
In recent years, Artificial Intelligence (AI) has played a more increasingly important role in the field of genomics, the study of an organism's genetic material. With the advancements in AI and machine learning, scientists can now analyze vast amounts of genomic data more accurately and efficiently than ever before. This has led to a wealth of new insights into the workings of our genetic code and has enabled the development of novel therapies for genetic diseases and healthcare innovations.
In this article, we discuss some key application areas and how AI is transforming genomics, leading to rapid breakthroughs in the healthcare and drug discovery field.
DNA / Gene Sequence Analysis
One of the most significant applications of AI in genomics is in the analysis of DNA sequences. DNA sequencing technology has advanced rapidly in recent years, and researchers can now generate vast amounts of genomic data in a short amount of time.
However, analyzing this data can be a daunting task, as it requires the identification of patterns and correlations across millions of base pairs. AI algorithms can help automate this process by identifying genetic variations and potentially associating them with specific diseases.
For example, in a study published in Nature Journal, researchers used AI to analyze the genomic data of over 4,000 people with autism. The algorithm identified several new genes that were associated with the condition, which could help researchers develop new therapies in the future.
In our case study with the University of Montreal Dr. Dumas and his team use AI and Machine Learning to study the effect of genome architecture and its relationship to constrained brain development.
AI algorithms can help in the assembly of the genome from large amounts of short-read sequencing data. For example, the AI-based software called Canu can assemble long-read sequences accurately and efficiently. Multiple various machine learning models are highly performant and fast in detecting differences in genomes that the human eye cannot.
AI can also help identify variants in the genome by comparing sequencing data with a reference genome. DeepVariant, an AI-based variant caller, uses a convolutional neural network to make accurate variant calls.
Not only DNA but, AI can also analyze RNA sequencing data to determine the different types and levels of gene expression. For instance, the AI-based software called Kallisto can accurately quantify gene expression levels. This is called Transcriptome analysis and it is fielding AI tools increasingly.
For running highly performant analyses of genomes, execute your workloads on Exxact AI Workstations and Deep Learning AI Servers built to accelerate the most strenuous projects.
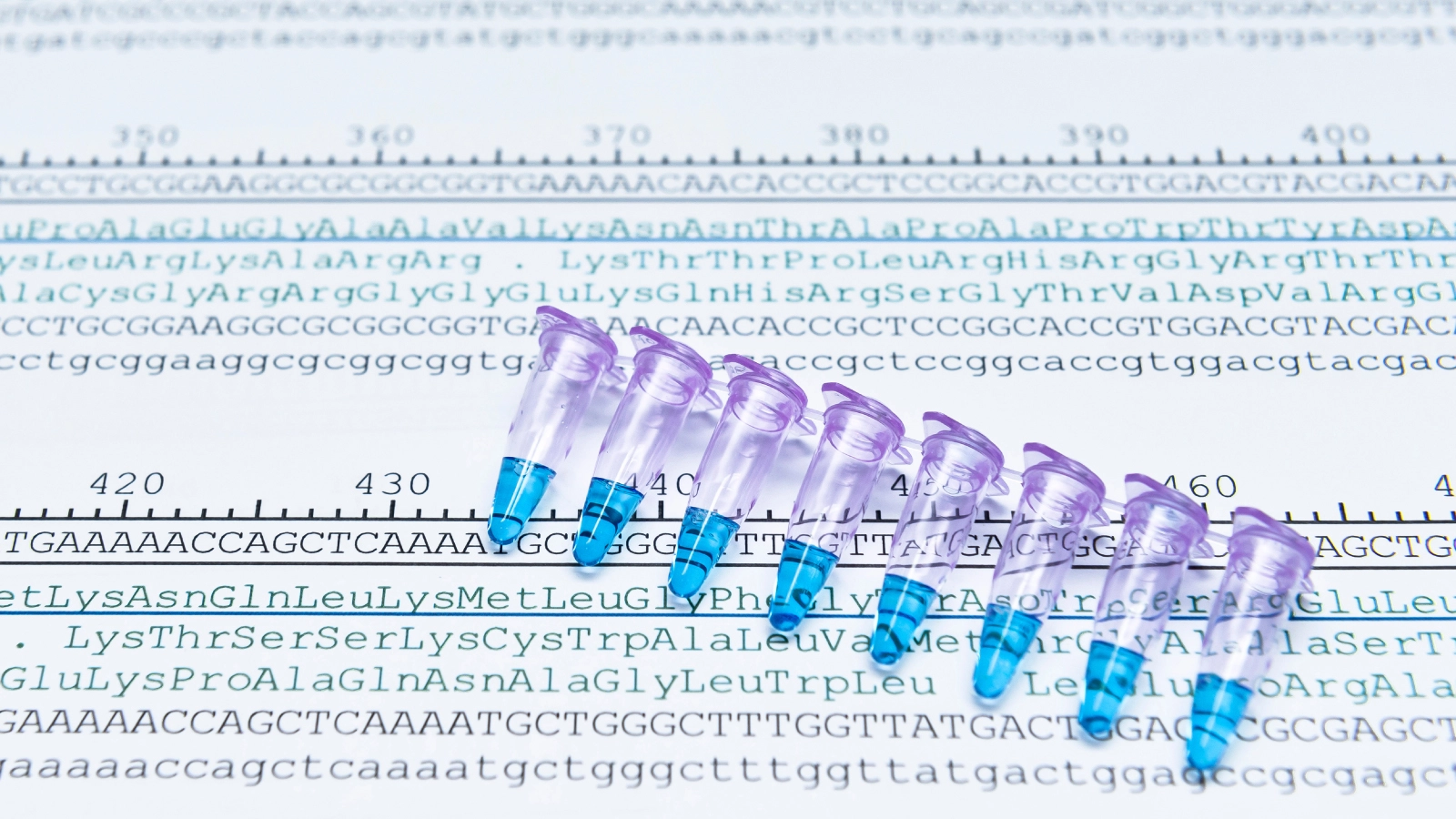
Drug Discovery and Clinical Diagnosis
AI is being used to identify new drug targets and develop new drugs. For example, the startup Atomwise uses AI to identify new drug candidates by predicting the binding of small molecules to target proteins. Moreover, AI can assist in the diagnosis of genetic diseases by analyzing genetic data and identifying disease-causing mutations.
