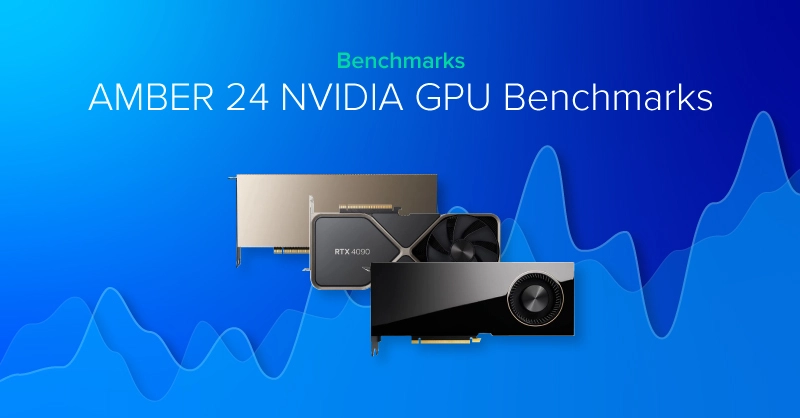
Updated 12/26/24 to add Grace Hopper GH200 Benchmark Numbers
AMBER 24 GPU Benchmarks on NVIDIA GeForce, RTX, and Data Center GPUs
*All benchmarks were performed using a single GPU configuration using Amber 24 & AmberTools 24 on NVIDIA CUDA 12.3 which could explain the slight increase in performance from Amber 22.
**NVIDIA GeForce and RTX GPUs were tested on an Exxact workstation and can have a maximum of a 2-way configuration. All other NVIDIA Professional GPUs (RTX and Data Center GPUs) are tested in an Exxact server and support 8-way GPU configuration.
*** Since AMBER computations are only performed by GPUs via CUDA, the variation between CPUs from workstation and server systems have little to no effect on throughput between benchmarks.
Quick AMBER GPU Benchmark Takeaways
NVIDIA Ada Lovelace Generation GPUs outperform all Ampere Generation GPUs. Ada Generation GPUs have increased performance and energy efficiency, which are worth the increase in price over Ampere.
- NVIDIA GH200 offers lackluster performance on less complex simulations with fewer atoms. However, on larger simulations and implicit solvent models, GH200 shines, outperforming most other GPUs.
- NVIDIA RTX 4090 offers the best performance, but due to the physical GPU card size and thus the lack of multi-GPU scalability, it is at a disadvantage.
- NVIDIA RTX 6000 Ada offers similar performance to the RTX 4090 but trades lower clock speed for better reliability. RTX 6000 Ada also has a larger memory capacity of 48GB and is multi-GPU scalable, making it better for larger simulation modeling and running calculations in parallel.
- RTX 5000 and 4500 are performing well above last generation's flagship RTX A6000. These might be the new best GPUs for AMBER with a great cost-to-performance ratio.
- Even the mid-range consumer card RTX 4070 Ti shows considerable performance gains over the last generation flagship RTX 3090
The NVIDIA H100 is on par as the 3rd most powerful GPU (behind the RTX 4090 and RTX 6000 Ada), winning in only a couple of tests. The H100 is more geared towards AI workloads, and its high price tag makes it a not worthwhile GPU for simulation-only workloads.
For the larger simulations, such as STMV Production NPT 4fs, the high-speed memory, memory capacity, and GPU clock speed play a large factor in performance. GH200, H100, RTX 6000, and 4090 dominate in this department.
For smaller simulations, the options are more varied. The 4070 Ti shows promising performance, but the RTX 5000 Ada and RTX 4080 deliver exceptional performance, trailing behind the bigger and better RTX 6000 and RTX 4090.
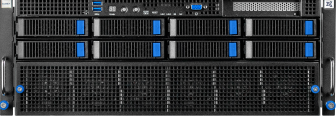
We're Here to Deliver the Tools to Power Your Research
With access to the highest-performing hardware, at Exxact, we offer customizable platforms for AMBER optimized for your deployment, budget, and desired performance so you can make an impact with your research!
