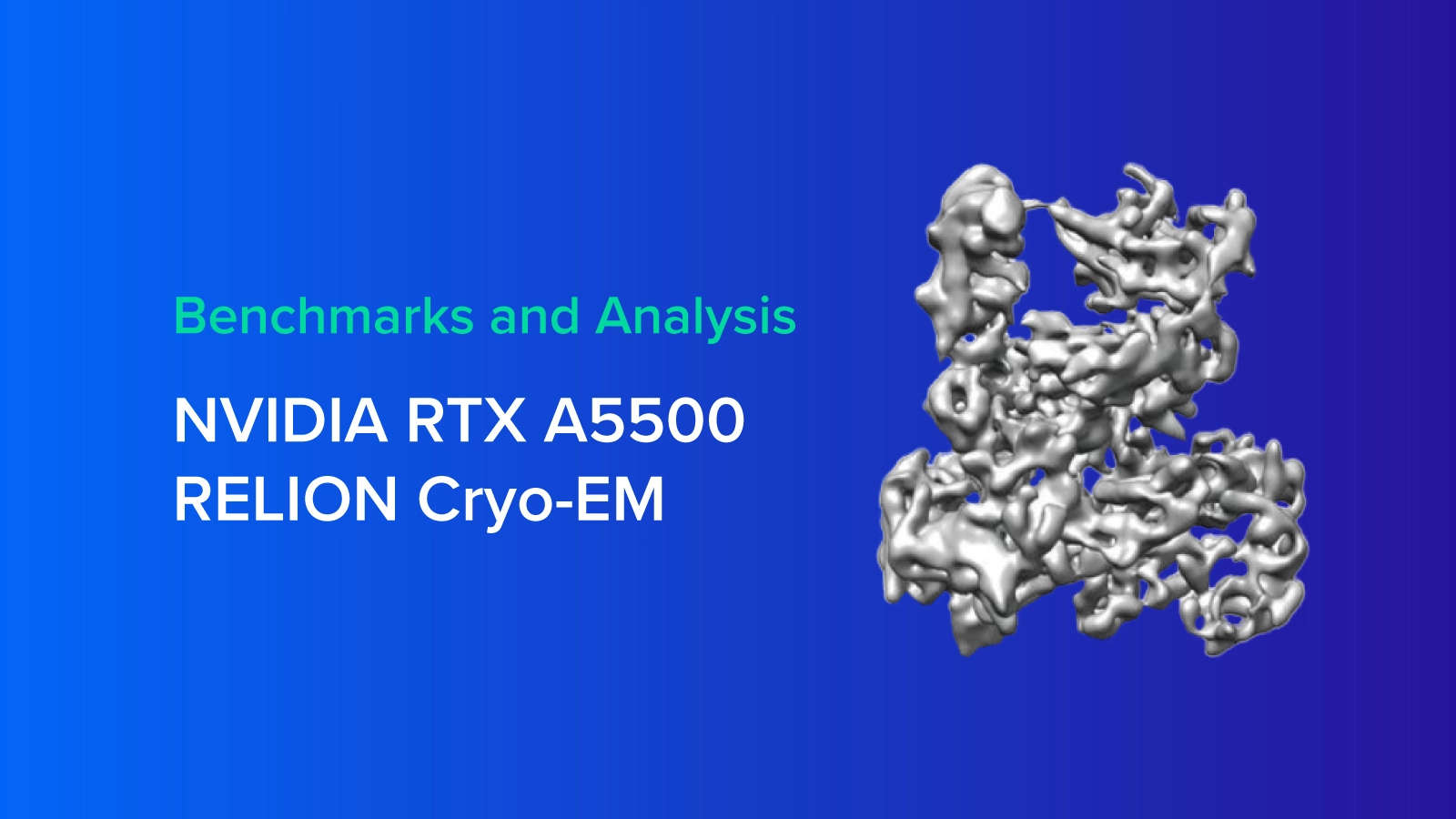
NVIDIA RTX A5500 Benchmarks Overview
As a value-added supplier of scientific workstations and servers, Exxact regularly provides reference benchmarks in various GPU configurations to guide Cryogenic Electron Microscopy (cryo-EM) scientists looking to procure systems optimized for their research. In this blog, we benchmark the NVIDIA RTX A5500 performance using Relion Cryo-EM, comparing GPU runtime to Total Runtime performance.
