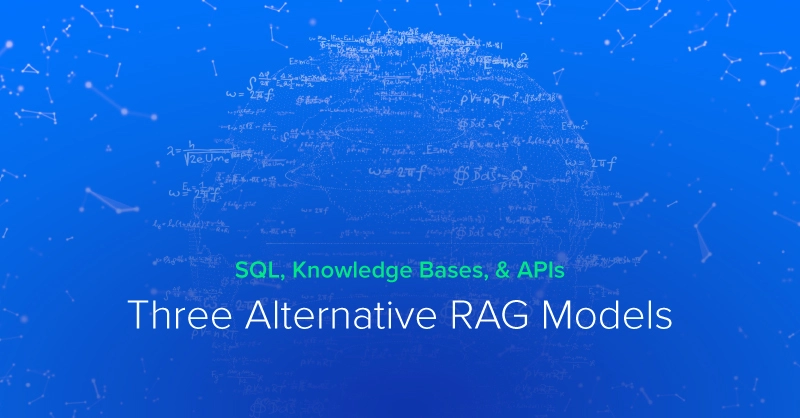
Introduction
Retrieval-Augmented Generation (RAG) has become a foundational technique for improving the accuracy and relevance of large language model (LLM) responses. By providing context via external data sources, RAG alleviates and reduces hallucinations, adds domain-specific context, and makes LLMs far more useful for enterprise and production applications that require less variability.
The most common RAG systems use vector databases, converting text into high-dimensional embeddings and using similarity search to find relevant context for a given query. While effective in most scenarios, it comes with trade-offs.
In this blog, we’ll explore three high-performance RAG systems that offer better accuracy, efficiency, or relevance than vector-based RAG—each suited for a different use case, like tapping into structured data, integrating real-time APIs, or using symbolic reasoning over knowledge graphs.
Why Vector-Based RAG Isn’t Always Optimal
Vector-based retrieval is the default backbone for most RAG implementations, offering a flexible way to match semantically relevant documents using embeddings and similarity search. However, despite its broad applicability, this approach can fall short in scenarios that demand precision or structured reasoning.
Some key limitations of vector-based RAG include:
- Semantic drift: Similarity search may return topic-related but not contextually precise documents.
- Latency at scale: As the corpus grows, retrieval latency can increase, especially without aggressive indexing or sharding.
- Lack of structure-awareness: Vectors obscure the structure of data, making it harder to answer questions that rely on schema, hierarchy, or logic.
And while these challenges are prevalent in other methodologies, they also open the door for alternative RAG methods that are purpose-built for specific data types and retrieval needs, offering improved performance and reliability. In RAG, all is fundamentally the same, where you augment your prompt by using a data retrieval system to provide context. All that’s changing in these other RAG systems is the type of data that can be fed to your model.
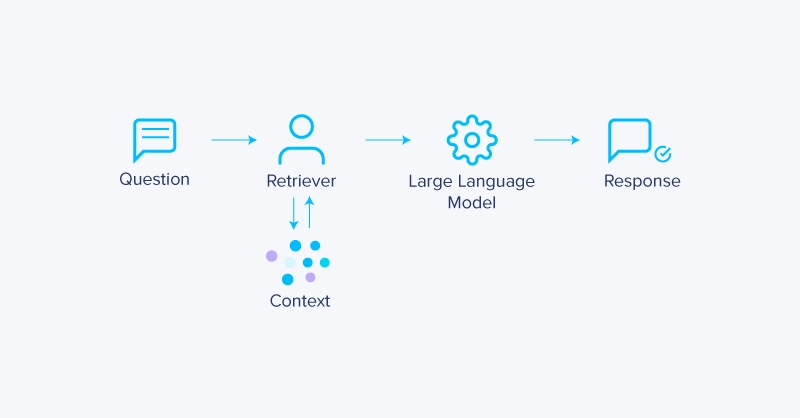
Here’s a summary of the trade-offs and strengths across different RAG architectures, including traditional vector-based RAG with three alternative approaches: Structured Retrieval, API-Augmented, and Knowledge Base RAG.