At the University of Toronto, the Acceleration Consortium used genomics and the AI-folded protein database AlphaFold to discover a potential drug candidate to treat liver cancer in just 30 days. The capabilities of AI being used to discover these new drug candidates at rapid and record speed reduces the need to screen thousands of maybes. The process that used to take years could shrink down to weeks.
Individually Personalized Medicine
Another related area where AI is having a significant impact is the development of precision medicine. Precision medicine aims to provide personalized treatments for patients based on their unique genetic makeup. With AI, companies like Deep Genomics can now analyze genomic data to identify genetic mutations that are driving a patient's disease and develop targeted therapies to treat those mutations.
In a study published in the journal Human Genetics in 2019, authors reviewed the status and future directions of AI applications in cancer genomics within the context of workflows to integrate genomic analysis for precision cancer care. The existing solutions of AI and their limitations in cancer genetic testing and diagnostics such as variant calling and interpretation are critically analyzed.
Some more key breakthrough research are cited below,
- Diagnosis of Skin Cancer: AI has been utilized to enhance the diagnosis of skin cancer. Researchers at Stanford University developed a deep learning algorithm that could identify skin cancer with an accuracy rate of 91%. The study was published in the Nature Journal in 2017. (Esteva et al., 2017)
- Prognosis of Breast Cancer: AI has also been used to predict the prognosis of breast cancer. Detailed applications of AI tools and techniques for breast cancer imaging and prognosis application is published in Nature Communications in 2022 (Koh et al., 2022).
- Diagnosis of Alzheimer's Disease: AI has been used to enhance the diagnosis of Alzheimer's disease. A robust framework to investigate the reliability and stability of explainable artificial intelligence markers of Mild Cognitive Impairment and Alzheimer’s Disease was developed by researchers at the Università degli Studi di Bari Aldo Moro. The study was published in the journal "Brain Informatics" in 2022. (Lombardi et al., 2022)
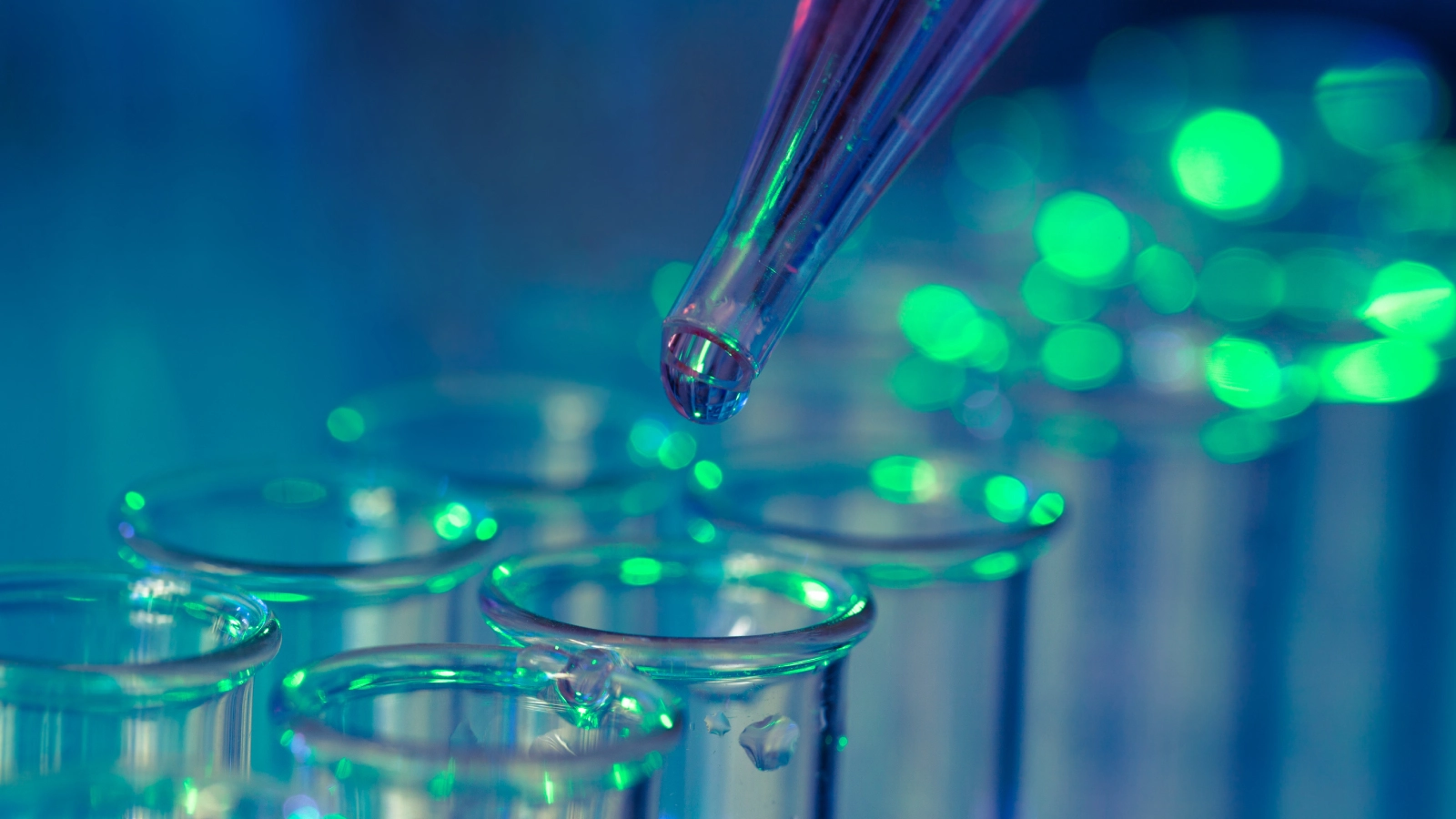
Gene Editing
AI is also playing a role in the development of gene editing technologies, which allow researchers to modify the genetic code of living organisms. One of the most promising gene editing technologies is CRISPR-Cas9, which uses a system of molecular scissors to cut and edit DNA.
In a study published in the journal Cancer Informatics in 2022, researchers used AI to identify new CRISPR-Cas9 targets that could be used to edit the genomes of plants. The use of artificial intelligence (AI) capacity to edit genomes through CRISPR/Cas9 enables modification of gene mutations and molecular simulation.
