Supported Software


Deep Learning
TensorFlow
TensorFlow is an open-source software library for numerical computation using data flow graphs. Conduct machine learning and deep neural networks research. The flexible architecture allows you to deploy computation with a single API.
Capabilities & Features
Easy Model Building
Build and train ML models easily using intuitive high-level APIs like Keras with eager execution, which makes for immediate model iteration and easy debugging.
Robust ML Production
Easily train and deploy models in the cloud, on-prem, in the browser, or on-device no matter what language you use.
Powerful AI Experimentation
A simple and flexible architecture to take new ideas from concept to code, to state-of-the-art models, and to publication faster.
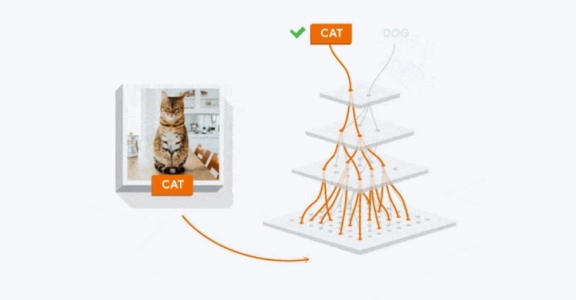
Why Develop with TensorFlow?
TensorFlow is an end-to-end open source platform for machine learning. It has a comprehensive, flexible ecosystem of tools, libraries and community resources that lets researchers push the state-of-the-art in ML and developers easily build and deploy ML powered applications.
The TensorFlow Ecosystem
The TensorFlow community is an active group of developers, researchers, visionaries, tinkerers and problem solvers. The door is always open to contribute, collaborate and share your ideas.
TensorFlow Core
Build and train ML models easily using intuitive high-level APIs like Keras with eager execution, which makes for immediate model iteration and easy debugging.
TensorFlow.js
TensorFlow.js is a JavaScript library for training and deploying models in the browser and on Node.js.
TensorFlow Lite
TensorFlow Lite is a lightweight library for deploying models on mobile and embedded devices.
TensorFlow Extended
TensorFlow Extended is an end-to-end platform for preparing data, training, validating, and deploying models in large production environments.