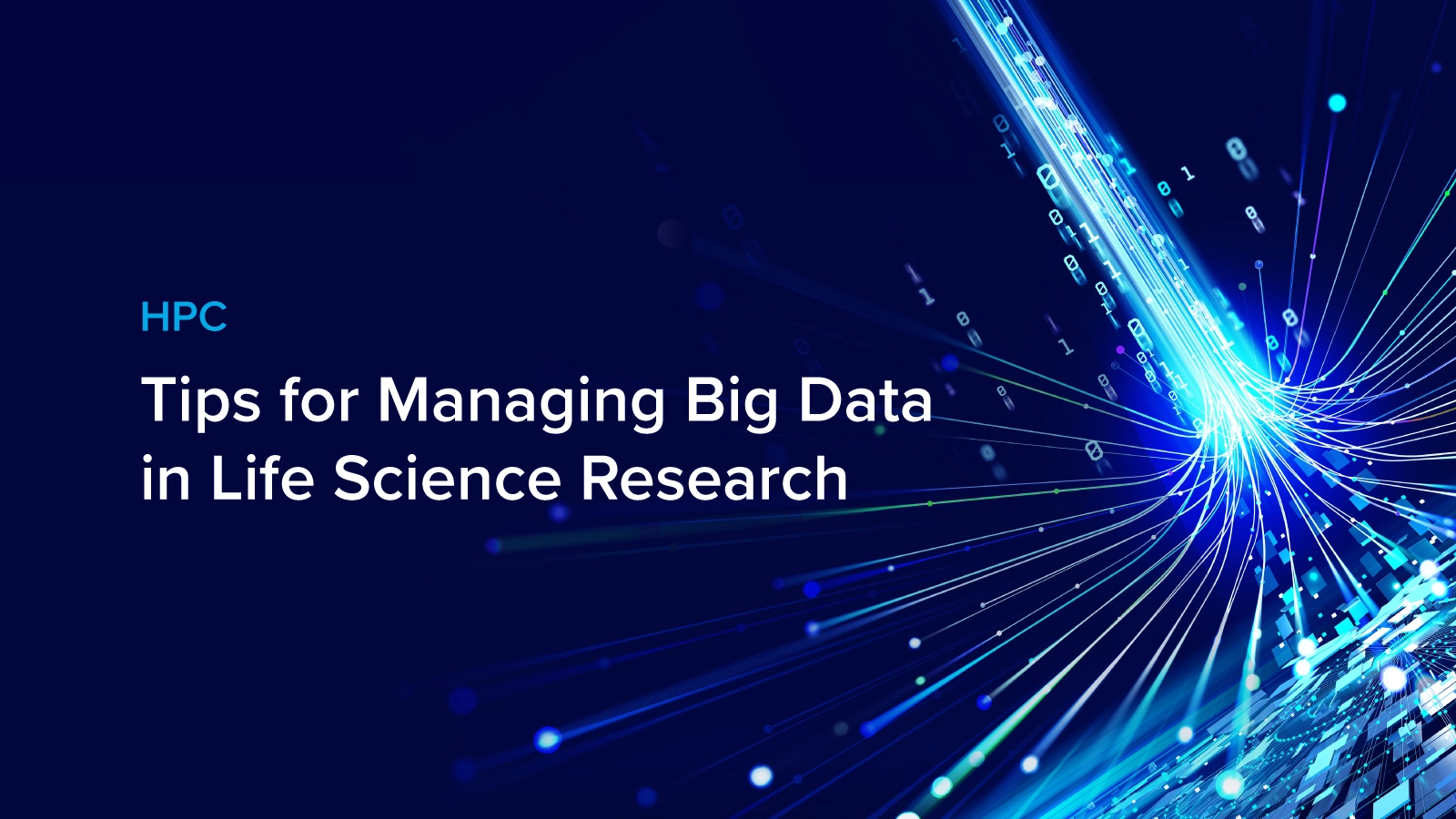
Intro to Big Data and Research
In recent years the amount of data generated has skyrocketed exponentially. The application of big data in life science research has transformed the field, giving rise to new avenues of exploration and revolutionizing our understanding of various diseases. But, as with any significant change, this transformation presents a challenge, requiring us to use advanced tools and techniques to handle the massive volume of data generated.
To drive innovative discoveries and achieve accurate results, it's crucial to manage big data in life science research efficiently. Developing effective strategies and adopting new technologies can drive new innovations faster than ever.
Challenges in managing big data in life science research
The management of big data in life science research is complicated by several factors, including data heterogeneity, data complexity, and data volume.
- Data Heterogeneity: Life science research involves generating data from various sources, such as experiments, literature, and databases. Each source of data may have different formats, structures, and standards, making it challenging to combine and analyze.