AI approaches include knowledge discovery approaches, antigen and epitope prediction approaches, and agent-based-model approaches. These methods in combination with CRISPR/Cas9 can be used in vaccine design.
Protein and Gene Network Analysis
AI is also being used to analyze the complex networks of genes and proteins that underlie many diseases. By analyzing these networks, researchers can identify the key genes and proteins that are involved in disease progression and develop new therapies to target those molecules.
In a study published in the journal Aging and Disease in 2020, researchers used AI to analyze the network of genes and proteins involved in Alzheimer's disease. A comprehensive review is focused on the application of AI in the genetic research of AD, including the diagnosis and prognosis of AD based on genetic data, the analysis of genetic variation, gene expression profile, gene-gene interaction in AD, and genetic analysis of AD based on a knowledge base.
Don't overlook the advantages AMD EPYC 9004 offers to your groundbreaking research. Experience EPYC performance for yourself with Exxact servers powered by AMD EPYC 9004.
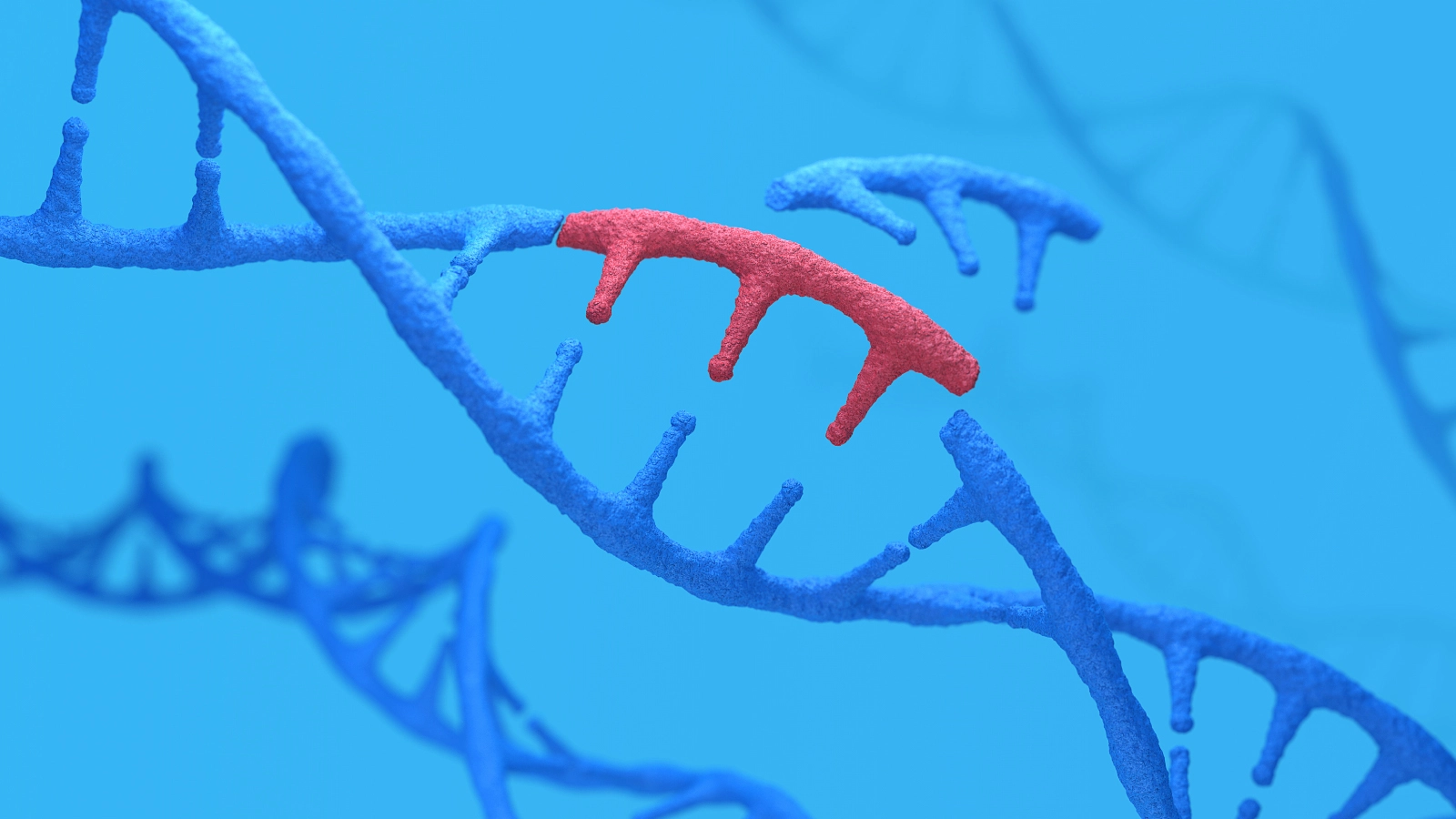
Key Research References
- Artificial Intelligence to guide precision anticancer therapy with multitargeted kinase inhibitors: In this study, researchers proposed a novel graph deep learning technique - CancerOmicsNet. This network integrates multiple heterogeneous data by utilizing a deep graph learning model with sophisticated attention propagation mechanisms to extract highly predictive features from cancer-specific networks. The AI-based system was devised to provide more accurate and robust predictions than data-driven therapeutic discovery using gene signature reversion.
- DeepTorrent: a deep learning-based approach for predicting DNA N4-methylcytosine sites: In this study, researchers proposed a deep learning-based approach, called DeepTorrent, for improved prediction of 4mC sites from DNA sequences. It combines four different feature encoding schemes to encode raw DNA sequences and employs multi-layer convolutional neural networks with an inception module integrated with bidirectional long short-term memory to effectively learn the higher-order feature representations., which could help researchers better understand genetic diseases.
- Artificial Intelligence in drug development: present status and future prospects: At present, the pharmaceutical industry is facing challenges in sustaining its drug development programs because of increased R&D costs and reduced efficiency. In this review, we discuss the major causes of attrition rates in new drug approvals, the possible ways that AI can improve the efficiency of the drug development process, and the collaboration of pharmaceutical industry giants with AI-powered drug discovery firms.