Configure your Ideal GPU System for AMBERBenchmark Hardware and Specifications
GPU Benchmarked
GeForce | RTX (Quadro) | Data Center (Tesla) |
Benchmark | RTX 6000 Ada | RTX 5000 Ada | RTX 4500 Ada | GH200 Superchip | H100 PCIe | RTX 4090 | RTX 4080 | RTX 4070 Ti | A100 PCIe | RTX A6000 | RTX A5500 | RTX A5000 | RTX A4500 | RTX A4000 | RTX 3090 | RTX 3080 |
JAC Production NVE 4fs | 1697.34 | 1562.48 | 1297.88 | 1323.31 | 1532.08 | 1706.21 | 1596.79 | 1385.68 | 1226.40 | 1132.86 | 1116.01 | 1029.89 | 963.52 | 841.32 | 1228.41 | 1160.34 |
JAC Production NPT 4fs | 1666.84 | 1550.32 | 1278.02 | 1322.17 | 1500.37 | 1641.18 | 1598.79 | 1293.92 | 1257.77 | 1117.95 | 1126.87 | 1025.84 | 951.60 | 829.49 | 1197.09 | 1158.95 |
JAC Production NVE 2fs | 917.70 | 843.16 | 698.69 | 759.44 | 806.39 | 934.00 | 868.79 | 740.55 | 642.79 | 615.92 | 596.22 | 559.03 | 518.54 | 448.53 | 655.52 | 608.60 |
JAC Production NPT 2fs | 906.35 | 835.59 | 693.10 | 686.54 | 752.49 | 915.99 | 843.32 | 722.34 | 654.20 | 601.67 | 586.07 | 544.16 | 521.92 | 443.81 | 643.68 | 599.03 |
FactorIX Production NVE 2fs | 489.93 | 406.98 | 306.57 | 191.85 | 410.77 | 488.16 | 400.22 | 315.32 | 283.70 | 273.64 | 242.84 | 225.58 | 201.79 | 161.63 | 276.82 | 246.65 |
FactorIX Production NPT 2fs | 442.91 | 376.67 | 288.13 | 206.06 | 385.12 | 471.74 | 377.42 | 299.88 | 264.03 | 253.98 | 233.43 | 216.11 | 193.83 | 158.24 | 262.36 | 234.06 |
Cellulose Production NVE 2fs | 123.98 | 95.91 | 67.63 | 167.20 | 125.82 | 136.85 | 96.16 | 72.90 | 90.17 | 63.15 | 55.07 | 49.63 | 42.14 | 33.57 | 67.08 | 57.07 |
Cellulose Production NPT 2fs | 114.99 | 92.32 | 63.78 | 152.40 | 113.81 | 125.63 | 91.30 | 68.14 | 82.74 | 58.00 | 52.03 | 47.86 | 40.33 | 31.89 | 60.81 | 51.68 |
STMV Production NPT 4fs | 70.97 | 55.30 | 37.58 | 101.31 | 74.50 | 82.60 | 57.99 | 39.36 | 53.84 | 39.08 | 35.12 | 32.29 | 27.66 | 21.87 | 41.05 | 34.05 |
TRPCage GB 2fs | 1477.12 | 1448.25 | 1424.88 | 1524.52 | 1399.51 | 1491.75 | 1578.44 | 1512.26 | 1027.35 | 1145.56 | 1176.41 | 1209.86 | 1175.60 | 1248.80 | 1231.97 | 1348.60 |
Myoglobin GB 2fs | 1016.00 | 841.93 | 740.65 | 1159.35 | 1094.57 | 888.21 | 843.83 | 772.38 | 656.65 | 648.58 | 592.84 | 580.02 | 536.57 | 491.05 | 614.32 | 624.68 |
Nucleosome GB 2fs | 31.59 | 26.11 | 18.80 | 37.24 | 37.83 | 35.90 | 27.60 | 20.87 | 29.60 | 19.70 | 15.32 | 15.18 | 11.58 | 10.98 | 21.12 | 17.60 |
JAC Production NVE 4fs - 23,558 Atoms
JAC Production NPT 4fs- 23,558 Atoms
JAC Production NVE 2fs - 23,558 Atoms
JAC Production NPT 2fs - 23,558 Atoms
FactorIX Production NVE 2FS - 90,906 Atoms
FactorIX Production NPT 2fs - 90,906 Atoms
Cellulose Production NVE 2fs - 408,609 Atoms
Cellulose Production NPT 2fs - 408,609 Atoms
STMV Production NPT 4fs - 1,067,095 Atoms
TRPCage Production GB - 304 Atoms [Implicit]
Myoglobin Production GB - 2,492 Atoms [Implicit]
Nucleosome Production GB - 25,095 Atoms [Implicit]
AMBER 24 Background & Hardware Recommendations
AMBER consists of several different software packages with the molecular dynamics engine PMEMD as the most compute-intensive and the engine we want to optimize the most. This consists of single CPU (pmemd), multi-CPU (pmemd.MPI), single-GPU (pmemd.cuda) and multi-GPU (pmemd.cuda.MPI) versions. Traditionally, MD simulations are executed on CPUs. However, the increased use of GPUs and native support to run AMBER MD simulations on CUDA have made GPUs the most logical choice for speed and cost efficiency.
