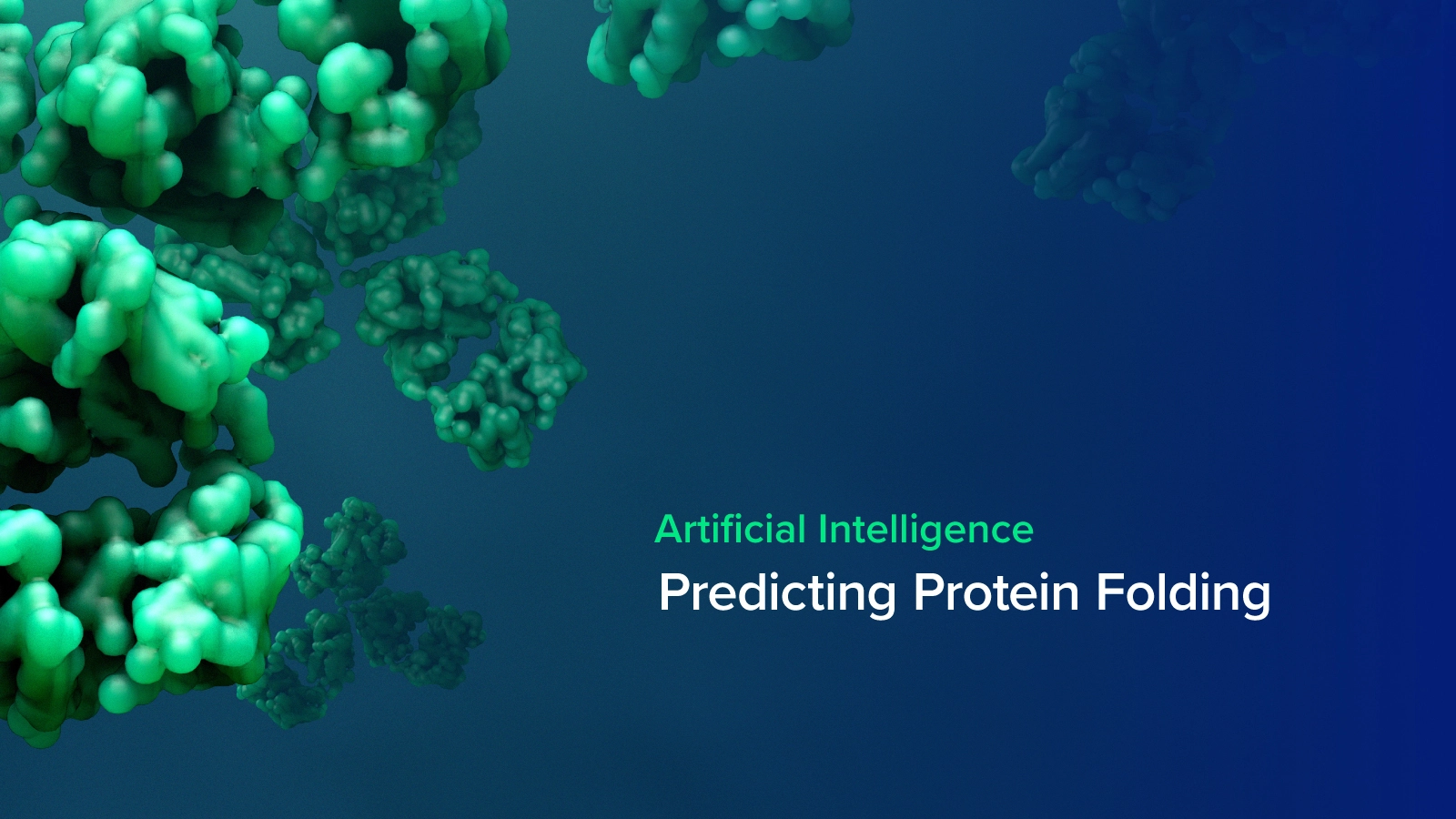
What are Proteins?
