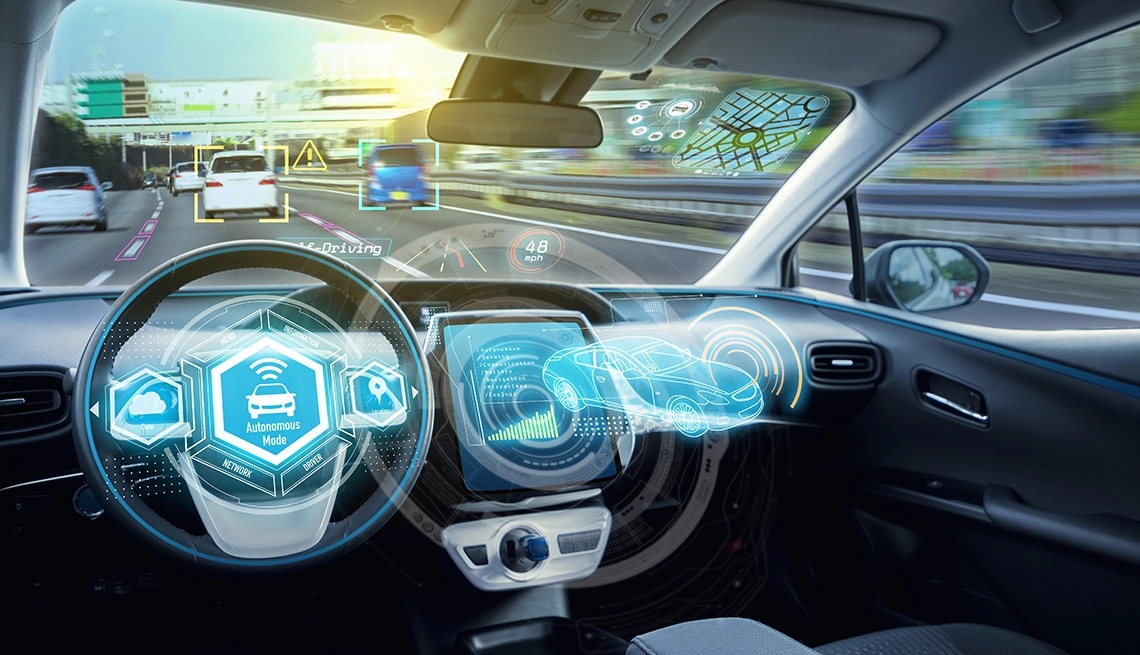
The Acceleration of Autonomous Vehicle Applications due to COVID-19
Hint: It’s not focused on personal transportation
Autonomous vehicles have long been a mainstay of both outlandish fiction and legitimate research, in some interpretations predating the invention of the car itself. By some accounts, full self-driving capabilities would be a major boon in terms of safety and promises to narrow wealth-based gaps in good access to transportation. Perhaps the impact could be as significant as the adoption of safety bicycles, which expanded the distance one could travel by 3 to 4 times or more, without incurring the costs of owning a horse (or car) and the facilities to care for them.
A Common Perspective on Autonomous Driving
The most visible application of autonomous vehicles, and probably what most of us think of when we hear the term ‘autonomous vehicles,’ is the idea of self-driving cars, aka autonomous vehicles for personal transport. But personal transport may be the worst application for autonomous vehicles in terms of safety and attainability.
Self-driving cars may very well be remembered as the 2010’s jet pack: a much-hyped vaporware that’s never ready for widespread consumer use. At this point it’s essentially a tradition that every year (since 2016) Elon Musk predicts the imminent arrival of full self-driving capabilities in Tesla vehicles—any day now. As 2020 approaches its end, there’s no better time to reflect on missed expectations and disappointments, but let’s not forget to look for silver linings.
Jet pack comic cover in the public domain from Wikipedia
The Pandemic Accelerated Some Economic Trends
The COVID-19 pandemic has in many ways accelerated economic trends that have been predicted to occur anyway. Some of the accelerated shifts are troubling, like widening wealth gaps and a K-shaped recovery from recession. While other shifts, like increased support for remote working, are positive (though a widespread shift to work-from-home has also yielded nefarious downsides). This article posits that the arrival of full self-driving for private transportation is not one of those accelerated trends. In fact, several large players have taken 2020’s economic turmoil as an opportunity to divest from full self-driving research and development.
The stress that 2020 has placed on the logistics system and a boom in delivery services fueled by remote work and stay-at-home orders have revealed more promising areas for autonomous vehicles. There are a number of reasons why self-driving for personal transportation is not the best application to focus on for autonomous vehicle development, at least when considered in context next to the (relatively) low-hanging fruit of cargo transport and last-mile delivery. As we’ll argue below, the near-term future of autonomous vehicles will be more of an automated local courier service rather than a robo-taxi fleet.
Why You Shouldn’t Expect Your Personal FSD Vehicle Any Time Soon
#1 Driving is Dangerous
Driving is one of the most dangerous activities undertaken on a regular basis by large numbers of people. In the US, motor vehicle accidents are either the first or second leading cause of death by injury for all adult age groups according to the CDC, and injurious deaths are currently almost double the number of fatalities due to heart disease and cancer combined.
