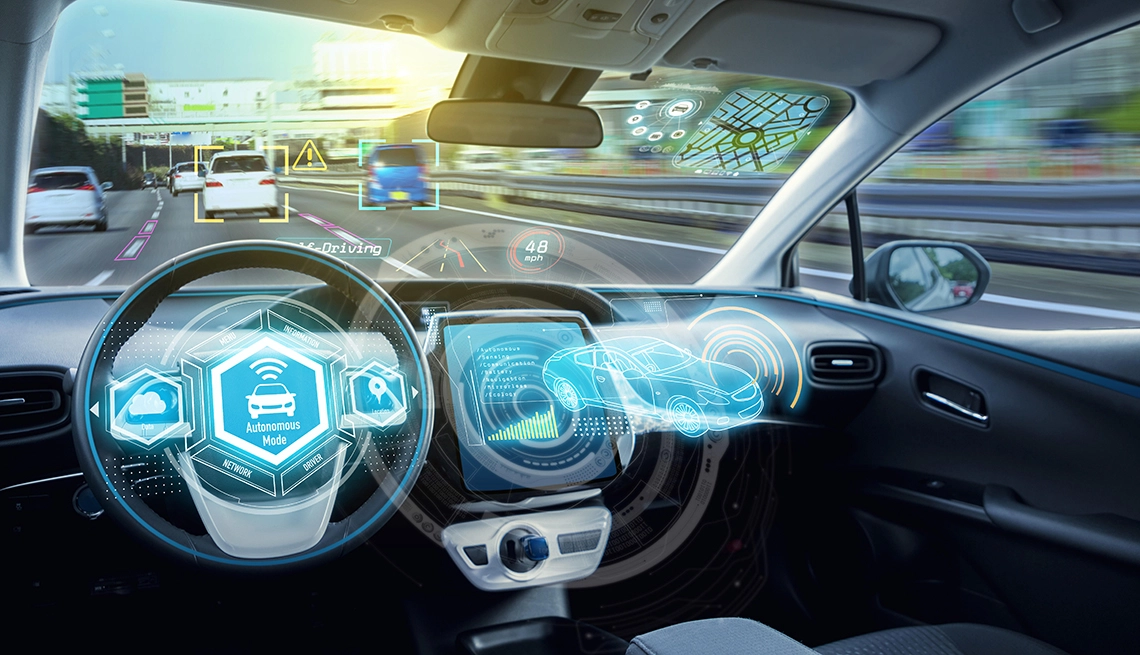
Deep Learning Problems in Robotics
When data scientists talk about Deep Learning, they’re usually speaking about image generation, detection, classification and regression tasks. Still, the thing that deep learning and artificial intelligence are getting vastly used for is in the field of robotics, and solving some its most significant challenges. It is deep learning for computer vision that is powering the pursuit of self driving autonomous cars. Reinforcement learning is also powering some of the initiatives like AlphaGo, where the agent tries to act in the world to maximize its rewards.
The advancements in deep learning have been many, but still, we want to reach the ultimate goal at some point in time — Artificial General Intelligence.
What is AGI and Can a Robot Perform a Task?
Artificial General Intelligence (AGI) is the hypothetical intelligence of a machine that can understand or learn any intellectual task that a human being can.
One of the tests to confirm human-level AGI is suggested by Steve Wozniak in which “A machine/robot is required to enter any average American home and figure out how to make coffee” — That is, the robot has to find the coffee machine and coffee, add water, find a mug, and brew the coffee.
Can a Robot Make coffee?
This task might look too easy for humans. Still, for a robot, it essentially means interacting with the environment in the right ways using various computer vision techniques to recognize and use the objects, and then taking all the right actions based on its understanding of the task.
Yet another test to confirm AGI is the Turing test itself, in which a human talks to a machine/human and the first human has to guess if he/she is talking to the machine/human. If the machine fools the human a significant number of times, the machine will pass the test.
This test involves a deep understanding of human language and its structure, a sense of how humans use language in continuity rather than single instances.
How Can AI help?
A lot of public figures, chief among them Elon Musk, Bill Gates, and the late Stephen Hawking, endorse that AI might pose an existential risk (think Terminator’s Skynet); but we are nowhere near the point where we really must be afraid of it.