Most AMBER simulations can fit on a single GPU and run strictly on CUDA, thus the CPU, CPU memory (RAM), and storage speed have little to no influence on simulation throughput performance. Running simulations on a single GPU means that parallelizing multi-GPUs on a single calculation won’t incur much speed up. To fully utilize a multi-GPU or multi-node deployment is to run multiple independent AMBER simulations simultaneously on multiple GPUs in the same node or on different nodes.
Hardware Recommendation
Our top 3 GPU recommendations for running AMBER and our reasonings:
- For cost-effective parallel computing, the RTX 5000 Ada or the RTX 4500 Ada offers A-tier and B-tier performance for much lower cost compared with the RTX 6000 Ada. The additional cost of the RTX 6000 Ada stems from the better performance and larger memory, which won’t be utilized in most AMBER calculations. The extra cost can be allocated to more GPUs and thus more calculations running in parallel. A deployment with 8x RTX 4500 Ada GPUs is similar in price to a deployment with 4x RTX 6000 Ada GPUs, but can drastically parallelize your workflow.
- For peak single GPU throughput with smaller teams would be the NVIDIA RTX 4090 with its S+ tier performance. If you don’t need to run multiple simulations simultaneously, the RTX 4090 delivers the fastest results.
- For peak throughput and parallel computing, the RTX 6000 Ada GPU delivers S-tier performance akin to the RTX 4090 but allows deployments to slot 4x GPUs in a 2U node or 8x GPUs in a 4U node.
Our CPU & Memory Recommendation
- There is no need to overspend on a CPU since it will not run the calculations. The bare minimum would be to allocate a CPU core for every GPU in the system. Additional GPUs require dual CPUs for additional PCIe lanes.
- Recommended RAM would be 32GB per GPU. You can get by with 16GB of RAM per GPU as well.
Conclusion
Not all use cases are the same and AMBER is most likely not the only application used in your research. At Exxact Corp., we strive to provide the resources to configure the best custom system fit for you.
Since AMBER’s performance is not highly affected by the different setups, you may benefit from optimizing your system to other more selective application requirements that you may also use. Applications like GROMACS or NAMD can benefit from additional cores or higher-end CPUs and can be a tradeoff that can benefit other workflows.
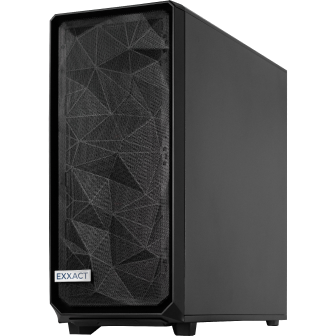
We're Here to Deliver the Tools to Power Your Research
With access to the highest performing hardware, at Exxact, we can offer the platform optimized for your deployment, budget, and desired performance so you can make an impact with your research!