Estimates vary, but if you drive you probably have around a 1% chance of meeting your end due to a vehicle crash according to the National Safety Council. Minor accidents are even more common, and average drivers can expect to be involved in several over their lifetime.
Drivers can be dangerous to pedestrians and cyclists as well, and unfortunately that’s the sort of edge case that can confuse deep learning algorithms, if not trained for specifically. Even if a vision model was trained with a dataset with tens of thousands of examples of individuals riding upright safety bikes, a recumbent bike or adaptive trike may not be recognized as such, causing unpredictable problems.
Driving safety is a double-edge argument in justifying the development of self-driving cars. Self-driving could and should be safer than human drivers, and that is the number one reason why we might want widespread deployment of self-driving vehicles in the first place. Nonetheless, the intrinsic risks associated with big chunks of metal hurtling through space at speed couples high-stakes with a difficult problem domain.
As far as personal transportation is concerned, the first applications you should expect to see of self-driving “cars” will hardly fit our expectations. We see this already in dedicated vehicles like the miniature delivery van from Nuro.ai or the short-lived WePod trialed in the Netherlands. It just doesn’t make sense to retrofit a highway vehicle for slow, short, city transport on known routes, the most likely application area for self-driving to take hold.
A dedicated self-driving vehicle. Photo by Pixabay user falco (Pixabay license).
#2 Self-Driving is Hard
Go back a few years and there was nothing but optimism about the inevitable rise of self-driving vehicles. Driven in large part by early successes in deep learning for computer vision, it seemed like only a matter of time and training before driving yourself to work or to the shops would be a thing of the past. This wasn’t the only problem we expected deep learning to solve; throw a deep enough pile of artificial neurons at a problem, the thinking went, and your problem disappears (after a lucky hyperparameter search).
OpenAI even released their Universe project in 2016 with the promise of turning any computer task you can run in a Docker container into a reinforcement learning environment, the implication being that the sort of end-to-end intelligence necessary to solve a wide range of tasks with minimal experience was just around the corner.
Training is Important for Self-Driving Cars
Since those early days of optimism way back in the mid-2010s, we’ve learned a lot about the importance of good training data and the sheer difficulty of many problems for neural network models. Deep learning doesn’t do well with rare edge cases, is vulnerable to adversarial attacks and, in general, deep learning decisions remain inscrutable as they can’t explain themselves. Melanie Mitchell, a complexity researcher at the Santa Fe Institute and Portland State University and author of Artificial Intelligence: A Guide for Thinking Humans, posits that true full self-driving at a level comparable to humans essentially requires solving general intelligence. While automated transportation systems operating in controlled, known environments like largely static routes in urban/suburban areas are certainly possible with today’s technology, it’s unlikely that consumers will be able to buy a vehicle capable of a hands-free coast-to-coast road trip in unknown conditions anytime soon.
Deep Learning in Self-Driving Cars
Self-driving cars are notoriously bad at recognizing situations they haven’t seen before, and the deep learning models at the heart of their computer vision systems can fail in surprising ways. One of the first fatalities directly attributable to self-driving technology came from Tesla’s Autopilot in 2016. In the accident, Autopilot mistook the bright side of a semi-trailer as sky, colliding with the underside of the trailer at a speed sufficient to kill the driver.
Interested in a deep learning solution?
Learn more about Exxact AI workstations starting at $3,700
The fatal 2016 Autopilot failure and others like it emphasize a particular risk of self-driving and advanced driver assistance systems when drivers become complacent, but they’re not the most notorious examples of self-driving crashes. The 2018 fatality of Elaine Herzberg at the wheels of Uber’s self-driving Volvo XC90 was arguably due to a lax safety culture, as emergency braking was disabled and the supervising driver was allegedly watching videos on their mobile phone. These failures are not comprehensive, but they are noteworthy as stemming from situations that were created by the semi/autonomous driving features themselves.
Adversarial Attacks in Vision Models
Another avenue for failure is adversarial attacks. In 2017 researchers demonstrated that they could cause a computer vision model to mistake stop signs for speed limit signs with only a few pieces of tape. These attacks may be rare in the wild and quickly patched (and likely prosecuted) when found, but they certainly point to the brittle fragility of deep learning models. That’s not a great mix with the high stakes of automobile travel. One can bet that if self-driving vehicles become commonplace, human drivers will quickly discover behavioral adversarial attacks against self-driving systems.
Ultimately, where a few years ago several self-driving startups had high hopes for end-to-end learning, i.e. formulating driving as a reinforcement learning problem and training driving agents like you might teach a dog new tricks. While companies like Wayve and Comma are ostensibly still following this strategy, most larger players like Tesla, GM, Waymo, and others are focusing on a hybrid approach.
Are We Witnessing a Shift in Self-Driving Cars?
Thanks to the vulnerability of conventional deep learning models to uncertainty and risk (to say nothing of deliberate adversarial perturbations), production self-driving systems are more likely to weave together deep learning for computer vision and sensor representation fusion with more conventional hand-coded automation. In other words, self-driving is starting to look a lot more like driver assistance functionality, which raises another challenge any self-driving developer, investor, or enthusiast would be remiss to ignore.