Proteins are essential to all life on Earth. Inside every cell of every living organism, proteins are hard at work to carry out critical functions. In the human body, proteins repair damaged tissue, carry oxygen in your blood, digest the food you eat, regulate hormones, and so much more. They make up your hair, nails, skin, bones, and muscles, proteins are part of what makes you who you are. Aside from proteins in humans, there are hundreds of millions of proteins yet to be mapped and more to be discovered, like plant proteins, organism proteins, and even malicious proteins.
Protein Structure
A protein is made up of one or more linear chains of amino acids. There are 20 different amino acids that can be combined, and the sequence defines each protein's specific function and determines its 3D structure. Interactions between the amino acids make the protein fold as it finds its shape out of almost limitless possibilities.
The ability to predict the 3D structure unlocks a greater understanding of what the protein does and how it works. By advancing scientific discoveries, the future of protein folding could assist in developing drugs to treat diseases and finding enzymes to break down industrial waste.
Interested in working with AlphaFold?
Learn more about Exxact AI-Assisted Life Science Solutions
Determining Protein Structures
With over 100 million know proteins and more to be discovered, researchers only know the 3D structure of a small tiny fraction of them. For many years, researchers have experimented with various techniques to examine, determine, and reconstruct protein structures.
- Nuclear magnetic resonance spectroscopy relies on a powerful magnet and radio frequency signals to determine the distance between adjacent nuclei, which in turn can be used to determine the overall structure of the protein.
- X-ray crystallography relies on measuring the angles and intensities of diffracted X-ray beams from a crystalline structure, such as protein.
- Cryogenic electron microscopy (cryo-EM), involves flash-freezing molecules in liquid nitrogen and bombarding them with electrons to capture images with a special camera.
Even with advancements in these methods, scientists still rely on expensive and extensive trial and error, taking years to determine just one structure, and requiring the use of multi-million-dollar specialized equipment. Not to mention, some proteins are too fragile to be modeled using physical means like freezing or crystallizing.
Predicting Protein Fold
As an alternative to the pricey and time consuming methods of determining a protein’s 3D structure, researchers have been working hard to computationally predict a protein’s shape based solely on its one-dimensional amino acid sequence. The number of ways a protein could theoretically fold before settling into its final 3D structure is astronomical, some 10300 ways!
In search of a solution to the 50-year-old “protein folding problem,” Critical Assessment of protein Structure Prediction (CASP) was founded in 1994 as a biennial competition to catalyze research and measure progress on the newest methods for improving the accuracy of predictions. Teams are given a selection of protein's amino acid sequences for proteins. These proteins have had their exact 3D shape mapped but have not yet been released into the public domain. The teams will submit their best predictions and be given a score for the accuracy against the subsequently revealed structures.
Using AI to Solve Things
In 2016, Alphabet Inc. subsidiary DeepMind created an artificial intelligence system called AlphaFold to tackle the protein folding problem. DeepMind’s mission has been to test the limits of AI and task it to learn astronomically complex problems. DeepMind made a pioneering breakthrough by training a Deep Q-Network algorithm to play classic games from Atari to playing the age-old, complexly nuanced game of Chess. With a game with over 10170 possible board configurations DeepMind's AlphaGo was an ultimate display of the capabilities of self-training deep neural network AI, defeating the top player in the world at a game it learned in less than a year.
DeepMind loved training its AI on games since it gave numerical value for winning or losing which allowed AI training to advance quickly. CEO Demis Hassabis's vision for developing AI was not to win games but to ultimately fuel the next generation of scientific discovery; “From the beginning, this is what we set out to do: to make breakthroughs in AI, test that on games, [and] apply that to real-world problems, to see if we can accelerate scientific breakthroughs and use those to benefit humanity.” If computers can learn to play games as complex as Go and Chess, surely they can fold some proteins, right?
DeepMind Takes on Protein Folding
In CASP13 (2018), for the first time, there was an effective application of artificial intelligence. CASP is graded on Global Distance Test (GDT) to measure the accuracy of predictions ranging from 0 to 100, with 90 GDT considered competitively accurate against methods like cryo-EM and X-ray crystallography. DeepMind's AlphaFold was placed first with a median GDT of 61.4, a record high for CASP! It was able to approximately predict the correct shapes for many of the proteins, but the details and exactly where each atom sits were quite as accurate as they hoped. The shapes emulated the ground truth shapes but were atom positions were skewed to try represent the real protein. DeepMind went back to the drawing table.