While advancements like AlphaGo beating a Go world champion do make us ask if the computers have really surpassed humans, the point we have to understand is that the machine is essentially trying to mimic logic based on the millions of games it has seen. These games all have a lot of training data present, and the degree of freedom when it comes to the actions taken by the AI is vastly limited compared to the real world. Another thing is that these AIs we have are not multi-functional. This means that while they could be great at doing a single thing, they don’t do anything else. Making something as powerful as a machine that could think on its own and have real-world consequences is virtually a thing of the future. In fact, it might be better to think along the lines of Andrew Ng, who states that AI existential risk is “like worrying about overpopulation on Mars when we have not even set foot on the planet yet.”
We are pretty far from a dystopian future.
That being said, we are making some progress towards realizing it. And, figuring out how to put filters or restrictions in place now to make sure an AI doesn’t get carried away and wipe out humanity should absolutely be considered. We are already struggling with AI bias based on how they are trained and by whom, so there are still some barriers in place before we realize a fully functioning, autonomous AI of the future.
Researchers are already working on deep learning for computer vision (CV) which can help a robot and robotics applications understand their environments using various sensors, and help them navigate through obstacles in their path. This is called the mapping challenge, and machine learning and CV technologies can really help with this. This is going to be an essential part of autonomous, self-driven cars which do have to learn how to see in new conditions even when no training data is present.
Interested in a deep learning solution?
Learn more about Exxact AI workstations starting at $3,700
A lot of research has also been happening around speech recognition which can be used to convert spoken language into machine language, and Natural Language Processing (NLP) which can provide a machine with the ability to understand and talk to a human. A very inferior version of such technologies is already utilized by some of us through our Alexa, Portal and Google home devices. In fact, GPT-3, a new language-generation model that has been in the news for creating interesting write-ups, is again powered by using deep learning in natural language processing.
Some Parting Thoughts About Deep Learning in Robotics
The state of AI these days tends to solve problems in a very disconnected way. The vision problem is solved separately from the language/speech problem, for example.
The next thing we need to do is integrate all this into a working robot which can make its own decisions. The agent needs to be able to experience the real world first hand to create its own view of the world and acquire additional data from its environment that it could use to train itself.
While it is easier said than done, tiny step advancements are required to reach our destination, but we continue to move in the right direction. It’s only a matter of time until robots attain AGI and open a whole new world of possibilities.
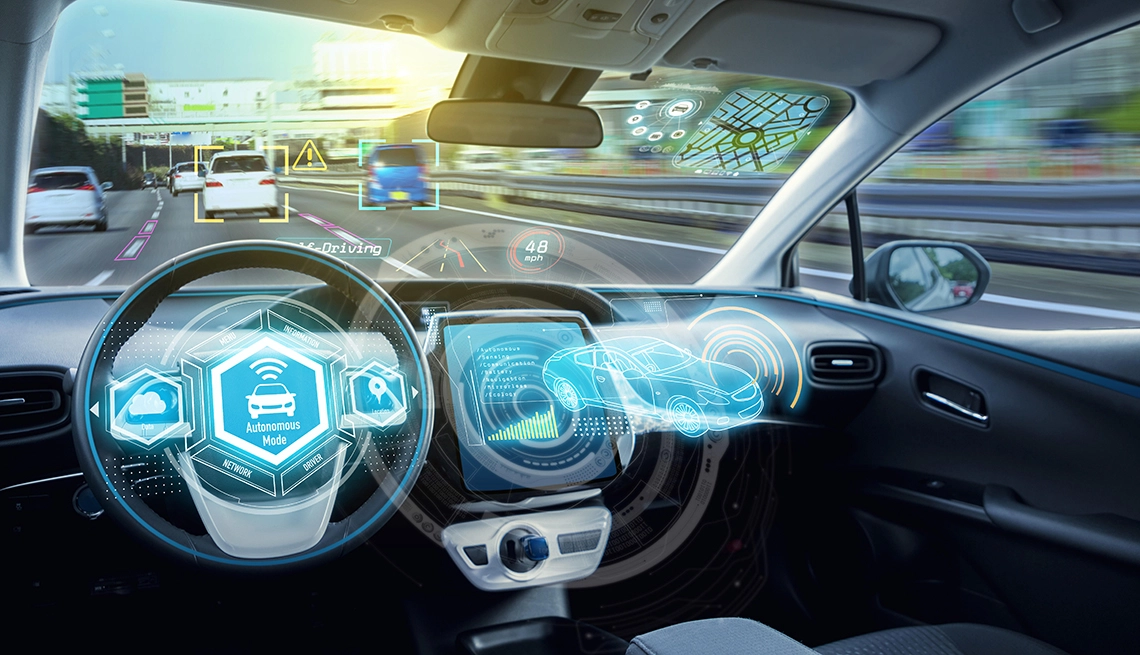
How Can New Deep Learning Initiatives Overcome Challenges in Robotics?