#3 The Bar for Self-Driving Cars is a Moving Target
Driving is one of the most dangerous activities that many people partake in on a regular basis. This offers both opportunities and challenges for self-driving vehicles. On the one hand, it means there’s room to do better with autonomous vehicles: software does not get tired, bored, or distracted like humans do. On the other hand, it’s indicative of a genuinely difficult problem domain.
What’s more is that any improvement in safety developed for self-driving is almost universally accompanied by associated improvement in driver assistance capabilities. It’s hard to beat the combination of the robotic reflexes and unwavering attention of software with the flexible problem solving and general adaptability of humans.
It’s unlikely that self-driving vehicles will be approved for widespread use if they don’t significantly (statistically and in terms of effect size) improve on the safety of human drivers. However, despite the high yearly cost of driving in terms of fatalities and injuries, individual human drivers are already pretty safe drivers considering the distances travelled, and driver assistance functionality does seem to be decreasing insurance claims when in use despite some criticisms of drivers being overly reliant on features like emergency braking, automatic blind spot monitoring, and lane keeping assistance. If human drivers keep getting safer, albeit with some automated safety assistance, self-driving cars will have a harder time overcoming the threshold.
Companies Involved in Self-Driving Cars
Company | Status | Sensors |
Waymo | Consistently considered a leader in the AV space. | “Laser Bear Honeycomb” lidar, cameras, radar |
Tesla | Efforts led by Andrej Karpathy focusing on computer vision | Radar, cameras, ultrasonic sensors. Notably lacking lidar |
Uber ATG | Sold to Aurora | “FirstLight” lidar, cameras, radar |
Wayve | Actively pursuing end-to-end autonomous driving | Cameras |
Argo | Backed by Ford | Lidar, cameras, radar |
Comma | Comma Two hooks into onboard driver assistance features. | Cameras (including driver monitoring), onboard sensors. Runs on hardware essentially equivalent to a mobile phone. |
Selected players in the self-driving field. In addition to the sensors listed, most self-driving systems use GPS and some use pre-defined high-definition maps.
Why Last Mile Delivery?
Here we’ll focus on the tricky last mile of transport and delivery. This domain includes the type of journey more suited to a bicycle courier than to a truck driver. Some estimates predict that last-mile deliveries will be performed by robots 85% of the time by 2025.
#1 ‘Sidewalk’ Delivery is an Intrinsically Safer Domain
A two-ton automobile traveling at 75 mph has a kinetic energy of a little more than 1 MegaJoule, or about as much energy as half a pound of TNT. A 100 pound robot traveling at a moderate cycling speed of 12 mph has about 678 Joules of kinetic energy, and an injury potential similar to that of a slow cyclist. On sidewalks, where speeds would normally be limited to a brisk walking pace, the potential for injury is even less. It’s also not particularly dangerous (though it will be annoying) for a small delivery robot that gets confused to just stop or pull to the side of the sidewalk to await instructions.
The passengers are certainly lower-stakes as well. If an autonomous delivery robot drives your burrito off a cliff, no big deal, the order can easily be replaced and your life goes on, albeit with dinner a bit delayed. The same can’t be said for a self-driving car suffering catastrophic failure while taking you to the drive-thru.
#2 Delivery Can Be an Easier Problem
The stakes are lower for last-mile delivery relative to full self-driving, but the problem may also be somewhat easier to solve. The speeds are slower (making reaction times less important), and routes are likely to be well-known. You could even imagine a delivery vehicle designed for deriving maximum empathy from bystanders that could issue a pitiable cry for help to solicit a rescue if it got stuck in a pothole.
Those factors and others should make it easier to develop a system that works “well enough.” When things go wrong, and they inevitably will, it’s easier to rescue a lost robot because when making local deliveries they are never out of range of remote control help. The most effective way is probably going to be to have a team of teleoperation supervisors ready to take control at a moment’s notice, making for a hybrid semi-autonomous last-mile delivery system.
#3 Last-Mile Delivery is Well-Suited to Human-in-the-Loop Deployment
Given the complexity and unpredictability of the real world, it’s inevitable that surprises will occur. When they do, a broken robot or lost meal is a lot more manageable downside than death and dismemberment. That being said, last-mile delivery is also more amenable than full self-driving cars human-in-the-loop interventions when things go wrong.
As mentioned in the section on self-driving cars, a human driver with supervisory duties over an autonomous automobile tends to become bored, complacent, and over-reliant on semi-autonomous vehicles. A single human teleoperator would have plenty to keep them busy, and can look after a fleet of numerous delivery robots. That’s probably why Postmates contracted teleoperation specialists Phantom Auto for their expertise, and DoorDash acquired remote operation startup Scotty Labs.
A Hybrid Approach is More Than Likely The Future
A hybrid approach to autonomous vehicles may feel like it falls short of the myriad examples we’ve come to expect from science fiction and comic books, but it’s a pragmatic solution to a practical problem. Thanks to boredom and complacency, self-driving cars that only occasionally require human intervention are probably more dangerous than both fully autonomous (level five) or semi-autonomous vehicles that require correction more often. Delivery robots that require fewer interventions just means that remote operators can handle supervision of more vehicles.