- DeepChrome: deep-learning for predicting gene expression from histone modifications: In this study, researchers developed a unified discriminative framework using a deep convolutional neural network to classify gene expression using histone modification data as input. Their novel framework, called DeepChrome, allows the automatic extraction of complex interactions among important features. This could potentially help researchers better understand gene regulation.
- Cutting-Edge AI Technologies Meet Precision Medicine to Improve Cancer Care: To provide precision medicine for better cancer care, researchers must work on clinical patient data, such as electronic medical records, computerized tomography scans, physiological eval results, biochemistry lab results, digital pathology, and the genetic landscape. To interpret this biodata in cancer genomics, an operational flow based on AI and ML models and medical management platforms with high-performance computing must be set up for precision cancer genomics in clinical practice. This is a review article covering recent developments in these areas.
What is the Future of AI in Genomics?
One of the most significant areas where AI technologies are being utilized is cancer care, where they help in identifying the specific genetic mutations that cause cancer and developing personalized treatments. Precision medicine, which involves using genetic information to tailor medical treatments to individual patients, is another area where AI and machine learning are making a difference.
These technologies are also being used to analyze gene expression patterns and identify disease biomarkers, which can aid in early diagnosis and treatment. The role of AI and machine learning in drug discovery, including the prediction of potential drug targets and the optimization of drug design is becoming more significant every day.
As computers advance and accelerate large computationally heavy workloads, AI and machine learning will continue to revolutionize healthcare research. Cancer care, precision medicine, gene expression analysis, and drug discovery all are made possible with better hardware, and better machine learning models. With further advancements in these technologies, it is likely that their impact on genomics research will soon become groundbreaking scientific marvels.
Combine your drug discovery and genomics workflow with AI to boost your capabilities.
Contact us today to learn more!

How AI is Used in the Healthcare and Genomics Industry
In recent years, Artificial Intelligence (AI) has played a more increasingly important role in the field of genomics, the study of an organism's genetic material. With the advancements in AI and machine learning, scientists can now analyze vast amounts of genomic data more accurately and efficiently than ever before. This has led to a wealth of new insights into the workings of our genetic code and has enabled the development of novel therapies for genetic diseases and healthcare innovations.