RAG Type | Best For | Strengths | Weaknesses | Possible Use Case |
Vector-Based RAG |
|
|
|
|
Structured RAG |
|
|
|
|
API-Augmented RAG |
|
|
|
|
Knowledge-Based RAG |
|
|
|
|
Structured Retrieval RAG (SQL/Tabular RAG)
Structured Retrieval RAG integrates LLMs with relational databases or structured tabular sources like SQL tables and CSV files. Instead of relying on vector similarity, the system formulates precise queries to fetch exact data values, ensuring high factual accuracy and traceability, making it ideal for enterprise and regulated environments. Advantages include:
- Deterministic results: Queries return exact matches based on defined schema and constraints.
- Schema awareness: The model understands table structure, field names, and relationships between entities.
- Reduced hallucination risk: Outputs are grounded in verifiable, structured data.
This method is especially effective in finance, healthcare, and manufacturing, where factual precision and data lineage are critical.
API-Augmented RAG
API-Augmented RAG retrieves external information in real time by calling APIs as part of the model’s reasoning process. Instead of relying on pre-ingested document stores, the model accesses dynamic data, like current stock prices, weather conditions, or IoT devices, through live API endpoints. You can even set up a Google Search API. Advantages include:
- Access to real-time data: Ideal for time-sensitive or frequently changing information.
- No static index required: Reduces maintenance overhead and avoids stale context.
- Flexible integrations: Easily connects to proprietary or public services across domains.
This approach is commonly used in LLM-based agents and assistants that operate in dynamic environments or require up-to-the-minute accuracy.
Knowledge-Based RAG (Symbolic RAG)
Knowledge Base RAG systems combine LLMs with structured knowledge representations like knowledge graphs, ontologies, or logic-based rule engines. Rather than relying on fuzzy similarity, these systems retrieve facts or entities based on explicit relationships and logical reasoning, enabling high interpretability and precision. Advantages include:
- Explainability: Retrieval is based on defined entities, relations, and rules, offering full traceability.
- Exactness: Ideal for use cases where legal, technical, or scientific accuracy is mandatory.
- Domain modeling: Capable of capturing domain-specific logic in a structured form.
This method is particularly valuable in sectors like law, compliance, or research, where interpretability and correctness matter more than breadth of knowledge.
Choosing the Right RAG Architecture
Selecting the optimal RAG architecture depends on the nature of your data and the reliable outputs. Consider your specific requirements across these key dimensions:
Data Structure | Data Timeliness | |
Vector RAG | Unstructured | Static |
Structured DB RAG | Structured | Static |
API RAG | Both | Real-Time |
Knowledge Base RAG | Structured | Static & Real Time |
- Data type: Use vector RAG for unstructured text; use structured or symbolic RAG when working with tabular, graph-based, or logic-driven data.
- Real-time requirements: API-Augmented RAG is best when information changes frequently or requires up-to-the-minute accuracy.
- Precision and traceability: Structured and symbolic RAGs shine when deterministic, verifiable answers are necessary.
- Implementation complexity: Vector-based RAG is easier to set up initially, while alternatives may require domain-specific integrations and logic handling.
Ultimately, a hybrid approach—combining multiple retrieval methods—can often deliver the best of all worlds, allowing systems to adapt intelligently based on the type of query or required output quality.
Conclusions
The challenge for any task is picking the right tools to do the job. Retrieval Augmented Generation has impacted how LLMs can be tailored with knowledge. Providing AI with context in the prompt can supercharge its abilities. Vector-based RAG is a versatile choice for broad use cases, but alternative methods like structured, API-augmented, and knowledge base RAG can deliver superior results when precision, context control, or real-time access are critical.
To further level up your AI implementation comes down to the hardware, networking, and storage speed. Talk to our engineers at Exxact to configure your ideal computing infrastructure to power your workload. Our solutions range from ready-to-go GPU workstations, configurable servers, to full rack-integrated clusters.

We're Here to Deliver the Tools to Power Your Research
With access to the highest performing hardware, at Exxact, we can offer the platform optimized for your deployment, budget, and desired performance so you can make an impact with your research!