Companies Involved in Autonomous Delivery
Company | Status | The Robot | Sensors |
Starship | In operation in several US and UK locations. | A good example of a six-wheeled robot in the cooler-sized class | Cameras, unspecified edge sensors (perhaps ultrasonic) |
Postmates | Acquired by Uber | “Serve,” about the size of a drinks cooler on wheels” can carry ~50 pounds, with human-in-the-loop teleoperation. | Lidar, sonar, cameras |
Amazon | Acquired Dispatch in 2019 | “Scout” is a small six-wheeled robot sporting the Amazon Prime smile logo. | Cameras |
Nuro | Recently completed $500 million funding round, running trials in Houston, Texas and Scottsdale, Arizona. | “R2,” a small autonomous van with capacity for an entire week’s groceries for several recipients at once. | Cameras, radar, lidar |
Academy of Robotics | Trialling services in London and Surrey | The “Kar-Go” delivery vehicle, smaller than an ATV, is probably the coolest-looking robot on the list. | Uses Bayesian Simultaneous Localization and Mapping (SLAM) algorithms to fuse vision, lidar, and inertial sensors. |
UDI | Chinese delivery startup that stepped into the limelight during spring lockdowns. | Boxy, autonomous van similar to Nuro’s R2. | Cameras, lidar |
Noteworthy players in the autonomous last-mile delivery space.
Challenges to Robotic Delivery
As we outlined above, robotic delivery is in many ways an easier problem with lower stakes than self-driving cars for personal transportation. That doesn’t mean the task comes without its own challenges, the first of which will fit nicely into the dystopian worldview you’ve come to know and love.
#1 Human Delivery Workers are Cheap
How do gig economy services employing (excuse me, contracting) a ragtag collection of semi-professional drivers and couriers compete with established players employing professionals? In some cases this may be a matter of servicing the long tail of under-served niches, but the main advantage seems to be cheapening the cost of labor. Rideshare and delivery providers like Uber and Lyft benefit from offloading many of the costs of doing business (vehicle maintenance, payroll taxes, health insurance etc.) to their workers. They’re willing to lobby heavily to protect the status quo, recently spending a combined $205 million on California’s Prop 22 to do just that.
Depending on who produces the estimates, Uber and Lyft drivers make somewhere between $5.64 and $27.58 an hour, with the study producing the latter number funded by Lyft. Estimates for gig work delivery couriers tend to fall on the lower end of that spectrum as well.
No matter which way you slice it, cheap human labor is powering the gig economy, but that could change if regulations, collective action, or a tightening labor market push wages higher. If and when that happens the opportunity for friendly robotic replacements will grow, so long as they are accepted by the humans they rub elbows with.
#2 Critics Decry the Takeover of Public Spaces
hitchBOT was a stationary robot that interacted with humans with basic conversational skills and blinking lights, ultimately using its charm to hitchhike through Canada and Europe. Then hitchBOT tried its luck on a route from San Francisco to Boston, crossing all the way to Philadelphia before being destroyed. Pennsylvania may have legally classified delivery robots as pedestrians, but that doesn’t mean the robots themselves aren’t vulnerable.
Photo of hitchBOT and friends CC BY flickr user archieflickers.
The expected proliferation of delivery robots using sidewalks is expected by some to be a major source of conflict going forward, stressing public infrastructure that is already given second-class status in US cities. Robotic delivery services with slightly larger vehicles like China’s UDI, Nuro in the United States, or that of Academy of Robotics in England, may fare better integrating into motorway traffic.
Given the public response to undocked scooter and bike share vehicles invading public sidewalks, providers of delivery robot services will have to be careful. Robots sharing human spaces need to elicit empathy from those around them and act respectfully in crowded areas.
The Pandemic Has Affected the Self-Driving Industry in Many Ways
With overall consumer excitement for self-driving cars in general seriously waning by 2020, a pandemic-related recession further dampened enthusiasm in the industry, already at risk due to wavering consumer sentiment and a recognition that solving the problem is harder than anticipated. But the explosion of demand for contactless delivery services has had the opposite effect on the appetite for autonomous local and last-mile delivery startups, which is readily apparent in the recent $500 million dollar investment round completed by robotic delivery startup Nuro.
Photo by Bill Nino on Unsplash (Unsplash license)
There may be no better example of where the industry is headed than the example of Uber. In addition to taking advantage of the recession to issue thousands of layoffs and disband the blue-sky Uber AI Lab, the company sold most of its stake in its remaining self-driving arm (Uber ATG) while acquiring delivery firm Postmates. This indicates a shift from robo-taxis to last-mile delivery accelerated by the pandemic, and Uber is not alone. Self-driving startup Argo, backed by Ford, has signaled similar interest in delivery, testing the waters while partnering with a food-relief program to deliver contactless meals to students and their families.
The tech sector’s business model often aligns with the “move fast and break things” mentality, but they’ll have to be careful and courteous while integrating delivery robots into public pedestrian spaces and roadways. It certainly won’t hurt for them to be cute either.