Deep Learning Problems in Robotics
When data scientists talk about Deep Learning, they’re usually speaking about image generation, detection, classification and regression tasks. Still, the thing that deep learning and artificial intelligence are getting vastly used for is in the field of robotics, and solving some its most significant challenges. It is deep learning for computer vision that is powering the pursuit of self driving autonomous cars. Reinforcement learning is also powering some of the initiatives like AlphaGo, where the agent tries to act in the world to maximize its rewards.
The advancements in deep learning have been many, but still, we want to reach the ultimate goal at some point in time — Artificial General Intelligence.
What is AGI and Can a Robot Perform a Task?
Artificial General Intelligence (AGI) is the hypothetical intelligence of a machine that can understand or learn any intellectual task that a human being can.
One of the tests to confirm human-level AGI is suggested by Steve Wozniak in which “A machine/robot is required to enter any average American home and figure out how to make coffee” — That is, the robot has to find the coffee machine and coffee, add water, find a mug, and brew the coffee.
Can a Robot Make coffee?
This task might look too easy for humans. Still, for a robot, it essentially means interacting with the environment in the right ways using various computer vision techniques to recognize and use the objects, and then taking all the right actions based on its understanding of the task.
Yet another test to confirm AGI is the Turing test itself, in which a human talks to a machine/human and the first human has to guess if he/she is talking to the machine/human. If the machine fools the human a significant number of times, the machine will pass the test.
This test involves a deep understanding of human language and its structure, a sense of how humans use language in continuity rather than single instances.
How Can AI help?
A lot of public figures, chief among them Elon Musk, Bill Gates, and the late Stephen Hawking, endorse that AI might pose an existential risk (think Terminator’s Skynet); but we are nowhere near the point where we really must be afraid of it.
While advancements like AlphaGo beating a Go world champion do make us ask if the computers have really surpassed humans, the point we have to understand is that the machine is essentially trying to mimic logic based on the millions of games it has seen. These games all have a lot of training data present, and the degree of freedom when it comes to the actions taken by the AI is vastly limited compared to the real world. Another thing is that these AIs we have are not multi-functional. This means that while they could be great at doing a single thing, they don’t do anything else. Making something as powerful as a machine that could think on its own and have real-world consequences is virtually a thing of the future. In fact, it might be better to think along the lines of Andrew Ng, who states that AI existential risk is “like worrying about overpopulation on Mars when we have not even set foot on the planet yet.”
We are pretty far from a dystopian future.
That being said, we are making some progress towards realizing it. And, figuring out how to put filters or restrictions in place now to make sure an AI doesn’t get carried away and wipe out humanity should absolutely be considered. We are already struggling with AI bias based on how they are trained and by whom, so there are still some barriers in place before we realize a fully functioning, autonomous AI of the future.
Researchers are already working on deep learning for computer vision (CV) which can help a robot and robotics applications understand their environments using various sensors, and help them navigate through obstacles in their path. This is called the mapping challenge, and machine learning and CV technologies can really help with this. This is going to be an essential part of autonomous, self-driven cars which do have to learn how to see in new conditions even when no training data is present.
Interested in a deep learning solution?
Learn more about Exxact AI workstations starting at $3,700
A lot of research has also been happening around speech recognition which can be used to convert spoken language into machine language, and Natural Language Processing (NLP) which can provide a machine with the ability to understand and talk to a human. A very inferior version of such technologies is already utilized by some of us through our Alexa, Portal and Google home devices. In fact, GPT-3, a new language-generation model that has been in the news for creating interesting write-ups, is again powered by using deep learning in natural language processing.
Some Parting Thoughts About Deep Learning in Robotics
The state of AI these days tends to solve problems in a very disconnected way. The vision problem is solved separately from the language/speech problem, for example.
The next thing we need to do is integrate all this into a working robot which can make its own decisions. The agent needs to be able to experience the real world first hand to create its own view of the world and acquire additional data from its environment that it could use to train itself.
While it is easier said than done, tiny step advancements are required to reach our destination, but we continue to move in the right direction. It’s only a matter of time until robots attain AGI and open a whole new world of possibilities.