Software Summary
RELION (REgularised LIkelihood OptimisatioN), or Relion, has revolutionized the cryo-EM field since 2012. Developed by Scheres Lab at the MRC Laboratory of Molecular Biology, this stand-alone computer program uses a Bayesian approach to refine macromolecular structures by single-particle analysis of electron cryo-microscopy data.
The development of RELION is supported through long-term funding by the UK Medical Research Council and is distributed under a GPLv2 license. This means that anyone (including commercial users) can download, use and modify RELION free of cost. The MRC Laboratory just request that if RELION is useful in your work, you will cite their papers.
RELION GPU Support Summary
With advancements in automation, computing power, and visual technology, the scope and complexity of datasets used in cryo-EM have grown substantially. GPU support and acceleration are essential for the flexibility of resource management, prevention of memory limitations, and addressing the most computationally intensive processes of cryo-EM such as image classification, and high-resolution refinement.
Exxact RTX A5500 Workstation System Specs:
Nodes | 1 |
Processor / Count | 2x AMD EPYC 7552 |
Physical Cores | 96 |
Memory | DDR4 512 GB |
Storage | NVMe 3.84 TB |
OS | Rocky 8.5 |
CUDA Version | 11.4 |
RELION Version | 4.0 |
GPU Benchmarks
Benchmarks below are 3D classifications performed using the Plasmodium ribosome dataset
- If runs are performed using the --scratch_dir option in RELION, the time to copy to scratch is subtracted from the total runtime of the command. This allows for the comparison of runs to be independent of storage pool variability.
- "GPU Time" is reported as the time spent on the Expectation step in each of the 25 iterations of classification, which provides a solid snapshot of GPU speed as this is the primary GPU compute step in RELION.
- All benchmarks in this chart are performed using N+1 MPI-ranks where N = Number of GPUS and six threads per process (--j 6). The Systems used in these results have eight GPU cards installed.
Performance Overview
- The NVIDIA RTX A5500 performs as expected showing improvements from 1 GPU through 4.
- The performance improvement drops off after $x GPUs. This is most likely because of the overhead (thread count) while using Multiple GPUs
RELION Benchmarks – GPU Run Time Performance. Note: GPUs 1, 2, and 4 had a standard thread count of 4.
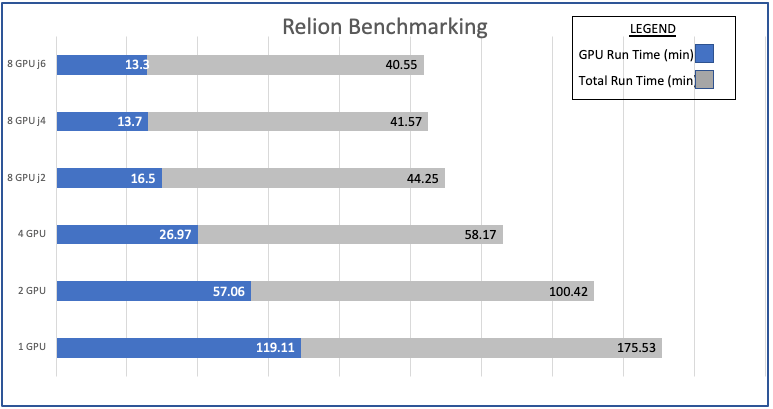
Notes on System Memory
Although a minimum of 64 GB of RAM is recommended to run RELION with small image sizes (eg. 200×200) on either the original or accelerated versions of RELION, 360×360 problems run best on systems with more than 128GB of RAM. Systems with 256GB or more RAM are recommended for the CPU-accelerated kernels on larger image sizes. Insufficient memory causes individual MPI-ranks to be killed, leading to zombie RELION jobs.