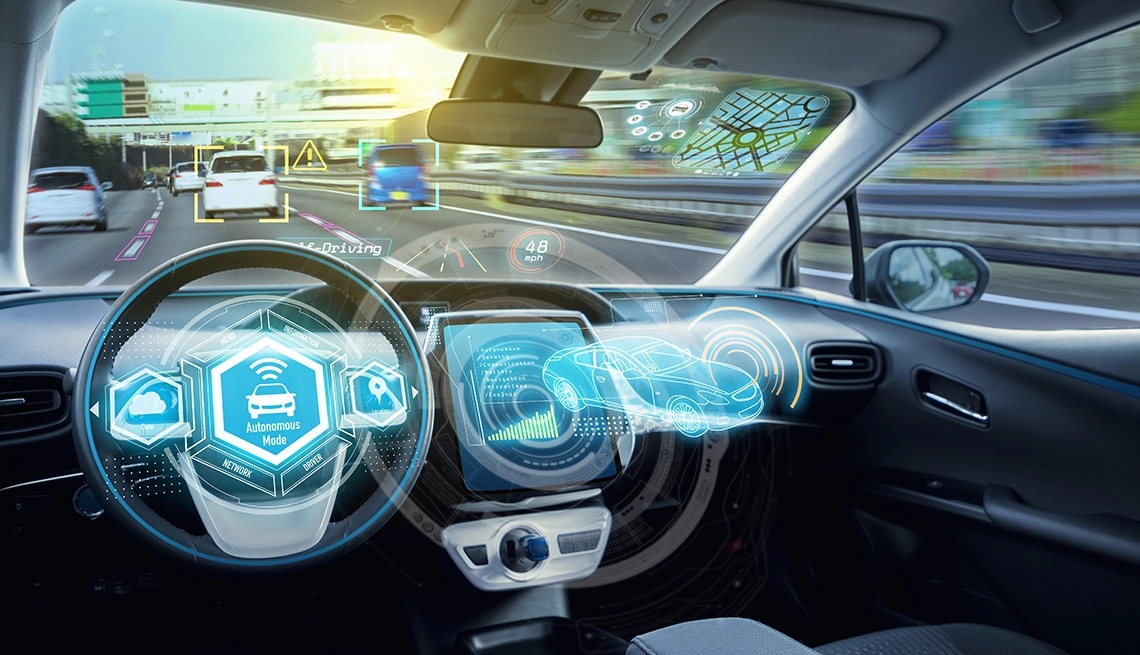
The Last Mile – How the Pandemic Revealed the New Applications of Autonomous Vehicles
The Acceleration of Autonomous Vehicle Applications due to COVID-19
Hint: It’s not focused on personal transportation
Autonomous vehicles have long been a mainstay of both outlandish fiction and legitimate research, in some interpretations predating the invention of the car itself. By some accounts, full self-driving capabilities would be a major boon in terms of safety and promises to narrow wealth-based gaps in good access to transportation. Perhaps the impact could be as significant as the adoption of safety bicycles, which expanded the distance one could travel by 3 to 4 times or more, without incurring the costs of owning a horse (or car) and the facilities to care for them.
A Common Perspective on Autonomous Driving
The most visible application of autonomous vehicles, and probably what most of us think of when we hear the term ‘autonomous vehicles,’ is the idea of self-driving cars, aka autonomous vehicles for personal transport. But personal transport may be the worst application for autonomous vehicles in terms of safety and attainability.
Self-driving cars may very well be remembered as the 2010’s jet pack: a much-hyped vaporware that’s never ready for widespread consumer use. At this point it’s essentially a tradition that every year (since 2016) Elon Musk predicts the imminent arrival of full self-driving capabilities in Tesla vehicles—any day now. As 2020 approaches its end, there’s no better time to reflect on missed expectations and disappointments, but let’s not forget to look for silver linings.
Jet pack comic cover in the public domain from Wikipedia
The Pandemic Accelerated Some Economic Trends
The COVID-19 pandemic has in many ways accelerated economic trends that have been predicted to occur anyway. Some of the accelerated shifts are troubling, like widening wealth gaps and a K-shaped recovery from recession. While other shifts, like increased support for remote working, are positive (though a widespread shift to work-from-home has also yielded nefarious downsides). This article posits that the arrival of full self-driving for private transportation is not one of those accelerated trends. In fact, several large players have taken 2020’s economic turmoil as an opportunity to divest from full self-driving research and development.
The stress that 2020 has placed on the logistics system and a boom in delivery services fueled by remote work and stay-at-home orders have revealed more promising areas for autonomous vehicles. There are a number of reasons why self-driving for personal transportation is not the best application to focus on for autonomous vehicle development, at least when considered in context next to the (relatively) low-hanging fruit of cargo transport and last-mile delivery. As we’ll argue below, the near-term future of autonomous vehicles will be more of an automated local courier service rather than a robo-taxi fleet.
Why You Shouldn’t Expect Your Personal FSD Vehicle Any Time Soon
#1 Driving is Dangerous
Driving is one of the most dangerous activities undertaken on a regular basis by large numbers of people. In the US, motor vehicle accidents are either the first or second leading cause of death by injury for all adult age groups according to the CDC, and injurious deaths are currently almost double the number of fatalities due to heart disease and cancer combined.