For CASP14 (2020), DeepMind reentered the competition with their revised solution AlphaFold 2 to continue its AI approach. By switching from a convolutional neural network, AlphaFold 2 used a transformer attention-based neural network and trained on 170,000 known protein structures from the Protein Data Bank. Right off the bat AlphaFold 2 delivered atomic accuracy for a very difficult SARS-CoV-2 protein called ORF8 in the free-modeling category. AlphaFold achieved a minimum score of 87.0 GDT on the most difficult protein but was able to achieve a median of 92.4 GDT with some proteins predicted at a 99% match. With a score of over 90%, many remarked that the protein folding problem had been solved. This is one of the biggest advancements in structural biology and artificial intelligence in recent history.
DeepMind and the European Molecular Biology Laboratory (EMBL) currently host a public database of about 1 million protein structures on the AlphaFold website. In addition, AlphaFold has folded the entire human proteome and key organisms with great accuracy. DeepMind plans to help fold, document, and list 100 million known proteins this year to the database. Not only has AlphaFold proven that AI could accurately predict the shape of a protein with high accuracy, but it can do so at scale, compared to years using prior methods. AlphaFold2 is run on the Google Cloud with enterprise hardware; if a protein is not listed in the extensive database, users can submit new proteins to fold on the cloud. RoseTTAFold offers a similar protein folding solution but requires significantly less computing power and can be executed using a modern desktop. Although RoseTTAFold is not as accurate as AlphaFold, its easy access and low computing allow researchers to fold proteins right there in their lab.
The era of digital biology is next for DeepMind. CEO Demis Hassabis describes Biology as an information processing unit at its most fundamental level, a perfect environment for AI to advance scientific discovery. There’s a lot more work to be done but unlocking the shapes of these building blocks could help scientists better understand the natural world and perhaps expand the knowledge of life itself.
Have any questions?
Contact Exxact Today
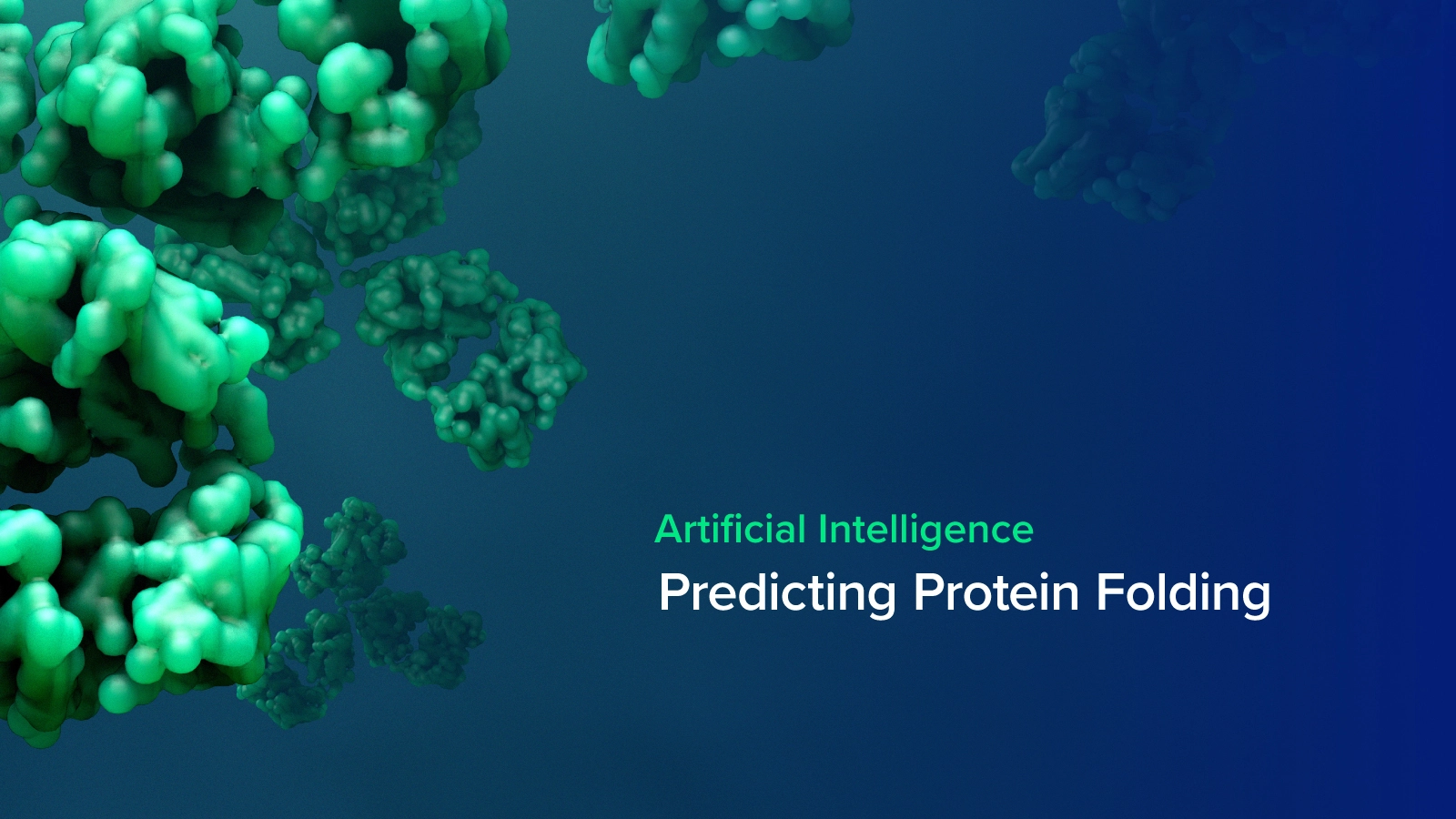
Using Artificial Intelligence to Predict Protein Folding
What are Proteins?