Configure your Life Science Solution Today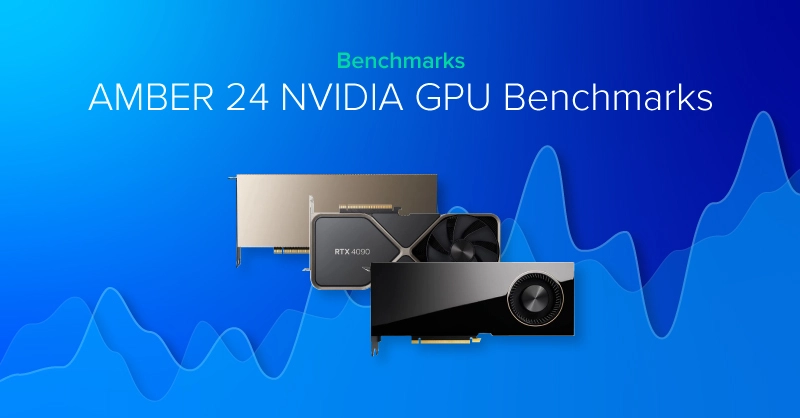
AMBER 24 NVIDIA GPU Benchmarks
Updated 12/26/24 to add Grace Hopper GH200 Benchmark Numbers
AMBER 24 GPU Benchmarks on NVIDIA GeForce, RTX, and Data Center GPUs
*All benchmarks were performed using a single GPU configuration using Amber 24 & AmberTools 24 on NVIDIA CUDA 12.3 which could explain the slight increase in performance from Amber 22.
**NVIDIA GeForce and RTX GPUs were tested on an Exxact workstation and can have a maximum of a 2-way configuration. All other NVIDIA Professional GPUs (RTX and Data Center GPUs) are tested in an Exxact server and support 8-way GPU configuration.
*** Since AMBER computations are only performed by GPUs via CUDA, the variation between CPUs from workstation and server systems have little to no effect on throughput between benchmarks.
Quick AMBER GPU Benchmark Takeaways
NVIDIA Ada Lovelace Generation GPUs outperform all Ampere Generation GPUs. Ada Generation GPUs have increased performance and energy efficiency, which are worth the increase in price over Ampere.
- NVIDIA GH200 offers lackluster performance on less complex simulations with fewer atoms. However, on larger simulations and implicit solvent models, GH200 shines, outperforming most other GPUs.
- NVIDIA RTX 4090 offers the best performance, but due to the physical GPU card size and thus the lack of multi-GPU scalability, it is at a disadvantage.
- NVIDIA RTX 6000 Ada offers similar performance to the RTX 4090 but trades lower clock speed for better reliability. RTX 6000 Ada also has a larger memory capacity of 48GB and is multi-GPU scalable, making it better for larger simulation modeling and running calculations in parallel.
- RTX 5000 and 4500 are performing well above last generation's flagship RTX A6000. These might be the new best GPUs for AMBER with a great cost-to-performance ratio.
- Even the mid-range consumer card RTX 4070 Ti shows considerable performance gains over the last generation flagship RTX 3090
The NVIDIA H100 is on par as the 3rd most powerful GPU (behind the RTX 4090 and RTX 6000 Ada), winning in only a couple of tests. The H100 is more geared towards AI workloads, and its high price tag makes it a not worthwhile GPU for simulation-only workloads.
For the larger simulations, such as STMV Production NPT 4fs, the high-speed memory, memory capacity, and GPU clock speed play a large factor in performance. GH200, H100, RTX 6000, and 4090 dominate in this department.
For smaller simulations, the options are more varied. The 4070 Ti shows promising performance, but the RTX 5000 Ada and RTX 4080 deliver exceptional performance, trailing behind the bigger and better RTX 6000 and RTX 4090.
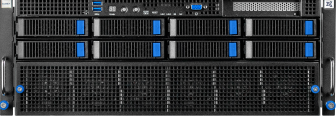
We're Here to Deliver the Tools to Power Your Research
With access to the highest-performing hardware, at Exxact, we offer customizable platforms for AMBER optimized for your deployment, budget, and desired performance so you can make an impact with your research!
