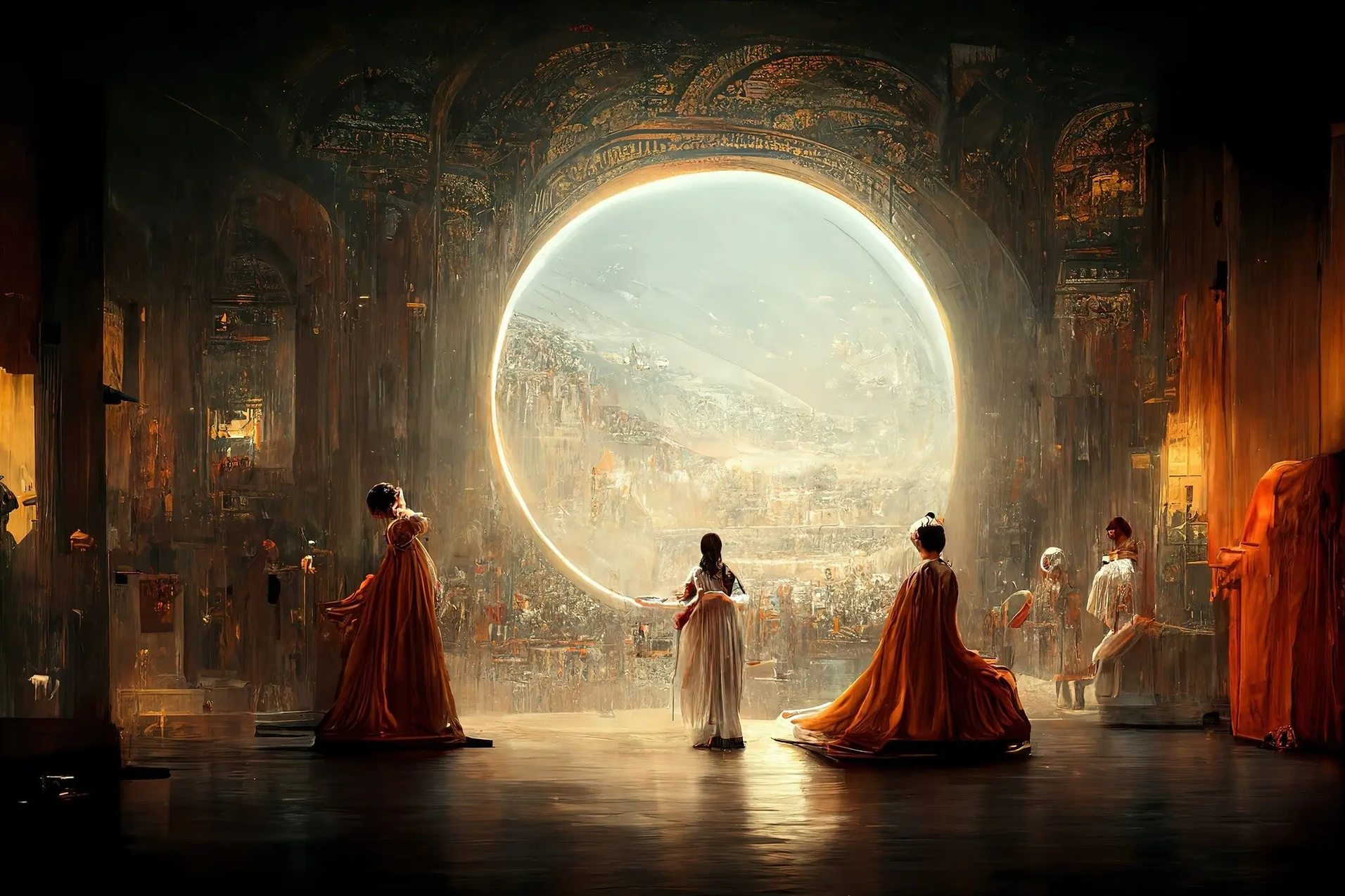
The most polarizing, well known, and widespread generative AI application was and is still ChatGPT, which holds the fastest growing application with over 100 million users in just 2 months of release. ChatGPT is powered by OpenAI’s GPT3 model, now updated to use GPT-3.5 (which includes better parameters and finetuning) and GPT-4 (which increased the training dataset, allows web search, and multi-modal input).
Quick intro to ChatGPT and its backend GPT-X foundation; it is a generative AI large language model formatted as a chatbot that creates original and comprehensive text according to prompt. You can tell it to write a song, answer a question, summarize text, or even assist in code development. The tool is truly revolutionary with many companies utilizing it as an API for specialized use cases such as creating outlines, assisting with grammar, writing fully fledged blogs, and identifying code bugs and errors.
Generative AI models' creativity is a double-edged sword. Asking these models for highly creative outputs can cause hallucination a phenomenon where the output is erroneous disguised as true. This includes text-to-image AIs to fail at depicting hands and text, or AI LLMs stating false facts. Sometimes the AI models may even gaslight users that the generated output is accurate! There are serious efforts to attempt to mitigate the occurrence of hallucinations, but because of the complex inner workings of generative AI, this can possibly never be 100% solved.
How Generative AI Models are Developed?
At its core, generative AI involves the creation of models that can produce new, original content by learning patterns and structures from vast datasets. These models begin with a robust foundation in machine learning and a keen understanding of the underlying principles of neural networks.