Proteins are essential to all life on Earth. Inside every cell of every living organism, proteins are hard at work to carry out critical functions. In the human body, proteins repair damaged tissue, carry oxygen in your blood, digest the food you eat, regulate hormones, and so much more. They make up your hair, nails, skin, bones, and muscles, proteins are part of what makes you who you are. Aside from proteins in humans, there are hundreds of millions of proteins yet to be mapped and more to be discovered, like plant proteins, organism proteins, and even malicious proteins.
Protein Structure
A protein is made up of one or more linear chains of amino acids. There are 20 different amino acids that can be combined, and the sequence defines each protein's specific function and determines its 3D structure. Interactions between the amino acids make the protein fold as it finds its shape out of almost limitless possibilities.
The ability to predict the 3D structure unlocks a greater understanding of what the protein does and how it works. By advancing scientific discoveries, the future of protein folding could assist in developing drugs to treat diseases and finding enzymes to break down industrial waste.
Interested in working with AlphaFold?
Learn more about Exxact AI-Assisted Life Science Solutions
Determining Protein Structures
With over 100 million know proteins and more to be discovered, researchers only know the 3D structure of a small tiny fraction of them. For many years, researchers have experimented with various techniques to examine, determine, and reconstruct protein structures.
- Nuclear magnetic resonance spectroscopy relies on a powerful magnet and radio frequency signals to determine the distance between adjacent nuclei, which in turn can be used to determine the overall structure of the protein.
- X-ray crystallography relies on measuring the angles and intensities of diffracted X-ray beams from a crystalline structure, such as protein.
- Cryogenic electron microscopy (cryo-EM), involves flash-freezing molecules in liquid nitrogen and bombarding them with electrons to capture images with a special camera.
Even with advancements in these methods, scientists still rely on expensive and extensive trial and error, taking years to determine just one structure, and requiring the use of multi-million-dollar specialized equipment. Not to mention, some proteins are too fragile to be modeled using physical means like freezing or crystallizing.
Predicting Protein Fold
As an alternative to the pricey and time consuming methods of determining a protein’s 3D structure, researchers have been working hard to computationally predict a protein’s shape based solely on its one-dimensional amino acid sequence. The number of ways a protein could theoretically fold before settling into its final 3D structure is astronomical, some 10300 ways!