In this article, we discuss some key application areas and how AI is transforming genomics, leading to rapid breakthroughs in the healthcare and drug discovery field.
DNA / Gene Sequence Analysis
One of the most significant applications of AI in genomics is in the analysis of DNA sequences. DNA sequencing technology has advanced rapidly in recent years, and researchers can now generate vast amounts of genomic data in a short amount of time.
However, analyzing this data can be a daunting task, as it requires the identification of patterns and correlations across millions of base pairs. AI algorithms can help automate this process by identifying genetic variations and potentially associating them with specific diseases.
For example, in a study published in Nature Journal, researchers used AI to analyze the genomic data of over 4,000 people with autism. The algorithm identified several new genes that were associated with the condition, which could help researchers develop new therapies in the future.
In our case study with the University of Montreal Dr. Dumas and his team use AI and Machine Learning to study the effect of genome architecture and its relationship to constrained brain development.
AI algorithms can help in the assembly of the genome from large amounts of short-read sequencing data. For example, the AI-based software called Canu can assemble long-read sequences accurately and efficiently. Multiple various machine learning models are highly performant and fast in detecting differences in genomes that the human eye cannot.
AI can also help identify variants in the genome by comparing sequencing data with a reference genome. DeepVariant, an AI-based variant caller, uses a convolutional neural network to make accurate variant calls.
Not only DNA but, AI can also analyze RNA sequencing data to determine the different types and levels of gene expression. For instance, the AI-based software called Kallisto can accurately quantify gene expression levels. This is called Transcriptome analysis and it is fielding AI tools increasingly.
For running highly performant analyses of genomes, execute your workloads on Exxact AI Workstations and Deep Learning AI Servers built to accelerate the most strenuous projects.
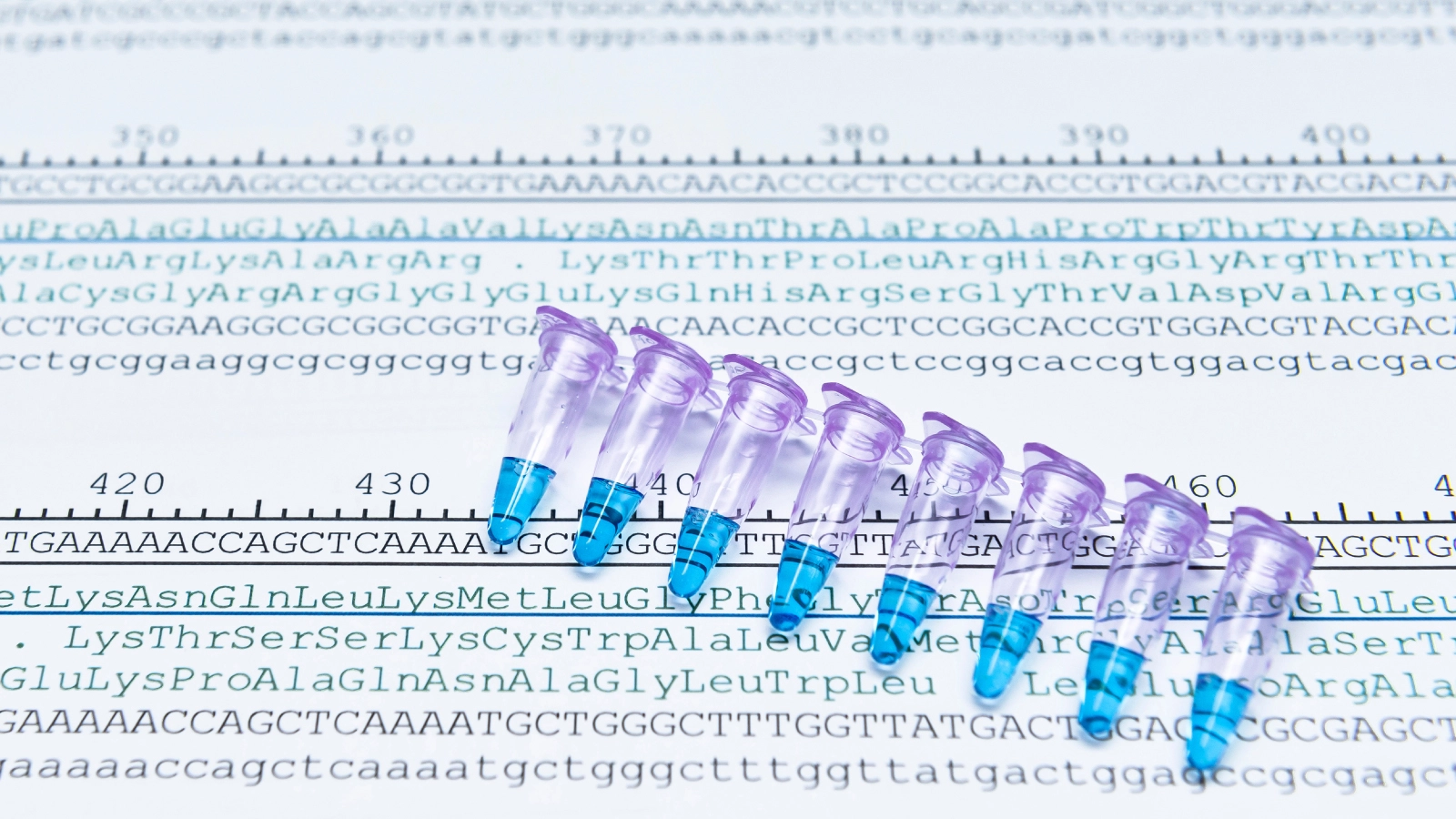
Drug Discovery and Clinical Diagnosis
AI is being used to identify new drug targets and develop new drugs. For example, the startup Atomwise uses AI to identify new drug candidates by predicting the binding of small molecules to target proteins. Moreover, AI can assist in the diagnosis of genetic diseases by analyzing genetic data and identifying disease-causing mutations.