It first starts with a very substantial dataset, essential for the neural network to comprehend intricacies and variations within a chosen domain whether text, images, or other modes. To train and run millions and billions of datapoints through the complex neural network requires ample computing, often servers configured with multiple GPUs are the building blocks for large scale computing infrastructures capable of training the most complex generative AI models. Exxact Corp. specializes in delivering high performance computing platforms to enterprise and researchers looking to train deep learning AI and generative AI models, ranging from a single server to multiple racks of servers.
The training process itself presents challenges. Training large-scale generative models is resource-intensive, requiring substantial time and computational power. Developers grapple with fine-tuning parameters and optimizing algorithms to prevent issues like overfitting or mode collapse. This intricate process demands expertise and patience in balancing hyperparameter tuning and model architecture refinement.
Generative AI in Deep Learning
To effectively train deep learning models and achieve accurate predictions, a substantial amount of data is essential. Data holds immense value, particularly in fields where highly specific real-world data is limited. Leveraging generative AI to generate synthetic data enhances the capacity to train a resilient model. Synthetic data can be useful for training AI models when data is nonexistent, private, niche, and or imbalanced.
- Cost Effectiveness: Collecting large amounts of data is both expensive and time consuming. If your business is not already capturing massive amounts of data, it can be difficult to acquire a substantial amount to train a convincing model. Synthetic data can used to generate large training datasets without manual labeling based on a subset of existing data.
- Private & Sensitive: Genuine data may contain sensitive information, like medical histories, making it challenging to share or use for training purposes. Generative AI models can analyze this data and produce statistically accurate synthetic data similar to real data but does not contain any sensitive information, to mitigate the risk of leaking private information and ensuring the security of sensitive data.
- Imbalanced and Biased Data: Synthetic data aids in identifying and correcting potential biases found in real-world data is. For example, if there's a bias in the data labeling most doctors as male where in your model can detract from its purpose, synthetic data can rectify this imbalance by generating more examples of underrepresented classes, preventing biases in your models that would’ve sent to wrong message unintentionally.
- Risky Data: In situations when data is rare and risky to replicate, such as in medical procedures, industrial operations, or autonomous driving simulations, generative AI can generate synthetic data replicating these scenarios without the need for actual data collection in potentially hazardous or complex settings.
Generative AI in Life Science and Healthcare
Researching and tackling the world's most complex issues heavily involves the exploration and examination of drugs and molecules. The life sciences sector stands to undergo a significant transformation through the utilization of generative AI, as it can lower expenses, enhance efficiency and precision, and aid in the creation of reports.
- Drug Discovery: Generative AI models can be train on domain knowledge using datasets containing molecules with distinct characteristics. Researchers can then instruct these models to generate novel molecules that satisfy specific criteria, serving as a valuable initial phase in drug development. This approach not only saves time but also mitigates the costs linked to identifying potential drug candidates. Models like AlphaFold do this already, developing 3D representations of models based on an amino acid sequence.
- Examining and Generating Text: Researchers harness the capabilities of Large Language Model (LLM) generative AI to streamline the summarization of extensive research papers. This method offers scientists a rapid snapshot of research methods and results, enabling them to grasp the essence of a paper swiftly. Additionally, these generative AIs prove instrumental in crafting scientific papers from the ground up. By guiding the AI to outline or produce concise summaries of discoveries, scientists can obtain preliminary drafts of their research reports, cutting down on human effort and accelerating the overall process.
- Patient Monitoring: Generative AI can be used to analyze patient data and create personalized treatment plans. It can also be used to predict patient outcomes and identify potential illnesses and those at risk of developing complications. Healthcare providers can develop interactive intelligent chatbots that can talk to patients about their symptoms via simple language. This can assist by offering treatment suggestions and reduce wait times on trivial calls or alert the institution when urgent action is needed.
Generative AI in Content Creation
The emergence of generative AI delivers a game changing tool for creative professionals. In the domain of content creation, it transforms the conventional approach to designing and animating virtual environments, characters, and objects. These innovations not only broaden creative possibilities but also enhance efficiency in a range of creative and technical workflows.
- Inspiration: Stable Diffusion, Midjourney, and DALLE-2, though not without imperfections, can serve as inspiration. Artists can employ text-to-image models as effective tools to overcome creators block, ensuring a continuous flow of ideas. Moreover, these models can be directed to emulate a particular art style, enabling the generation of images that closely align with the specified aesthetic.
- Assets Creation: Generative AI models revolutionize the industry but accelerating the process of creating models and assets. It can be trained to generate rough 3D models of objects, characters, and environments. It can also be used to quickly generate textures and materials that are automatically tiled which can save time and effort for artists and designers. By employing generative AI to spark creative ideas, design iterations can be significantly accelerated, valuable in fields like automotive and product design.
The Impact of Generative AI
The benefits stemming from training deep learning models to generate original content are significant. Generative AI empowers individuals and creative professionals by providing a means to bypass the challenge of starting from scratch, thereby boosting overall productivity. This capability to transform language or input into creative output redefines the possibilities achievable with artificial intelligence.
However, the implementation of these solutions is not a straightforward process and requires substantial computational resources. AI models like ChatGPT, for instance, rely on the support of thousands of servers to ensure their functionality is accessible to users seeking to leverage these tools. While these models are public, to train and deploy your own model requires on domain knowledge requires ample computing of your own.
Contact Us today if you’re interested in training your own model on domain knowledge for tailored outcomes, Exxact offers expertise in providing the appropriate workstations, servers, or clusters to meet your Deep Learning and AI requirements.