Talk to an Engineer Today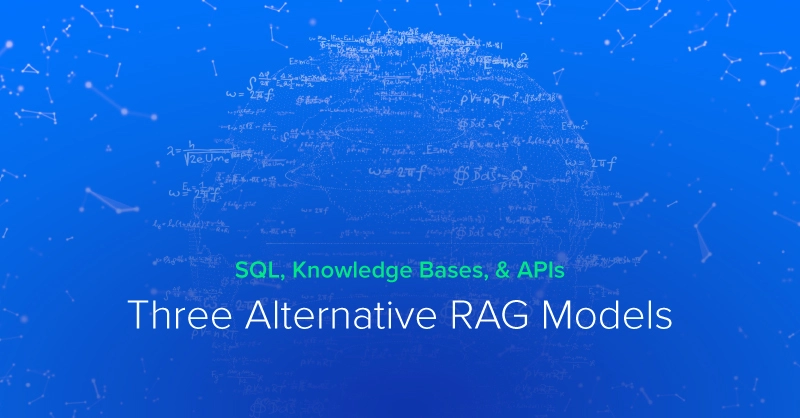
Three Alternative RAG Models - SQL, Knowledge Bases, & APIs
Introduction
Retrieval-Augmented Generation (RAG) has become a foundational technique for improving the accuracy and relevance of large language model (LLM) responses. By providing context via external data sources, RAG alleviates and reduces hallucinations, adds domain-specific context, and makes LLMs far more useful for enterprise and production applications that require less variability.
The most common RAG systems use vector databases, converting text into high-dimensional embeddings and using similarity search to find relevant context for a given query. While effective in most scenarios, it comes with trade-offs.
In this blog, we’ll explore three high-performance RAG systems that offer better accuracy, efficiency, or relevance than vector-based RAG—each suited for a different use case, like tapping into structured data, integrating real-time APIs, or using symbolic reasoning over knowledge graphs.
Why Vector-Based RAG Isn’t Always Optimal
Vector-based retrieval is the default backbone for most RAG implementations, offering a flexible way to match semantically relevant documents using embeddings and similarity search. However, despite its broad applicability, this approach can fall short in scenarios that demand precision or structured reasoning.
Some key limitations of vector-based RAG include:
- Semantic drift: Similarity search may return topic-related but not contextually precise documents.
- Latency at scale: As the corpus grows, retrieval latency can increase, especially without aggressive indexing or sharding.
- Lack of structure-awareness: Vectors obscure the structure of data, making it harder to answer questions that rely on schema, hierarchy, or logic.
And while these challenges are prevalent in other methodologies, they also open the door for alternative RAG methods that are purpose-built for specific data types and retrieval needs, offering improved performance and reliability. In RAG, all is fundamentally the same, where you augment your prompt by using a data retrieval system to provide context. All that’s changing in these other RAG systems is the type of data that can be fed to your model.
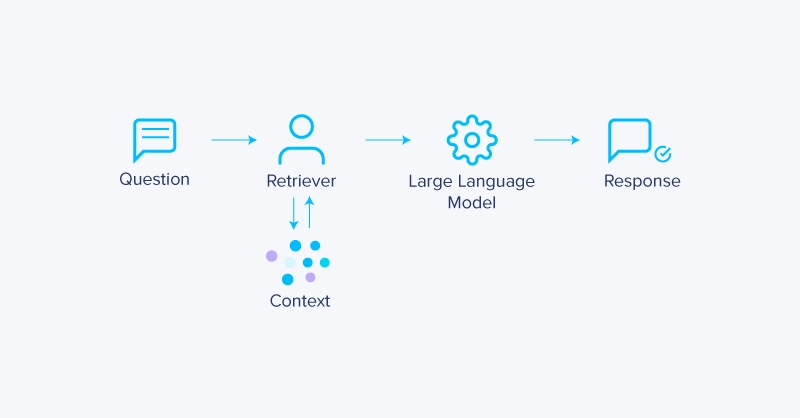
Here’s a summary of the trade-offs and strengths across different RAG architectures, including traditional vector-based RAG with three alternative approaches: Structured Retrieval, API-Augmented, and Knowledge Base RAG.