At the University of Toronto, the Acceleration Consortium used genomics and the AI-folded protein database AlphaFold to discover a potential drug candidate to treat liver cancer in just 30 days. The capabilities of AI being used to discover these new drug candidates at rapid and record speed reduces the need to screen thousands of maybes. The process that used to take years could shrink down to weeks.
Individually Personalized Medicine
Another related area where AI is having a significant impact is the development of precision medicine. Precision medicine aims to provide personalized treatments for patients based on their unique genetic makeup. With AI, companies like Deep Genomics can now analyze genomic data to identify genetic mutations that are driving a patient's disease and develop targeted therapies to treat those mutations.
In a study published in the journal Human Genetics in 2019, authors reviewed the status and future directions of AI applications in cancer genomics within the context of workflows to integrate genomic analysis for precision cancer care. The existing solutions of AI and their limitations in cancer genetic testing and diagnostics such as variant calling and interpretation are critically analyzed.
Some more key breakthrough research are cited below,
- Diagnosis of Skin Cancer: AI has been utilized to enhance the diagnosis of skin cancer. Researchers at Stanford University developed a deep learning algorithm that could identify skin cancer with an accuracy rate of 91%. The study was published in the Nature Journal in 2017. (Esteva et al., 2017)
- Prognosis of Breast Cancer: AI has also been used to predict the prognosis of breast cancer. Detailed applications of AI tools and techniques for breast cancer imaging and prognosis application is published in Nature Communications in 2022 (Koh et al., 2022).
- Diagnosis of Alzheimer's Disease: AI has been used to enhance the diagnosis of Alzheimer's disease. A robust framework to investigate the reliability and stability of explainable artificial intelligence markers of Mild Cognitive Impairment and Alzheimer’s Disease was developed by researchers at the Università degli Studi di Bari Aldo Moro. The study was published in the journal "Brain Informatics" in 2022. (Lombardi et al., 2022)
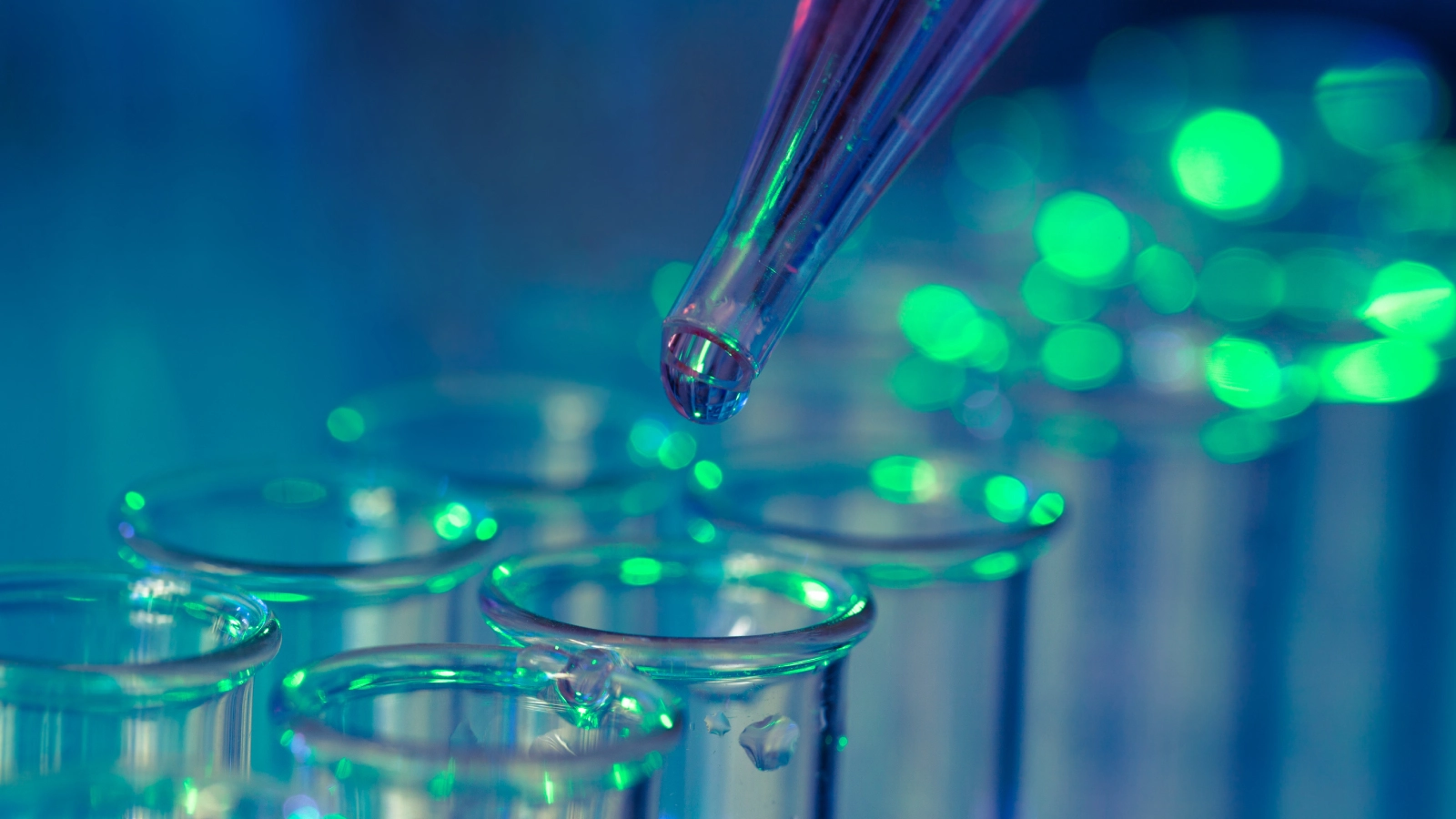
Gene Editing
AI is also playing a role in the development of gene editing technologies, which allow researchers to modify the genetic code of living organisms. One of the most promising gene editing technologies is CRISPR-Cas9, which uses a system of molecular scissors to cut and edit DNA.