MPI Settings
Where some users may want to run more than one MPI-rank per GPU, sufficient GPU memory is needed. Each MPI-slave that shares a GPU increases the use of memory. In this case, however, it’s recommended to run a single MPI-slave per GPU for good performance and stable execution.
Note that 3D auto-refinement always needs to be run with at least 3 MPI processes (a master, and one slave for each half-set). Therefore, machines with at least two GPU cards would be preferable for refinement using GPUs. If you need to (or want to) run multiple MPI-ranks on each GPU, RELION will attempt to do so in an efficient way if you simply specify more ranks than there are GPUs.
You can run multiple threads just as with previous versions of RELION, using the --j <x> option. Each MPI process will launch the specified number of threads. This may speed up calculations, without costing much extra memory either on the CPU (RAM) or on the GPU.
For example: On a 4-GPU development machine with 16 visible cores, you would run classifications or refinements using:
mpirun -n 5 ‘which relion_refine_mpi‘ --j 4 --gpu
Which produces 4 working (slave) MPI-ranks, each with 4 threads. This produces a single rank per card but allows multiple CPU cores to use each GPU, maximizing overall hardware utilization.
Each MPI-rank requires it’s own copy of the large object in CPU and GPU memory, but if it can fit into memory it could be faster to run 2 or more MPI processes on each GPU when they can become asynchronous; one MPI process executes
calculations, while the other MPI process, for example, reads images from the disk.
Notes on Scaling
The GPUs tested were Ampere-based. As a result, it is more beneficial to scale out than scale-up. Another thing to note is the diminishing returns in scaling once you pass 4 GPUs.
Have any questions about RELION or other applications for molecular dynamics?
Contact Exxact Today
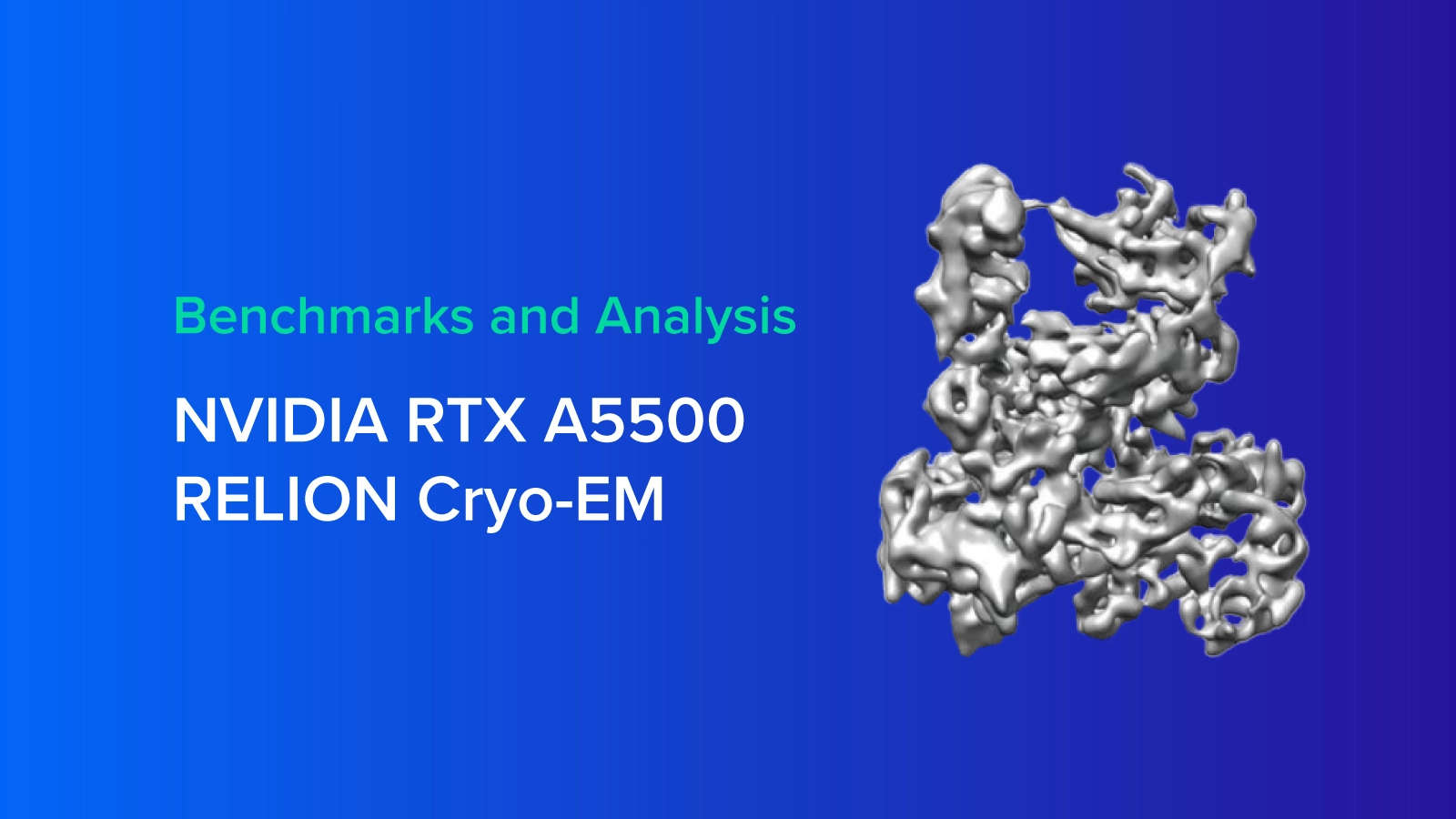
NVIDIA RTX A5500 RELION Cryo-EM Benchmarks and Analysis
NVIDIA RTX A5500 Benchmarks Overview