- Data complexity: Biological datasets are complex and difficult to process and analyze. For example, genomics data involves a massive amount of information, and analyzing this data requires advanced tools and techniques.
- Data Volume: Life science research generates vast amounts of data that require efficient storage and processing solutions.
Preparing for Data Management
Identifying the data sources is a crucial step in the data management process, as it sets the foundation for the subsequent steps. Researchers need to be aware of the different types of data generated in life science research, including genomics, proteomics, metabolomics, and clinical data, and where this data comes from. Data sources can include experimental equipment, databases, and literature, among others. Understanding this is essential as it helps researchers identify potential gaps in data collection and ensure that they have access to all the necessary data required to address their research questions.
Creating a data management plan is the next step in the process. Outlines your strategy for data management, including data collection, storage, sharing, and analysis. A well-structured plan ensures that data is collected, stored, and analyzed in a consistent and standardized manner while complying with regulatory requirements and funding agency guidelines.
Finally, determine the storage requirements to ensure that data is stored securely and easily accessible. Researchers need to assess the storage capacity required for the data generated and determine the type of storage that best suits their needs:
- Cloud-Base Storage: Cloud storage has no overhead and is easy to get started as you have your own data managing facility but is an ongoing cost, increasing with the amount of data stored. You can also be limited to your data's capabilities.
- Local Storage: Local storage, though having a higher startup cost, can ultimately reduce cost in the long run. Provisioning your own data can be daunting as well but is highly rewarding since you have complete control over adding capacity, upgraded networking, and more with a whole lot of other advanced capabilities you can choose or not choose to enable.
It's also important to have a backup and recovery procedure in place to safeguard against data loss or damage. Having adequate storage and backup procedures in place not only protects valuable research data but also helps researchers to easily retrieve and analyze data, saving time and improving efficiency.
Having storage is essential for any enterprise operation, from deep learning to life science research. Explore Exxact's storage servers for more information!
Storing and organizing data
Choosing the right data storage solution is critical to efficient data management. It involves selecting the appropriate storage technology, such as cloud-based storage or local storage, and ensuring that the storage solution can handle the volume and complexity of the data generated. Additionally, choosing a storage solution that supports collaboration and data sharing can enhance the efficiency of research.
Selecting high-performance hardware to assist with data storage can speed up the reading and writing of data on the storage platform. Constant access of data to hardware accelerators necessitates choosing fast drives. This can seem costly, but overall reduce your TCO based on the time they can save. NVMe SSDs deliver drastically faster read and write speeds to boost productivity and reduce wait times when moving files.
Ensuring data security and backups is critical to prevent data loss and maintain the integrity of the research. This includes implementing security measures, such as encryption and access controls, to protect sensitive data, as well as establishing backup and recovery procedures to ensure that data is not lost in the event of a system failure such as a RAID configuration. Backup data and cold storage (data that doesn’t move often) can be stored in more cost-effective solutions like SATA SSDs or hard drives.
It's also essential to consider data governance and compliance with sensitive data storing requirements which plays a vital role in protecting sensitive patient information and ensuring that data is collected and stored ethically and legally.
Analyzing big data
Analyzing big data requires advanced tools and techniques that can handle the volume and complexity of the data. Some of the most essential tools and techniques used for big data analysis are machine learning, artificial intelligence, and visualization tools and techniques.
The sheer volume of Life Science research and the complexity of these computations necessitate the use of High-Performance Computing. In the recent decade, the implementation of parallel computing with GPUs has greatly changed the landscape of scientific computing, speeding up computations and enabling faster, more reliable discoveries.
Machine learning and AI have revolutionized big data analysis in life science research. These techniques can analyze complex data sets, identify patterns, and make predictions based on the data alone. Machine learning and AI can also improve the accuracy and efficiency of data analysis, allowing researchers to extract more information from their data in less time and providing researchers with new insights into diseases and treatments.
Visualization tools and techniques such as Data Science and Data Analytics are also critical to communicating the results of big data analysis in life science research. These tools can create visual representations of complex data sets with ease, making research data more digestible. Visualization tools can also help researchers identify patterns and trends in the data that might be difficult to discern from raw data alone such as trend lines and correlation.
Within the field of Life Science Research, various applications have various hardware requirements. Exxact can tailor the right HPC research system for the right workload just for you!
Conclusion
In conclusion, managing big data in life science research presents both challenges and opportunities. The vast amount of data generated can provide valuable insights into various diseases and open new avenues for research enabling what was once impossible to become a reality.
Additionally, advanced tools and techniques such as machine learning and artificial intelligence, along with visualization tools and techniques, are essential to analyze big data in life science research accurately. By managing big data effectively, researchers can accelerate scientific discoveries and drive innovations in life science research that can positively impact human health.
Have any Questions?
Exploring your Computing Needs?
Contact Exxact today!