Estimates vary, but if you drive you probably have around a 1% chance of meeting your end due to a vehicle crash according to the National Safety Council. Minor accidents are even more common, and average drivers can expect to be involved in several over their lifetime.
Drivers can be dangerous to pedestrians and cyclists as well, and unfortunately that’s the sort of edge case that can confuse deep learning algorithms, if not trained for specifically. Even if a vision model was trained with a dataset with tens of thousands of examples of individuals riding upright safety bikes, a recumbent bike or adaptive trike may not be recognized as such, causing unpredictable problems.
Driving safety is a double-edge argument in justifying the development of self-driving cars. Self-driving could and should be safer than human drivers, and that is the number one reason why we might want widespread deployment of self-driving vehicles in the first place. Nonetheless, the intrinsic risks associated with big chunks of metal hurtling through space at speed couples high-stakes with a difficult problem domain.
As far as personal transportation is concerned, the first applications you should expect to see of self-driving “cars” will hardly fit our expectations. We see this already in dedicated vehicles like the miniature delivery van from Nuro.ai or the short-lived WePod trialed in the Netherlands. It just doesn’t make sense to retrofit a highway vehicle for slow, short, city transport on known routes, the most likely application area for self-driving to take hold.
A dedicated self-driving vehicle. Photo by Pixabay user falco (Pixabay license).
#2 Self-Driving is Hard
Go back a few years and there was nothing but optimism about the inevitable rise of self-driving vehicles. Driven in large part by early successes in deep learning for computer vision, it seemed like only a matter of time and training before driving yourself to work or to the shops would be a thing of the past. This wasn’t the only problem we expected deep learning to solve; throw a deep enough pile of artificial neurons at a problem, the thinking went, and your problem disappears (after a lucky hyperparameter search).
OpenAI even released their Universe project in 2016 with the promise of turning any computer task you can run in a Docker container into a reinforcement learning environment, the implication being that the sort of end-to-end intelligence necessary to solve a wide range of tasks with minimal experience was just around the corner.
Training is Important for Self-Driving Cars
Since those early days of optimism way back in the mid-2010s, we’ve learned a lot about the importance of good training data and the sheer difficulty of many problems for neural network models. Deep learning doesn’t do well with rare edge cases, is vulnerable to adversarial attacks and, in general, deep learning decisions remain inscrutable as they can’t explain themselves. Melanie Mitchell, a complexity researcher at the Santa Fe Institute and Portland State University and author of Artificial Intelligence: A Guide for Thinking Humans, posits that true full self-driving at a level comparable to humans essentially requires solving general intelligence. While automated transportation systems operating in controlled, known environments like largely static routes in urban/suburban areas are certainly possible with today’s technology, it’s unlikely that consumers will be able to buy a vehicle capable of a hands-free coast-to-coast road trip in unknown conditions anytime soon.
Deep Learning in Self-Driving Cars
Self-driving cars are notoriously bad at recognizing situations they haven’t seen before, and the deep learning models at the heart of their computer vision systems can fail in surprising ways. One of the first fatalities directly attributable to self-driving technology came from Tesla’s Autopilot in 2016. In the accident, Autopilot mistook the bright side of a semi-trailer as sky, colliding with the underside of the trailer at a speed sufficient to kill the driver.
Interested in a deep learning solution?
Learn more about Exxact AI workstations starting at $3,700
The fatal 2016 Autopilot failure and others like it emphasize a particular risk of self-driving and advanced driver assistance systems when drivers become complacent, but they’re not the most notorious examples of self-driving crashes. The 2018 fatality of Elaine Herzberg at the wheels of Uber’s self-driving Volvo XC90 was arguably due to a lax safety culture, as emergency braking was disabled and the supervising driver was allegedly watching videos on their mobile phone. These failures are not comprehensive, but they are noteworthy as stemming from situations that were created by the semi/autonomous driving features themselves.
Adversarial Attacks in Vision Models
Another avenue for failure is adversarial attacks. In 2017 researchers demonstrated that they could cause a computer vision model to mistake stop signs for speed limit signs with only a few pieces of tape. These attacks may be rare in the wild and quickly patched (and likely prosecuted) when found, but they certainly point to the brittle fragility of deep learning models. That’s not a great mix with the high stakes of automobile travel. One can bet that if self-driving vehicles become commonplace, human drivers will quickly discover behavioral adversarial attacks against self-driving systems.
Ultimately, where a few years ago several self-driving startups had high hopes for end-to-end learning, i.e. formulating driving as a reinforcement learning problem and training driving agents like you might teach a dog new tricks. While companies like Wayve and Comma are ostensibly still following this strategy, most larger players like Tesla, GM, Waymo, and others are focusing on a hybrid approach.
Are We Witnessing a Shift in Self-Driving Cars?
Thanks to the vulnerability of conventional deep learning models to uncertainty and risk (to say nothing of deliberate adversarial perturbations), production self-driving systems are more likely to weave together deep learning for computer vision and sensor representation fusion with more conventional hand-coded automation. In other words, self-driving is starting to look a lot more like driver assistance functionality, which raises another challenge any self-driving developer, investor, or enthusiast would be remiss to ignore.