As a value-added supplier of scientific workstations and servers, Exxact regularly provides reference benchmarks in various GPU configurations to guide Cryogenic Electron Microscopy (cryo-EM) scientists looking to procure systems optimized for their research. In this blog, we benchmark the NVIDIA RTX A5500 performance using Relion Cryo-EM, comparing GPU runtime to Total Runtime performance.
Software Summary
RELION (REgularised LIkelihood OptimisatioN), or Relion, has revolutionized the cryo-EM field since 2012. Developed by Scheres Lab at the MRC Laboratory of Molecular Biology, this stand-alone computer program uses a Bayesian approach to refine macromolecular structures by single-particle analysis of electron cryo-microscopy data.
The development of RELION is supported through long-term funding by the UK Medical Research Council and is distributed under a GPLv2 license. This means that anyone (including commercial users) can download, use and modify RELION free of cost. The MRC Laboratory just request that if RELION is useful in your work, you will cite their papers.
RELION GPU Support Summary
With advancements in automation, computing power, and visual technology, the scope and complexity of datasets used in cryo-EM have grown substantially. GPU support and acceleration are essential for the flexibility of resource management, prevention of memory limitations, and addressing the most computationally intensive processes of cryo-EM such as image classification, and high-resolution refinement.
Exxact RTX A5500 Workstation System Specs:
Nodes | 1 |
Processor / Count | 2x AMD EPYC 7552 |
Physical Cores | 96 |
Memory | DDR4 512 GB |
Storage | NVMe 3.84 TB |
OS | Rocky 8.5 |
CUDA Version | 11.4 |
RELION Version | 4.0 |
GPU Benchmarks
Benchmarks below are 3D classifications performed using the Plasmodium ribosome dataset
- If runs are performed using the --scratch_dir option in RELION, the time to copy to scratch is subtracted from the total runtime of the command. This allows for the comparison of runs to be independent of storage pool variability.