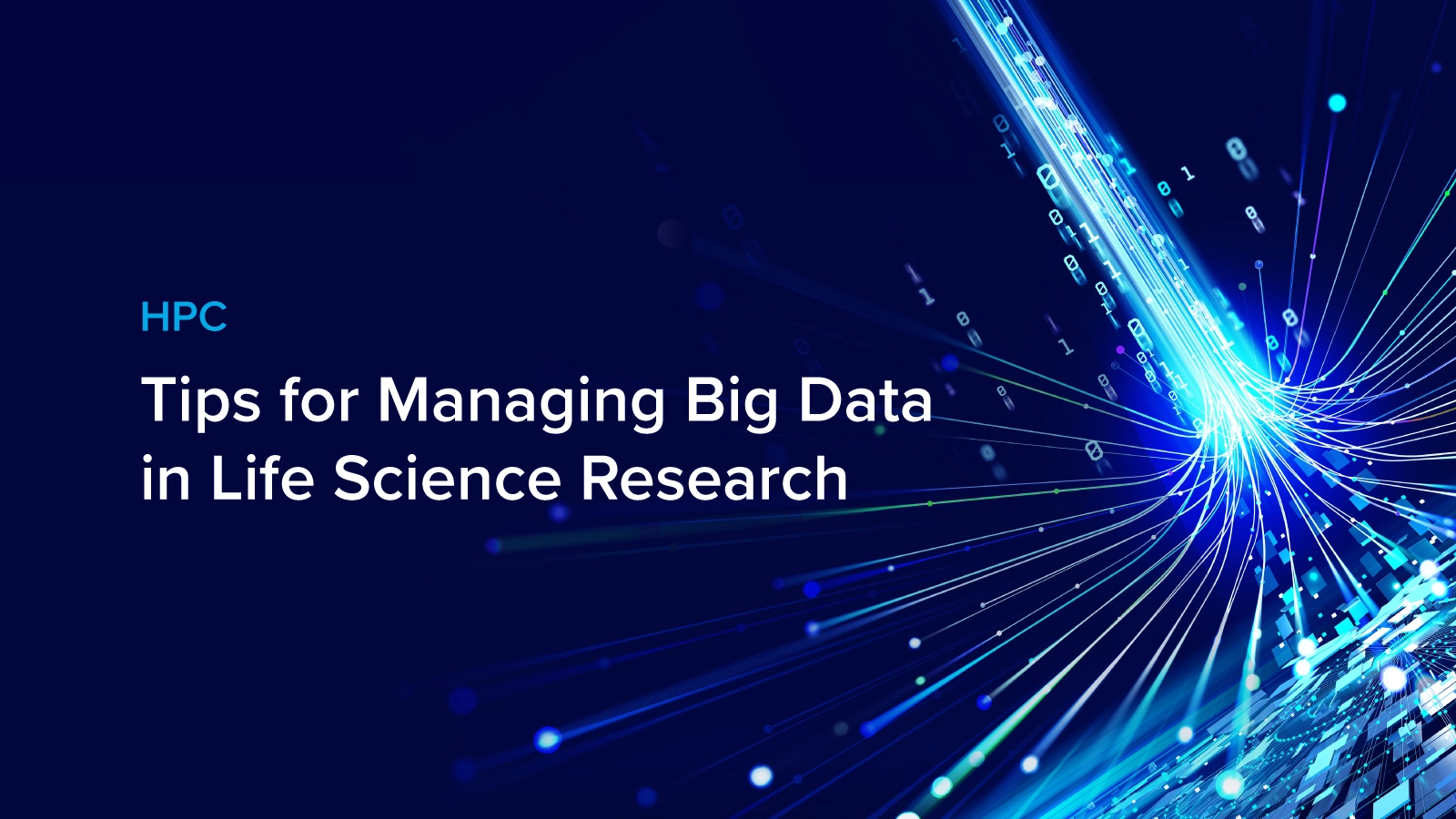
Tips for Managing Big Data in Life Science Research
Intro to Big Data and Research
In recent years the amount of data generated has skyrocketed exponentially. The application of big data in life science research has transformed the field, giving rise to new avenues of exploration and revolutionizing our understanding of various diseases. But, as with any significant change, this transformation presents a challenge, requiring us to use advanced tools and techniques to handle the massive volume of data generated.
To drive innovative discoveries and achieve accurate results, it's crucial to manage big data in life science research efficiently. Developing effective strategies and adopting new technologies can drive new innovations faster than ever.
Challenges in managing big data in life science research
The management of big data in life science research is complicated by several factors, including data heterogeneity, data complexity, and data volume.
- Data Heterogeneity: Life science research involves generating data from various sources, such as experiments, literature, and databases. Each source of data may have different formats, structures, and standards, making it challenging to combine and analyze.