In search of a solution to the 50-year-old “protein folding problem,” Critical Assessment of protein Structure Prediction (CASP) was founded in 1994 as a biennial competition to catalyze research and measure progress on the newest methods for improving the accuracy of predictions. Teams are given a selection of protein's amino acid sequences for proteins. These proteins have had their exact 3D shape mapped but have not yet been released into the public domain. The teams will submit their best predictions and be given a score for the accuracy against the subsequently revealed structures.
Using AI to Solve Things
In 2016, Alphabet Inc. subsidiary DeepMind created an artificial intelligence system called AlphaFold to tackle the protein folding problem. DeepMind’s mission has been to test the limits of AI and task it to learn astronomically complex problems. DeepMind made a pioneering breakthrough by training a Deep Q-Network algorithm to play classic games from Atari to playing the age-old, complexly nuanced game of Chess. With a game with over 10170 possible board configurations DeepMind's AlphaGo was an ultimate display of the capabilities of self-training deep neural network AI, defeating the top player in the world at a game it learned in less than a year.
DeepMind loved training its AI on games since it gave numerical value for winning or losing which allowed AI training to advance quickly. CEO Demis Hassabis's vision for developing AI was not to win games but to ultimately fuel the next generation of scientific discovery; “From the beginning, this is what we set out to do: to make breakthroughs in AI, test that on games, [and] apply that to real-world problems, to see if we can accelerate scientific breakthroughs and use those to benefit humanity.” If computers can learn to play games as complex as Go and Chess, surely they can fold some proteins, right?
DeepMind Takes on Protein Folding
In CASP13 (2018), for the first time, there was an effective application of artificial intelligence. CASP is graded on Global Distance Test (GDT) to measure the accuracy of predictions ranging from 0 to 100, with 90 GDT considered competitively accurate against methods like cryo-EM and X-ray crystallography. DeepMind's AlphaFold was placed first with a median GDT of 61.4, a record high for CASP! It was able to approximately predict the correct shapes for many of the proteins, but the details and exactly where each atom sits were quite as accurate as they hoped. The shapes emulated the ground truth shapes but were atom positions were skewed to try represent the real protein. DeepMind went back to the drawing table.
For CASP14 (2020), DeepMind reentered the competition with their revised solution AlphaFold 2 to continue its AI approach. By switching from a convolutional neural network, AlphaFold 2 used a transformer attention-based neural network and trained on 170,000 known protein structures from the Protein Data Bank. Right off the bat AlphaFold 2 delivered atomic accuracy for a very difficult SARS-CoV-2 protein called ORF8 in the free-modeling category. AlphaFold achieved a minimum score of 87.0 GDT on the most difficult protein but was able to achieve a median of 92.4 GDT with some proteins predicted at a 99% match. With a score of over 90%, many remarked that the protein folding problem had been solved. This is one of the biggest advancements in structural biology and artificial intelligence in recent history.
DeepMind and the European Molecular Biology Laboratory (EMBL) currently host a public database of about 1 million protein structures on the AlphaFold website. In addition, AlphaFold has folded the entire human proteome and key organisms with great accuracy. DeepMind plans to help fold, document, and list 100 million known proteins this year to the database. Not only has AlphaFold proven that AI could accurately predict the shape of a protein with high accuracy, but it can do so at scale, compared to years using prior methods. AlphaFold2 is run on the Google Cloud with enterprise hardware; if a protein is not listed in the extensive database, users can submit new proteins to fold on the cloud. RoseTTAFold offers a similar protein folding solution but requires significantly less computing power and can be executed using a modern desktop. Although RoseTTAFold is not as accurate as AlphaFold, its easy access and low computing allow researchers to fold proteins right there in their lab.
The era of digital biology is next for DeepMind. CEO Demis Hassabis describes Biology as an information processing unit at its most fundamental level, a perfect environment for AI to advance scientific discovery. There’s a lot more work to be done but unlocking the shapes of these building blocks could help scientists better understand the natural world and perhaps expand the knowledge of life itself.
Have any questions?
Contact Exxact Today