In a study published in the journal Cancer Informatics in 2022, researchers used AI to identify new CRISPR-Cas9 targets that could be used to edit the genomes of plants. The use of artificial intelligence (AI) capacity to edit genomes through CRISPR/Cas9 enables modification of gene mutations and molecular simulation.
AI approaches include knowledge discovery approaches, antigen and epitope prediction approaches, and agent-based-model approaches. These methods in combination with CRISPR/Cas9 can be used in vaccine design.
Protein and Gene Network Analysis
AI is also being used to analyze the complex networks of genes and proteins that underlie many diseases. By analyzing these networks, researchers can identify the key genes and proteins that are involved in disease progression and develop new therapies to target those molecules.
In a study published in the journal Aging and Disease in 2020, researchers used AI to analyze the network of genes and proteins involved in Alzheimer's disease. A comprehensive review is focused on the application of AI in the genetic research of AD, including the diagnosis and prognosis of AD based on genetic data, the analysis of genetic variation, gene expression profile, gene-gene interaction in AD, and genetic analysis of AD based on a knowledge base.
Don't overlook the advantages AMD EPYC 9004 offers to your groundbreaking research. Experience EPYC performance for yourself with Exxact servers powered by AMD EPYC 9004.

Navigating the Pitfalls of Applying Machine Learning in Genomics
Despite these promising developments, there are still several challenges to overcome in the field of AI and genomics. One of the biggest challenges is the lack of standardization in genomic data. Different labs and sequencing platforms generate genomic data in different formats, making it difficult to compare and analyze data across different studies.
To address this issue, several initiatives are underway to develop standardized methods for storing and analyzing genomic data. For example, the Global Alliance for Genomics and Health (GA4GH) is a collaborative effort to develop standards for sharing and analyzing genomic data across different platforms and research institutions.
Another challenge is the potential for bias in AI algorithms. Machine learning algorithms are only as good as the data they are trained on, and if the data is biased, the algorithm will be biased as well.
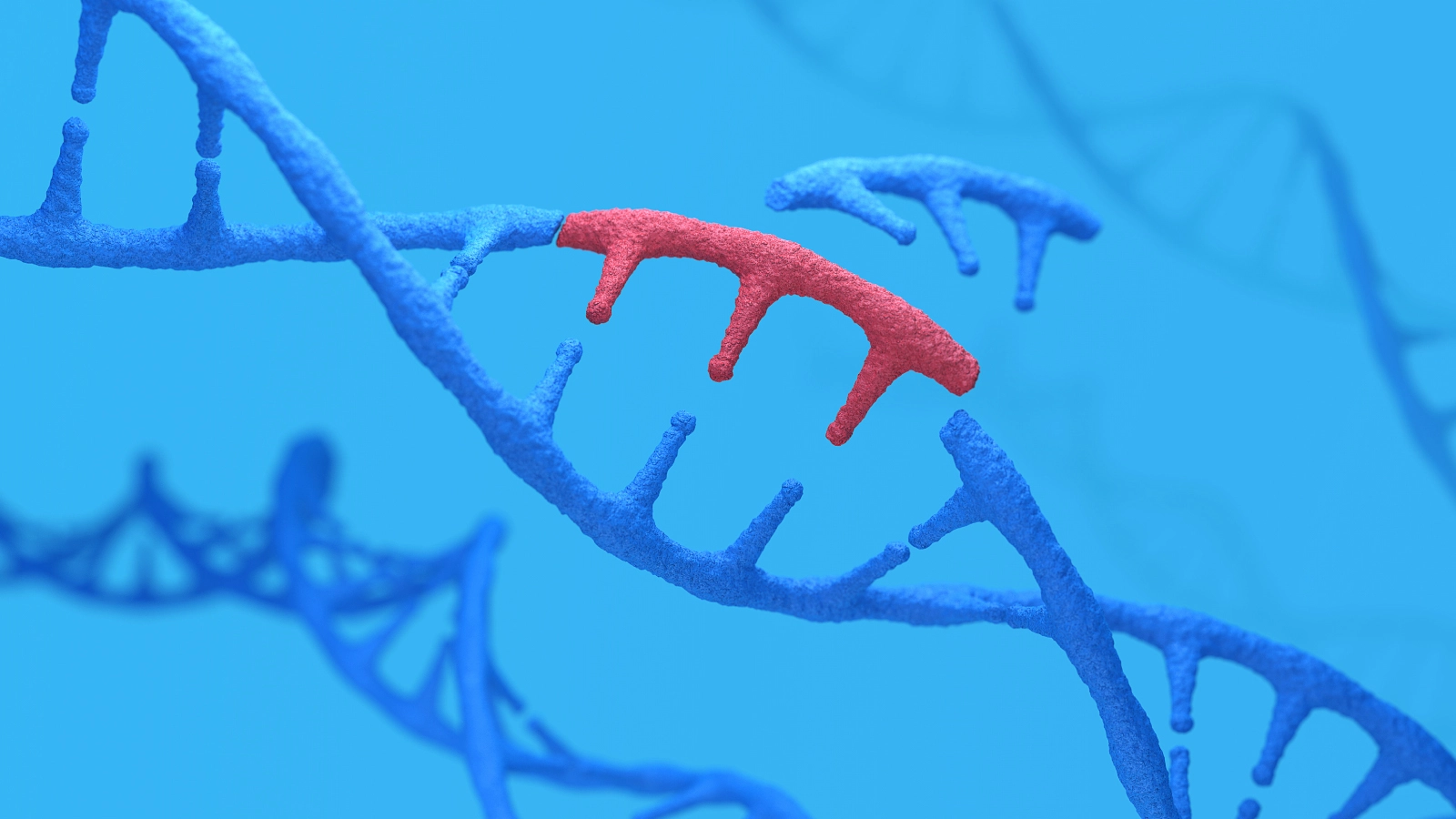
Key Research References
- Artificial Intelligence to guide precision anticancer therapy with multitargeted kinase inhibitors: In this study, researchers proposed a novel graph deep learning technique - CancerOmicsNet. This network integrates multiple heterogeneous data by utilizing a deep graph learning model with sophisticated attention propagation mechanisms to extract highly predictive features from cancer-specific networks. The AI-based system was devised to provide more accurate and robust predictions than data-driven therapeutic discovery using gene signature reversion.