- Data complexity: Biological datasets are complex and difficult to process and analyze. For example, genomics data involves a massive amount of information, and analyzing this data requires advanced tools and techniques.
- Data Volume: Life science research generates vast amounts of data that require efficient storage and processing solutions.
Preparing for Data Management
Identifying the data sources is a crucial step in the data management process, as it sets the foundation for the subsequent steps. Researchers need to be aware of the different types of data generated in life science research, including genomics, proteomics, metabolomics, and clinical data, and where this data comes from. Data sources can include experimental equipment, databases, and literature, among others. Understanding this is essential as it helps researchers identify potential gaps in data collection and ensure that they have access to all the necessary data required to address their research questions.
Creating a data management plan is the next step in the process. Outlines your strategy for data management, including data collection, storage, sharing, and analysis. A well-structured plan ensures that data is collected, stored, and analyzed in a consistent and standardized manner while complying with regulatory requirements and funding agency guidelines.
Finally, determine the storage requirements to ensure that data is stored securely and easily accessible. Researchers need to assess the storage capacity required for the data generated and determine the type of storage that best suits their needs:
- Cloud-Base Storage: Cloud storage has no overhead and is easy to get started as you have your own data managing facility but is an ongoing cost, increasing with the amount of data stored. You can also be limited to your data's capabilities.
- Local Storage: Local storage, though having a higher startup cost, can ultimately reduce cost in the long run. Provisioning your own data can be daunting as well but is highly rewarding since you have complete control over adding capacity, upgraded networking, and more with a whole lot of other advanced capabilities you can choose or not choose to enable.
It's also important to have a backup and recovery procedure in place to safeguard against data loss or damage. Having adequate storage and backup procedures in place not only protects valuable research data but also helps researchers to easily retrieve and analyze data, saving time and improving efficiency.
Having storage is essential for any enterprise operation, from deep learning to life science research. Explore Exxact's storage servers for more information!
Storing and organizing data
Choosing the right data storage solution is critical to efficient data management. It involves selecting the appropriate storage technology, such as cloud-based storage or local storage, and ensuring that the storage solution can handle the volume and complexity of the data generated. Additionally, choosing a storage solution that supports collaboration and data sharing can enhance the efficiency of research.
Selecting high-performance hardware to assist with data storage can speed up the reading and writing of data on the storage platform. Constant access of data to hardware accelerators necessitates choosing fast drives. This can seem costly, but overall reduce your TCO based on the time they can save. NVMe SSDs deliver drastically faster read and write speeds to boost productivity and reduce wait times when moving files.
Ensuring data security and backups is critical to prevent data loss and maintain the integrity of the research. This includes implementing security measures, such as encryption and access controls, to protect sensitive data, as well as establishing backup and recovery procedures to ensure that data is not lost in the event of a system failure such as a RAID configuration. Backup data and cold storage (data that doesn’t move often) can be stored in more cost-effective solutions like SATA SSDs or hard drives.
It's also essential to consider data governance and compliance with sensitive data storing requirements which plays a vital role in protecting sensitive patient information and ensuring that data is collected and stored ethically and legally.
Analyzing big data
Analyzing big data requires advanced tools and techniques that can handle the volume and complexity of the data. Some of the most essential tools and techniques used for big data analysis are machine learning, artificial intelligence, and visualization tools and techniques.
The sheer volume of Life Science research and the complexity of these computations necessitate the use of High-Performance Computing. In the recent decade, the implementation of parallel computing with GPUs has greatly changed the landscape of scientific computing, speeding up computations and enabling faster, more reliable discoveries.
Machine learning and AI have revolutionized big data analysis in life science research. These techniques can analyze complex data sets, identify patterns, and make predictions based on the data alone. Machine learning and AI can also improve the accuracy and efficiency of data analysis, allowing researchers to extract more information from their data in less time and providing researchers with new insights into diseases and treatments.
Visualization tools and techniques such as Data Science and Data Analytics are also critical to communicating the results of big data analysis in life science research. These tools can create visual representations of complex data sets with ease, making research data more digestible. Visualization tools can also help researchers identify patterns and trends in the data that might be difficult to discern from raw data alone such as trend lines and correlation.
Within the field of Life Science Research, various applications have various hardware requirements. Exxact can tailor the right HPC research system for the right workload just for you!
Conclusion
In conclusion, managing big data in life science research presents both challenges and opportunities. The vast amount of data generated can provide valuable insights into various diseases and open new avenues for research enabling what was once impossible to become a reality.
Additionally, advanced tools and techniques such as machine learning and artificial intelligence, along with visualization tools and techniques, are essential to analyze big data in life science research accurately. By managing big data effectively, researchers can accelerate scientific discoveries and drive innovations in life science research that can positively impact human health.
Have any Questions?
Exploring your Computing Needs?
Contact Exxact today!