- "GPU Time" is reported as the time spent on the Expectation step in each of the 25 iterations of classification, which provides a solid snapshot of GPU speed as this is the primary GPU compute step in RELION.
- All benchmarks in this chart are performed using N+1 MPI-ranks where N = Number of GPUS and six threads per process (--j 6). The Systems used in these results have eight GPU cards installed.
Performance Overview
- The NVIDIA RTX A5500 performs as expected showing improvements from 1 GPU through 4.
- The performance improvement drops off after $x GPUs. This is most likely because of the overhead (thread count) while using Multiple GPUs
RELION Benchmarks – GPU Run Time Performance. Note: GPUs 1, 2, and 4 had a standard thread count of 4.
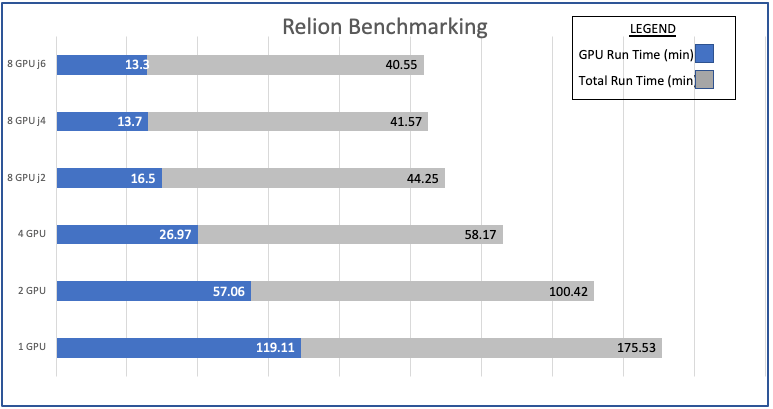
Notes on System Memory
Although a minimum of 64 GB of RAM is recommended to run RELION with small image sizes (eg. 200×200) on either the original or accelerated versions of RELION, 360×360 problems run best on systems with more than 128GB of RAM. Systems with 256GB or more RAM are recommended for the CPU-accelerated kernels on larger image sizes. Insufficient memory causes individual MPI-ranks to be killed, leading to zombie RELION jobs.
MPI Settings
Where some users may want to run more than one MPI-rank per GPU, sufficient GPU memory is needed. Each MPI-slave that shares a GPU increases the use of memory. In this case, however, it’s recommended to run a single MPI-slave per GPU for good performance and stable execution.
Note that 3D auto-refinement always needs to be run with at least 3 MPI processes (a master, and one slave for each half-set). Therefore, machines with at least two GPU cards would be preferable for refinement using GPUs. If you need to (or want to) run multiple MPI-ranks on each GPU, RELION will attempt to do so in an efficient way if you simply specify more ranks than there are GPUs.
You can run multiple threads just as with previous versions of RELION, using the --j <x> option. Each MPI process will launch the specified number of threads. This may speed up calculations, without costing much extra memory either on the CPU (RAM) or on the GPU.
For example: On a 4-GPU development machine with 16 visible cores, you would run classifications or refinements using:
mpirun -n 5 ‘which relion_refine_mpi‘ --j 4 --gpu
Which produces 4 working (slave) MPI-ranks, each with 4 threads. This produces a single rank per card but allows multiple CPU cores to use each GPU, maximizing overall hardware utilization.
Each MPI-rank requires it’s own copy of the large object in CPU and GPU memory, but if it can fit into memory it could be faster to run 2 or more MPI processes on each GPU when they can become asynchronous; one MPI process executes
calculations, while the other MPI process, for example, reads images from the disk.
Notes on Scaling
The GPUs tested were Ampere-based. As a result, it is more beneficial to scale out than scale-up. Another thing to note is the diminishing returns in scaling once you pass 4 GPUs.
Have any questions about RELION or other applications for molecular dynamics?
Contact Exxact Today