#3 The Bar for Self-Driving Cars is a Moving Target
Driving is one of the most dangerous activities that many people partake in on a regular basis. This offers both opportunities and challenges for self-driving vehicles. On the one hand, it means there’s room to do better with autonomous vehicles: software does not get tired, bored, or distracted like humans do. On the other hand, it’s indicative of a genuinely difficult problem domain.
What’s more is that any improvement in safety developed for self-driving is almost universally accompanied by associated improvement in driver assistance capabilities. It’s hard to beat the combination of the robotic reflexes and unwavering attention of software with the flexible problem solving and general adaptability of humans.
It’s unlikely that self-driving vehicles will be approved for widespread use if they don’t significantly (statistically and in terms of effect size) improve on the safety of human drivers. However, despite the high yearly cost of driving in terms of fatalities and injuries, individual human drivers are already pretty safe drivers considering the distances travelled, and driver assistance functionality does seem to be decreasing insurance claims when in use despite some criticisms of drivers being overly reliant on features like emergency braking, automatic blind spot monitoring, and lane keeping assistance. If human drivers keep getting safer, albeit with some automated safety assistance, self-driving cars will have a harder time overcoming the threshold.
Companies Involved in Self-Driving Cars
Company | Status | Sensors |
Waymo | Consistently considered a leader in the AV space. | “Laser Bear Honeycomb” lidar, cameras, radar |
Tesla | Efforts led by Andrej Karpathy focusing on computer vision | Radar, cameras, ultrasonic sensors. Notably lacking lidar |
Uber ATG | Sold to Aurora | “FirstLight” lidar, cameras, radar |
Wayve | Actively pursuing end-to-end autonomous driving | Cameras |
Argo | Backed by Ford | Lidar, cameras, radar |
Comma | Comma Two hooks into onboard driver assistance features. | Cameras (including driver monitoring), onboard sensors. Runs on hardware essentially equivalent to a mobile phone. |
Selected players in the self-driving field. In addition to the sensors listed, most self-driving systems use GPS and some use pre-defined high-definition maps.
Why Last Mile Delivery?
Here we’ll focus on the tricky last mile of transport and delivery. This domain includes the type of journey more suited to a bicycle courier than to a truck driver. Some estimates predict that last-mile deliveries will be performed by robots 85% of the time by 2025.
#1 ‘Sidewalk’ Delivery is an Intrinsically Safer Domain
A two-ton automobile traveling at 75 mph has a kinetic energy of a little more than 1 MegaJoule, or about as much energy as half a pound of TNT. A 100 pound robot traveling at a moderate cycling speed of 12 mph has about 678 Joules of kinetic energy, and an injury potential similar to that of a slow cyclist. On sidewalks, where speeds would normally be limited to a brisk walking pace, the potential for injury is even less. It’s also not particularly dangerous (though it will be annoying) for a small delivery robot that gets confused to just stop or pull to the side of the sidewalk to await instructions.
The passengers are certainly lower-stakes as well. If an autonomous delivery robot drives your burrito off a cliff, no big deal, the order can easily be replaced and your life goes on, albeit with dinner a bit delayed. The same can’t be said for a self-driving car suffering catastrophic failure while taking you to the drive-thru.
#2 Delivery Can Be an Easier Problem
The stakes are lower for last-mile delivery relative to full self-driving, but the problem may also be somewhat easier to solve. The speeds are slower (making reaction times less important), and routes are likely to be well-known. You could even imagine a delivery vehicle designed for deriving maximum empathy from bystanders that could issue a pitiable cry for help to solicit a rescue if it got stuck in a pothole.
Those factors and others should make it easier to develop a system that works “well enough.” When things go wrong, and they inevitably will, it’s easier to rescue a lost robot because when making local deliveries they are never out of range of remote control help. The most effective way is probably going to be to have a team of teleoperation supervisors ready to take control at a moment’s notice, making for a hybrid semi-autonomous last-mile delivery system.
#3 Last-Mile Delivery is Well-Suited to Human-in-the-Loop Deployment
Given the complexity and unpredictability of the real world, it’s inevitable that surprises will occur. When they do, a broken robot or lost meal is a lot more manageable downside than death and dismemberment. That being said, last-mile delivery is also more amenable than full self-driving cars human-in-the-loop interventions when things go wrong.
As mentioned in the section on self-driving cars, a human driver with supervisory duties over an autonomous automobile tends to become bored, complacent, and over-reliant on semi-autonomous vehicles. A single human teleoperator would have plenty to keep them busy, and can look after a fleet of numerous delivery robots. That’s probably why Postmates contracted teleoperation specialists Phantom Auto for their expertise, and DoorDash acquired remote operation startup Scotty Labs.
A Hybrid Approach is More Than Likely The Future
A hybrid approach to autonomous vehicles may feel like it falls short of the myriad examples we’ve come to expect from science fiction and comic books, but it’s a pragmatic solution to a practical problem. Thanks to boredom and complacency, self-driving cars that only occasionally require human intervention are probably more dangerous than both fully autonomous (level five) or semi-autonomous vehicles that require correction more often. Delivery robots that require fewer interventions just means that remote operators can handle supervision of more vehicles.