- DeepTorrent: a deep learning-based approach for predicting DNA N4-methylcytosine sites: In this study, researchers proposed a deep learning-based approach, called DeepTorrent, for improved prediction of 4mC sites from DNA sequences. It combines four different feature encoding schemes to encode raw DNA sequences and employs multi-layer convolutional neural networks with an inception module integrated with bidirectional long short-term memory to effectively learn the higher-order feature representations., which could help researchers better understand genetic diseases.
- Artificial Intelligence in drug development: present status and future prospects: At present, the pharmaceutical industry is facing challenges in sustaining its drug development programs because of increased R&D costs and reduced efficiency. In this review, we discuss the major causes of attrition rates in new drug approvals, the possible ways that AI can improve the efficiency of the drug development process, and the collaboration of pharmaceutical industry giants with AI-powered drug discovery firms.
- DeepChrome: deep-learning for predicting gene expression from histone modifications: In this study, researchers developed a unified discriminative framework using a deep convolutional neural network to classify gene expression using histone modification data as input. Their novel framework, called DeepChrome, allows the automatic extraction of complex interactions among important features. This could potentially help researchers better understand gene regulation.
- Cutting-Edge AI Technologies Meet Precision Medicine to Improve Cancer Care: To provide precision medicine for better cancer care, researchers must work on clinical patient data, such as electronic medical records, computerized tomography scans, physiological eval results, biochemistry lab results, digital pathology, and the genetic landscape. To interpret this biodata in cancer genomics, an operational flow based on AI and ML models and medical management platforms with high-performance computing must be set up for precision cancer genomics in clinical practice. This is a review article covering recent developments in these areas.
What is the Future of AI in Genomics?
One of the most significant areas where AI technologies are being utilized is cancer care, where they help in identifying the specific genetic mutations that cause cancer and developing personalized treatments. Precision medicine, which involves using genetic information to tailor medical treatments to individual patients, is another area where AI and machine learning are making a difference.
These technologies are also being used to analyze gene expression patterns and identify disease biomarkers, which can aid in early diagnosis and treatment. The role of AI and machine learning in drug discovery, including the prediction of potential drug targets and the optimization of drug design is becoming more significant every day.
As computers advance and accelerate large computationally heavy workloads, AI and machine learning will continue to revolutionize healthcare research. Cancer care, precision medicine, gene expression analysis, and drug discovery all are made possible with better hardware, and better machine learning models. With further advancements in these technologies, it is likely that their impact on genomics research will soon become groundbreaking scientific marvels.
Combine your drug discovery and genomics workflow with AI to boost your capabilities.
Contact us today to learn more!