Configure your Ideal GPU System for AMBERBenchmark Hardware and Specifications
GPU Benchmarked
GeForce | RTX (Quadro) | Data Center (Tesla) |
Benchmark | RTX 6000 Ada | RTX 5000 Ada | RTX 4500 Ada | GH200 Superchip | H100 PCIe | RTX 4090 | RTX 4080 | RTX 4070 Ti | A100 PCIe | RTX A6000 | RTX A5500 | RTX A5000 | RTX A4500 | RTX A4000 | RTX 3090 | RTX 3080 |
JAC Production NVE 4fs | 1697.34 | 1562.48 | 1297.88 | 1323.31 | 1532.08 | 1706.21 | 1596.79 | 1385.68 | 1226.40 | 1132.86 | 1116.01 | 1029.89 | 963.52 | 841.32 | 1228.41 | 1160.34 |
JAC Production NPT 4fs | 1666.84 | 1550.32 | 1278.02 | 1322.17 | 1500.37 | 1641.18 | 1598.79 | 1293.92 | 1257.77 | 1117.95 | 1126.87 | 1025.84 | 951.60 | 829.49 | 1197.09 | 1158.95 |
JAC Production NVE 2fs | 917.70 | 843.16 | 698.69 | 759.44 | 806.39 | 934.00 | 868.79 | 740.55 | 642.79 | 615.92 | 596.22 | 559.03 | 518.54 | 448.53 | 655.52 | 608.60 |
JAC Production NPT 2fs | 906.35 | 835.59 | 693.10 | 686.54 | 752.49 | 915.99 | 843.32 | 722.34 | 654.20 | 601.67 | 586.07 | 544.16 | 521.92 | 443.81 | 643.68 | 599.03 |
FactorIX Production NVE 2fs | 489.93 | 406.98 | 306.57 | 191.85 | 410.77 | 488.16 | 400.22 | 315.32 | 283.70 | 273.64 | 242.84 | 225.58 | 201.79 | 161.63 | 276.82 | 246.65 |
FactorIX Production NPT 2fs | 442.91 | 376.67 | 288.13 | 206.06 | 385.12 | 471.74 | 377.42 | 299.88 | 264.03 | 253.98 | 233.43 | 216.11 | 193.83 | 158.24 | 262.36 | 234.06 |
Cellulose Production NVE 2fs | 123.98 | 95.91 | 67.63 | 167.20 | 125.82 | 136.85 | 96.16 | 72.90 | 90.17 | 63.15 | 55.07 | 49.63 | 42.14 | 33.57 | 67.08 | 57.07 |
Cellulose Production NPT 2fs | 114.99 | 92.32 | 63.78 | 152.40 | 113.81 | 125.63 | 91.30 | 68.14 | 82.74 | 58.00 | 52.03 | 47.86 | 40.33 | 31.89 | 60.81 | 51.68 |
STMV Production NPT 4fs | 70.97 | 55.30 | 37.58 | 101.31 | 74.50 | 82.60 | 57.99 | 39.36 | 53.84 | 39.08 | 35.12 | 32.29 | 27.66 | 21.87 | 41.05 | 34.05 |
TRPCage GB 2fs | 1477.12 | 1448.25 | 1424.88 | 1524.52 | 1399.51 | 1491.75 | 1578.44 | 1512.26 | 1027.35 | 1145.56 | 1176.41 | 1209.86 | 1175.60 | 1248.80 | 1231.97 | 1348.60 |
Myoglobin GB 2fs | 1016.00 | 841.93 | 740.65 | 1159.35 | 1094.57 | 888.21 | 843.83 | 772.38 | 656.65 | 648.58 | 592.84 | 580.02 | 536.57 | 491.05 | 614.32 | 624.68 |
Nucleosome GB 2fs | 31.59 | 26.11 | 18.80 | 37.24 | 37.83 | 35.90 | 27.60 | 20.87 | 29.60 | 19.70 | 15.32 | 15.18 | 11.58 | 10.98 | 21.12 | 17.60 |
JAC Production NVE 4fs - 23,558 Atoms
JAC Production NPT 4fs- 23,558 Atoms
JAC Production NVE 2fs - 23,558 Atoms
JAC Production NPT 2fs - 23,558 Atoms
FactorIX Production NVE 2FS - 90,906 Atoms
FactorIX Production NPT 2fs - 90,906 Atoms
Cellulose Production NVE 2fs - 408,609 Atoms
Cellulose Production NPT 2fs - 408,609 Atoms
STMV Production NPT 4fs - 1,067,095 Atoms
TRPCage Production GB - 304 Atoms [Implicit]
Myoglobin Production GB - 2,492 Atoms [Implicit]
Nucleosome Production GB - 25,095 Atoms [Implicit]
AMBER 24 Background & Hardware Recommendations