Companies Involved in Autonomous Delivery
Company | Status | The Robot | Sensors |
Starship | In operation in several US and UK locations. | A good example of a six-wheeled robot in the cooler-sized class | Cameras, unspecified edge sensors (perhaps ultrasonic) |
Postmates | Acquired by Uber | “Serve,” about the size of a drinks cooler on wheels” can carry ~50 pounds, with human-in-the-loop teleoperation. | Lidar, sonar, cameras |
Amazon | Acquired Dispatch in 2019 | “Scout” is a small six-wheeled robot sporting the Amazon Prime smile logo. | Cameras |
Nuro | Recently completed $500 million funding round, running trials in Houston, Texas and Scottsdale, Arizona. | “R2,” a small autonomous van with capacity for an entire week’s groceries for several recipients at once. | Cameras, radar, lidar |
Academy of Robotics | Trialling services in London and Surrey | The “Kar-Go” delivery vehicle, smaller than an ATV, is probably the coolest-looking robot on the list. | Uses Bayesian Simultaneous Localization and Mapping (SLAM) algorithms to fuse vision, lidar, and inertial sensors. |
UDI | Chinese delivery startup that stepped into the limelight during spring lockdowns. | Boxy, autonomous van similar to Nuro’s R2. | Cameras, lidar |
Noteworthy players in the autonomous last-mile delivery space.
Challenges to Robotic Delivery
As we outlined above, robotic delivery is in many ways an easier problem with lower stakes than self-driving cars for personal transportation. That doesn’t mean the task comes without its own challenges, the first of which will fit nicely into the dystopian worldview you’ve come to know and love.
#1 Human Delivery Workers are Cheap
How do gig economy services employing (excuse me, contracting) a ragtag collection of semi-professional drivers and couriers compete with established players employing professionals? In some cases this may be a matter of servicing the long tail of under-served niches, but the main advantage seems to be cheapening the cost of labor. Rideshare and delivery providers like Uber and Lyft benefit from offloading many of the costs of doing business (vehicle maintenance, payroll taxes, health insurance etc.) to their workers. They’re willing to lobby heavily to protect the status quo, recently spending a combined $205 million on California’s Prop 22 to do just that.
Depending on who produces the estimates, Uber and Lyft drivers make somewhere between $5.64 and $27.58 an hour, with the study producing the latter number funded by Lyft. Estimates for gig work delivery couriers tend to fall on the lower end of that spectrum as well.
No matter which way you slice it, cheap human labor is powering the gig economy, but that could change if regulations, collective action, or a tightening labor market push wages higher. If and when that happens the opportunity for friendly robotic replacements will grow, so long as they are accepted by the humans they rub elbows with.
#2 Critics Decry the Takeover of Public Spaces
hitchBOT was a stationary robot that interacted with humans with basic conversational skills and blinking lights, ultimately using its charm to hitchhike through Canada and Europe. Then hitchBOT tried its luck on a route from San Francisco to Boston, crossing all the way to Philadelphia before being destroyed. Pennsylvania may have legally classified delivery robots as pedestrians, but that doesn’t mean the robots themselves aren’t vulnerable.
Photo of hitchBOT and friends CC BY flickr user archieflickers.
The expected proliferation of delivery robots using sidewalks is expected by some to be a major source of conflict going forward, stressing public infrastructure that is already given second-class status in US cities. Robotic delivery services with slightly larger vehicles like China’s UDI, Nuro in the United States, or that of Academy of Robotics in England, may fare better integrating into motorway traffic.
Given the public response to undocked scooter and bike share vehicles invading public sidewalks, providers of delivery robot services will have to be careful. Robots sharing human spaces need to elicit empathy from those around them and act respectfully in crowded areas.
The Pandemic Has Affected the Self-Driving Industry in Many Ways
With overall consumer excitement for self-driving cars in general seriously waning by 2020, a pandemic-related recession further dampened enthusiasm in the industry, already at risk due to wavering consumer sentiment and a recognition that solving the problem is harder than anticipated. But the explosion of demand for contactless delivery services has had the opposite effect on the appetite for autonomous local and last-mile delivery startups, which is readily apparent in the recent $500 million dollar investment round completed by robotic delivery startup Nuro.
Photo by Bill Nino on Unsplash (Unsplash license)
There may be no better example of where the industry is headed than the example of Uber. In addition to taking advantage of the recession to issue thousands of layoffs and disband the blue-sky Uber AI Lab, the company sold most of its stake in its remaining self-driving arm (Uber ATG) while acquiring delivery firm Postmates. This indicates a shift from robo-taxis to last-mile delivery accelerated by the pandemic, and Uber is not alone. Self-driving startup Argo, backed by Ford, has signaled similar interest in delivery, testing the waters while partnering with a food-relief program to deliver contactless meals to students and their families.
The tech sector’s business model often aligns with the “move fast and break things” mentality, but they’ll have to be careful and courteous while integrating delivery robots into public pedestrian spaces and roadways. It certainly won’t hurt for them to be cute either.