RAG Type | Best For | Strengths | Weaknesses | Possible Use Case |
Vector-Based RAG |
|
|
|
|
Structured RAG |
|
|
|
|
API-Augmented RAG |
|
|
|
|
Knowledge-Based RAG |
|
|
|
|
Structured Retrieval RAG (SQL/Tabular RAG)
Structured Retrieval RAG integrates LLMs with relational databases or structured tabular sources like SQL tables and CSV files. Instead of relying on vector similarity, the system formulates precise queries to fetch exact data values, ensuring high factual accuracy and traceability, making it ideal for enterprise and regulated environments. Advantages include:
- Deterministic results: Queries return exact matches based on defined schema and constraints.
- Schema awareness: The model understands table structure, field names, and relationships between entities.
- Reduced hallucination risk: Outputs are grounded in verifiable, structured data.
This method is especially effective in finance, healthcare, and manufacturing, where factual precision and data lineage are critical.
API-Augmented RAG
API-Augmented RAG retrieves external information in real time by calling APIs as part of the model’s reasoning process. Instead of relying on pre-ingested document stores, the model accesses dynamic data, like current stock prices, weather conditions, or IoT devices, through live API endpoints. You can even set up a Google Search API. Advantages include:
- Access to real-time data: Ideal for time-sensitive or frequently changing information.
- No static index required: Reduces maintenance overhead and avoids stale context.
- Flexible integrations: Easily connects to proprietary or public services across domains.
This approach is commonly used in LLM-based agents and assistants that operate in dynamic environments or require up-to-the-minute accuracy.
Knowledge-Based RAG (Symbolic RAG)
Knowledge Base RAG systems combine LLMs with structured knowledge representations like knowledge graphs, ontologies, or logic-based rule engines. Rather than relying on fuzzy similarity, these systems retrieve facts or entities based on explicit relationships and logical reasoning, enabling high interpretability and precision. Advantages include:
- Explainability: Retrieval is based on defined entities, relations, and rules, offering full traceability.
- Exactness: Ideal for use cases where legal, technical, or scientific accuracy is mandatory.
- Domain modeling: Capable of capturing domain-specific logic in a structured form.
This method is particularly valuable in sectors like law, compliance, or research, where interpretability and correctness matter more than breadth of knowledge.
Choosing the Right RAG Architecture
Selecting the optimal RAG architecture depends on the nature of your data and the reliable outputs. Consider your specific requirements across these key dimensions:
Data Structure | Data Timeliness | |
Vector RAG | Unstructured | Static |
Structured DB RAG | Structured | Static |
API RAG | Both | Real-Time |
Knowledge Base RAG | Structured | Static & Real Time |
- Data type: Use vector RAG for unstructured text; use structured or symbolic RAG when working with tabular, graph-based, or logic-driven data.
- Real-time requirements: API-Augmented RAG is best when information changes frequently or requires up-to-the-minute accuracy.
- Precision and traceability: Structured and symbolic RAGs shine when deterministic, verifiable answers are necessary.
- Implementation complexity: Vector-based RAG is easier to set up initially, while alternatives may require domain-specific integrations and logic handling.
Ultimately, a hybrid approach—combining multiple retrieval methods—can often deliver the best of all worlds, allowing systems to adapt intelligently based on the type of query or required output quality.
Conclusions
The challenge for any task is picking the right tools to do the job. Retrieval Augmented Generation has impacted how LLMs can be tailored with knowledge. Providing AI with context in the prompt can supercharge its abilities. Vector-based RAG is a versatile choice for broad use cases, but alternative methods like structured, API-augmented, and knowledge base RAG can deliver superior results when precision, context control, or real-time access are critical.
To further level up your AI implementation comes down to the hardware, networking, and storage speed. Talk to our engineers at Exxact to configure your ideal computing infrastructure to power your workload. Our solutions range from ready-to-go GPU workstations, configurable servers, to full rack-integrated clusters.

We're Here to Deliver the Tools to Power Your Research
With access to the highest performing hardware, at Exxact, we can offer the platform optimized for your deployment, budget, and desired performance so you can make an impact with your research!
Talk to an Engineer Today