AMBER consists of several different software packages with the molecular dynamics engine PMEMD as the most compute-intensive and the engine we want to optimize the most. This consists of single CPU (pmemd), multi-CPU (pmemd.MPI), single-GPU (pmemd.cuda) and multi-GPU (pmemd.cuda.MPI) versions. Traditionally, MD simulations are executed on CPUs. However, the increased use of GPUs and native support to run AMBER MD simulations on CUDA have made GPUs the most logical choice for speed and cost efficiency.
Most AMBER simulations can fit on a single GPU and run strictly on CUDA, thus the CPU, CPU memory (RAM), and storage speed have little to no influence on simulation throughput performance. Running simulations on a single GPU means that parallelizing multi-GPUs on a single calculation won’t incur much speed up. To fully utilize a multi-GPU or multi-node deployment is to run multiple independent AMBER simulations simultaneously on multiple GPUs in the same node or on different nodes.
Hardware Recommendation
Our top 3 GPU recommendations for running AMBER and our reasonings:
- For cost-effective parallel computing, the RTX 5000 Ada or the RTX 4500 Ada offers A-tier and B-tier performance for much lower cost compared with the RTX 6000 Ada. The additional cost of the RTX 6000 Ada stems from the better performance and larger memory, which won’t be utilized in most AMBER calculations. The extra cost can be allocated to more GPUs and thus more calculations running in parallel. A deployment with 8x RTX 4500 Ada GPUs is similar in price to a deployment with 4x RTX 6000 Ada GPUs, but can drastically parallelize your workflow.
- For peak single GPU throughput with smaller teams would be the NVIDIA RTX 4090 with its S+ tier performance. If you don’t need to run multiple simulations simultaneously, the RTX 4090 delivers the fastest results.
- For peak throughput and parallel computing, the RTX 6000 Ada GPU delivers S-tier performance akin to the RTX 4090 but allows deployments to slot 4x GPUs in a 2U node or 8x GPUs in a 4U node.
Our CPU & Memory Recommendation
- There is no need to overspend on a CPU since it will not run the calculations. The bare minimum would be to allocate a CPU core for every GPU in the system. Additional GPUs require dual CPUs for additional PCIe lanes.
- Recommended RAM would be 32GB per GPU. You can get by with 16GB of RAM per GPU as well.
Conclusion
Not all use cases are the same and AMBER is most likely not the only application used in your research. At Exxact Corp., we strive to provide the resources to configure the best custom system fit for you.
Since AMBER’s performance is not highly affected by the different setups, you may benefit from optimizing your system to other more selective application requirements that you may also use. Applications like GROMACS or NAMD can benefit from additional cores or higher-end CPUs and can be a tradeoff that can benefit other workflows.
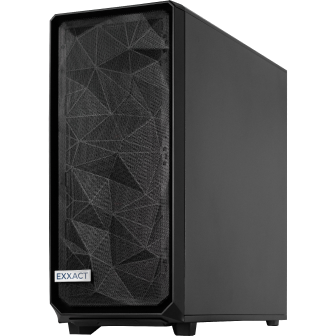
We're Here to Deliver the Tools to Power Your Research
With access to the highest performing hardware, at Exxact, we can offer the platform optimized for your deployment, budget, and desired performance so you can make an impact with your research!
Configure your Life Science Solution Today